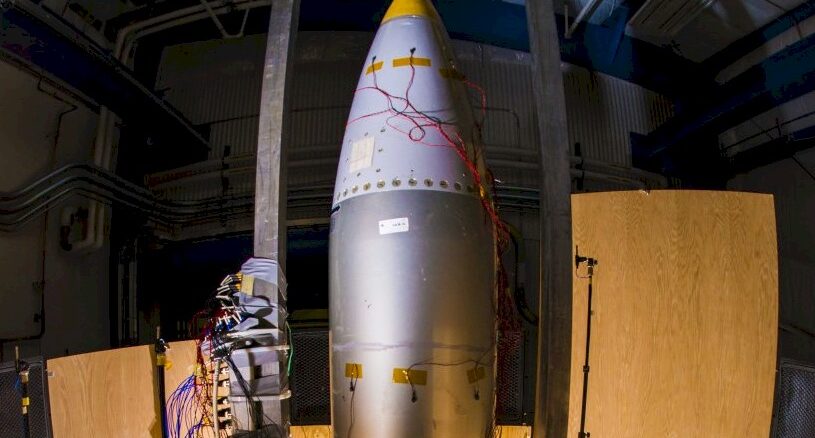
If the HPC community didn’t write the Comprehensive Nuclear Test Ban Treaty of 1996, it would have been necessary to invent it.
More than any of the many factors that drive the development of capability-class supercomputers, including the desire to do great science to change the world for the better and to save lives, the need to design, re-design, and maintain the nuclear weapons arsenals of the world has pushed the performance envelope of supercomputers.
So far, nuclear weapons have only been used twice to end World War II, and the stockpiles of 12,705 nuclear warheads – with 3,732 deployed in bombs and missiles, mostly in the United States and Russia, with the remaining 5,708 in “a state of high operational alert,” again in the US and Russia – and have acted as a deterrent to the start of World War III. The stakes have been too high to use such weapons, and thankfully we have not.
This is an amazing fact, and it warrants a thoughtful pause. So does the fact that we are able to have created such complex, powerful, and monstrous machines in the first place. The world might be safer without them, but maybe not. It is hard to say for sure.
The fact is, nuclear weapons exist, we have not had World War III, and nuclear weapons age. And in order to continue to be a deterrent, the threat of use for nuclear weapons has to be legit and unquestionable. That is what maintaining the nuclear arsenal really means. This is not just vacuuming the dust out of missile siloes and making sure batteries are charged and engines are fueled.
And so the major nuclear powers – the United States, Russia, France, and the United Kingdom, and China and to a lesser extent India, Pakistan, Israel, and North Korea – have spent untold fortunes over the decades not only to build their nuclear warheads and their delivery mechanisms, but to build the most advanced systems in the world to predict the behavior of these weapons, many of which are several decades old and to try to make modifications to them without actually testing those design changes by blowing one up.
It has been a long time since the US Department of Energy set up the National Nuclear Security Administration in 1995, and with it the Advanced Simulation and Computing program that funded, with a 15-year, $67 billion Congressional appropriation initially, the supercomputers that were created by IBM, Silicon Graphics, Intel, Cray, Thinking Machines, and others to be installed at the so-called Tri-Labs that take care of the nuclear arsenal. That’s Lawrence Livermore National Laboratory, Los Alamos National Laboratory, and Sandia National Laboratories, which because of this NNSA mission, still dominate supercomputing in the United States. Technically speaking, Oak Ridge National Laboratory does not do nuclear weapons work, but it certainly does a lot of science (some of which might be tangentially useful to the NNSA mission) and in recent years at least has been the home of the flagship supercomputer in America. And of course, for many decades, the US Defense Advanced Research Projects Agency was tag teaming with the DOE to drive supercomputing designs that benefitted the NNSA mission, and that stopped promptly after the “Cascade” line of XC supercomputers from Cray, with the “Aries” interconnect, once the Department of Defense realized it did not need a petaflops or an exaflops supercomputer, but a teraflops supercomputer that fit in a backpack, as Robert Colwell, director of the microsystems technology office at DARPA and a former Intel exec, explained at Hot Chips 25 a decade ago.
Since that time, the drive to exascale effort in the US has been shouldered by the DOE, and in particular the Tri-Labs facilities in conjunction with Argonne National Laboratory, Lawrence Berkeley National Laboratory, and Oak Ridge. And as far as we can tell, based on a reading of a paper put out by many luminaries in the HPC community pondering the future of computing at the NNSA under the auspices of the National Academy of Sciences, the simulation task is a lot harder than we ever imagined and certainly more complex than we ever remember.
That paper, Charting a Path in a Shifting Technical And Geopolitical Landscape: Post-Exascale Computing for the National Nuclear Security Administration, is a whopper. We read front to back and have been chewing on it for six days, mainly because it really does explain, and certainly not in layman’s terms, how hard it is to combine the data from 1,045 actual nuclear warhead explosions, countless physical experiments at the Nevada National Security Site, with heaven only knows how many simulations that have been run over the decades to ensure that the weapons work and that modifications made to them do not break them.
The major nuclear weapons labs were the sites of the largest concentrations of computing power on Earth until the rise of the hyperscalers, and perhaps back in 1995 when the NNSA was formed, the Defense Department and the Department of Energy were overly optimistic about how we would someday be able to simulate a full nuclear weapon and its explosion. We remember that transition from actual nuke testing to a combination of simulation and experiment happening in the mid-1990s, which is also when supercomputing itself was transitioning from vector machines to massively parallel RISC systems that offered an order of magnitude more performance and much better bang for the buck. (So to speak.)
We just never appreciated how hard the task of maintaining the nuclear stockpile and modifying weapons and knowing they will work, with a very low uncertainty qualification that is central to the NNSA mission, was. The fact that this was hard, and involved computers and simulation, saved the art of weapons design and the budgets for it, according to the Scientific American story cited above. But simulating nuclear processes is apparently harder than making a bomb. Witness the speed at which India, Pakistan, and North Korea got the bomb but are not supercomputing powerhouses. China is set to more than double its nuclear arsenal, and has become a supercomputing powerhouse.
Virtual Bang For The Real Buck
Computing and nukes have been hand-in-hand partners from the beginning.
The Manhattan Project had large teams of human calculators doing math at insurance companies – a kind of massively parallel processing – we know someone whose father worked at an insurance company in New York who did this tabulating work in a secure room for many months and never knew what it was all about until after the fact.
The vacuum tube-powered ENIAC computer, which made Sperry Rand a powerhouse in early computing, was used to do calculations as part of the Manhattan Project by none other than John von Neumann, who was a mathematician at Los Alamos working on the bomb. Los Alamos installed a custom variant of ENIAC in 1952, called the Mathematical Analyzer, Numerical Integrator, And Computer or MANIAC for short. (Of course.) The long line of supercomputers installed by Los Alamos, Sandia, and Lawrence Livermore will culminate this year with the installation of the capability-class, more than 2 exaflops “El Capitan” supercomputer at Lawrence Livermore, which will be based on the AMD Instinct MI300A hybrid CPU-GPU compute engines and which will cost $600 million to get the hardware and systems software into the field.
If the end of Moore’s Law and the need for computing power well in excess of 1 exaflops – the report is careful to never specify how much – all within a reasonable power budget were not bad enough, the report outlines the big change that we have been talking about for years: The economic model for capability-class supercomputing no longer works, and there is a non-zero chance that HPC will be eaten by the hyperscalers and clouds.
“The NNSA procurement model must change,” the National Academy of Sciences report states, and we would say that this also holds for non-NNSA supercomputing centers funded through the Department of Energy or the National Science Foundation and their analogs around the world. “Fixed price contracts for future, not yet developed products have created undue technical and financial risks for NNSA and vendors alike, as the technical and delivery challenges surround early exascale systems illustrate. A better model embraces current market and technical realities, building on true, collaborative co-design involving algorithms, software, and architecture experts that harvests products and systems when the results warrant. However, the committee emphasizes that these vendor partnerships must be much deeper, more collaborative, more flexible, and more financially rewarding for vendors than past vendor R&D programs. This will require a cultural shift in DOE’s business and procurement models.”
We will speak more bluntly than this. The HPC centers in the United States have had killer deals on HPC systems, probably paying half of list price or less, for decades. Vendors stepped up to the plate because they believed in the mission that deterrence prevented World War III and they could look at their losses (or lack of profits) as a means to develop future HPC systems that could be democratized to the world. While this has happened to some degree, we would observe that many institutions that should be using simulation and modeling do not, and now they have all caught the ChatGPT virus.
We concur that the days of fixed cost contracts are not just probably over, they are over. The only thing that is fair, given the wide variety of uncertainties, is a cost-plus contract. This is, by the way, the pricing that we think the major clouds will require since they are in business to make money. As were supercomputer vendors all these years. We definitely go so far as to say that the behavior of the US centers in demanding fixed cost contracts has driven consolidation in the supercomputing industry – it literally drove SGI and Cray into the arms of HPE – and even then HPE is not making any significant money (meaning profits) in HPC after shipping the world’s first official exascale supercomputer. IBM, in disgust, left the field because it cannot afford to lose money on HPC. Sun Microsystems, which could have been an HPC contender, is dead.
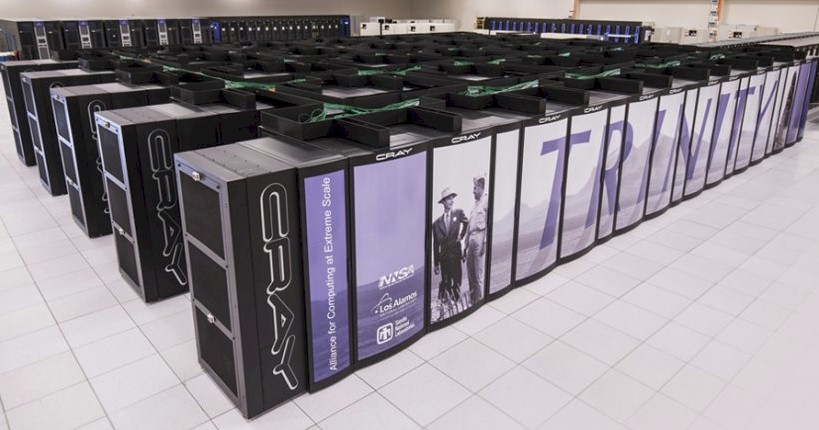
There was much talk about the clouds in the National Academy of Sciences report, which is natural enough. But this paragraph laid the issue facing the NNSA out plainly:
“Simply put, the scale, scope, and rapidity of these cloud and AI investments now dwarf that of the DOE ASCR and ASC programs, including ECP. Tellingly, the market capitalization of a leading cloud services company now exceeds the sum of market capitalizations for traditional computing vendors. Collectively, this combination of ecosystem shifts increasingly suggests that NNSA’s historical approach to system procurement and deployment – specifying and purchasing a leading-edge system roughly every 5–6 years –is unlikely to be successful in the future.”
We would argue that the pace of investment for both NNSA and for weather and climate modeling and for other concrete use cases in the simulation of systems that directly benefit the economy needs to be accelerated. We have been too conservative and have not built machines fast enough. For instance, we need a pair of 10 exaflops machines at the National Oceanographic and Atmospheric Administration to get global weather modeling down below the 1 kilometer grid resolution that will make weather forecasts a step function more accurate. Whether or not this is on a cloud remains to be seen. That is a deployment model, not a strategy. We don’t need to worry about whether this is a capital budget or an operational budget so much as that the United States, as a country, is woefully underinvesting in HPC.
Despite all of the HPC work that Microsoft Azure and Amazon Web Services are doing to expand into the HPC sector, we do not think the clouds are going to be able to support NNSA workloads without a change in pricing. A case could be made to use very expandable cloud hardware plus a homegrown HPC software stack, and we even suggested this week in looking at the future NERSC-10 system going into Lawrence Berkeley National Laboratory that the clouds might be the only possible alternative bid to HPE for the machine.
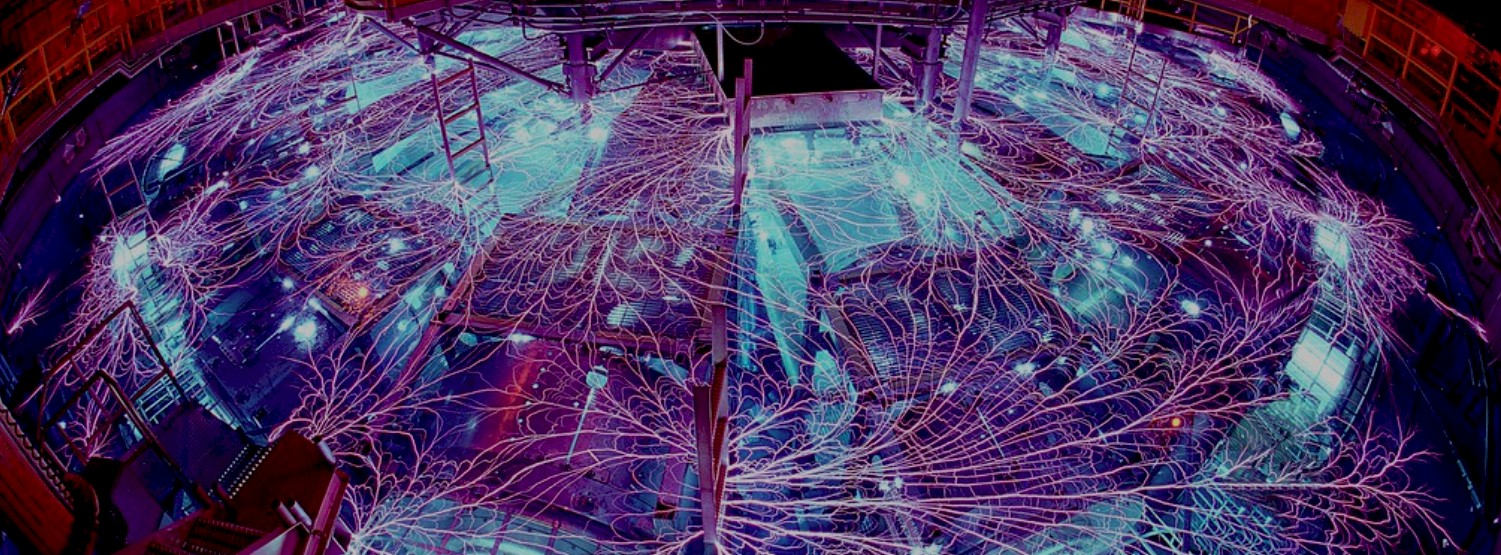
“Looking forward,” says the NNSA report, “it is possible that no vendor will be willing to assume the financial risk, relative to other market opportunities, needed to develop and deploy a future system suitable for NNSA needs. Second, the hardware ecosystem evolution is accelerating, with billions of dollars being spent on cloud and AI hardware each year. NNSA’s influence is limited by its relatively slow procurement cycles and, compared to the financial scale of other computing opportunities, is also limited in its financial leverage. Third, and even more importantly, the hardware ecosystem is increasingly focused on cloud services and machine learning, which overlap only in part with NNSA computing application requirements.”
That’s pretty sobering, isn’t it?
Running the possible scenarios in our head, we made this crazy suggestion in our NERSC-10 story, which bears repeating in the context of this NNSA report:
“Perhaps the Department of Energy and the Department of Defense will have to start their own supercomputer maker, behaving themselves like a hyperscaler and getting a US-based original design manufacturer (ODM) to build it. Why not? We need a big US-based ODM anyway, right? (Supermicro sort of counts as the biggest one located in the United States, but there are others.) And NERSC-10 is not being installed until 2026, so there is time to do something different. But if that happens, competitive bidding goes out the window because once it is set up, Uncle Sam Systems, as we might call it, will win all of the deals. It will have to in order to justify its existence.”
Way at the back-end of the report from the National Academy of Sciences on the future path of system procurement for the NNSA, this statement just stopped us dead, which we will quote in full:
“A fundamental concern in many areas such as the examples mentioned above is that relying on a weak-scaling approach based on larger versions of architectures currently being deployed at the exascale is no longer a viable strategy. Increasing fidelity by increasing spatial resolution necessitates an increase in temporal resolution. Consequently, even if one assumes ideal scaling, this weak-scaling paradigm results in increasing time to solution. For example, increasing the resolution of a three-dimensional high-explosive detonation simulation by an order of magnitude will increase resources needed to store the solution by three orders of magnitude and increase the time to solution by an order of magnitude (or more if any aspect of the simulation fails to scale ideally). For simulations that require several weeks or more to complete now, the time-to-solution for a higher-resolution version of the same problem will be on the order of a year or more for a single simulation, making it for all practical purposes infeasible. Strong scaling, in which additional resources are used for a fixed-size problem, has been shown to have only limited success because performance quickly becomes limited by communication costs. Several of the problems mentioned above, as well as many of the engineering analyses relevant to the complete weapons life cycle, share this characterization.”
Our take on this is that after three decades of building systems to shepherd the nuclear stockpile is that the way you might want to run these NNSA applications will not scale even if the clouds will because they don’t have the right architecture. And thus, we are awaiting a novel architecture that is not here yet. Under those circumstances, we fully expect for AI to be used as a topical panacea and to grease the skids for future HPC center budgets – in the hope that it helps.
But in the end, if this doesn’t work, there is always one other option, which the NNSA report from the National Academy of Sciences also does not even bring up.
The worst thing that can happen for the high-end of the HPC business, and which former President Trump threatened to do, is to go back to physical testing of nuclear weapons. Having to pay the actual price of real post-exascale systems – 10 exaflops or 100 exaflops or 1,000 exaflops – might push the United States back to doing that again. So it is important that running NNSA simulations on the cloud is now a lot cheaper – economically, politically, and socially – than unleashing real mushroom clouds.
Our hunch is that it was always a lot cheaper in the economic sense to physically test nukes, and the desire to slow the proliferation of nukes by making an artificial computational barrier was a good thing for the world as well as for the HPC business. May it continue.
I think that the post-pandemic economic context may be partly to blame for the lukewarm (maybe slightly pessimistic) outlook of the National Academies on government-sponsored industry-partnerships for HPC efforts. In more normal (optimistic) times, HPC is a great storefront showcase for a private company’s crown computational jewels — outstanding publicity, for which one accepts not receiving major income (on-balance, it is nearly free pub.). I’d have to look back at recent TNP articles to check, but would expect (for example) AMD to be displaying a very large smile, as it makes its way to the bank, after Frontier’s success — and this smile may have extended beyond ear-to-ear, a full 360 degrees, in standard (optimistic) economic times! IBM feels a bit like sour grapes today, whilst they really should focus on promoting the uniquely apt heterogeneous composable networked cephalopod potential of their tech (SerDes POWER10). Intel is just about out of its hubris-induced quicksand gutter, and should soon feel the uplift of a successful Aurora. The pandemic modulated this positivism (into slight depressiveness), but we beat it good! Real good! Dead into the ground! Clean off! That punk stood no chance against the human species and its many spirits! Let’s get back to business folks!
AMD won for sure, politically and PR wise, but HPE made zero money on Frontier. Zed. IBM probably lost money on Summit and Sierra, but it was a PR coup for Nvidia and IBM for sure. Not making money is a problem. That hasn’t changed in the many decades I have watched this. It is just that we are now talking about an order of magnitude more cost for a capability-class machine and there are not enough companies rich enough to do this beneficent work.
Point taken! Too much Zed and one’s ded (lesson from Marsellus Wallace and Butch Coolidge’s biography: “Pulp Fiction” — eh-eh!).
Phew, this whopper is quite the cognitive gut bomb! We’re not in 1972’s Kung-Fu anymore Dorothy … this is past Brandt’s 1977 multigrid looking glass (that Rude et al., 2018, show beats PCG at memory access balancing in their Fig. 4; ref. 1 on whopper p.45), beyond even the arithmetically intense fast multipole kung-fu (druken-style?) aimed at rebalancing comps & mem access further (same Rude paper). HPC battle royales may want to resynch on those modernized multiscale choreographies (partly quadtree-based), as appropriate (per TNP’s article on Jack Dongarra’s Turing Prize).
Willcox et al.’s 2021 slider (just 3-pages) pre-flavors many of the whopper’s tasty bits (ref. 12 on whopper p.54) with spicy “serendipitous models” that blend forward and inverse computational physics, with horror-backpropagation, and machine learning sauce. It seems that sciML is like a cookbook for this sorcery, with plenty of recipes in Julia (not quite Childs) language (if I understand). French boffins are clearly favored by this futuristic outlook of post-exascale kung-fu gastronomy. We can only hope that, unlike the French GPT (J’ai pété), it doesn’t provoke silent but deadly finger pulling contests (if any).
It seems then, that dialectically, TNP oracles were right for some time on this one (in agreement with NNSA); from thesis: Computational Physics Rulez!, and antithesis: AI/ML is the Bees’ Knees!, the NNSA Boffins’ synthesis is: Both, carefully blended together in a roux, and baked into a piece-montée of kung-fu soufflés. Strong and efficient, yet tender and harmonious. Just watch-out for frog-legs stiking out … with bat eyelids.
Picture the post-exascale kung-fu gastronomy revolution postponed, as the requisite cookware is either tied up in street protests against pension reform, or confiscated by French law enforcement (eh-eh)!
If financial institutions and big ecom. don’t use the cloud for critical operations due to security concerns, you want top secret nuclear weapon simulations to migrate to the cloud?!!
All is needed is an adjustment if the pricing strategy: a 5-7 year contract (recurrent revenue) instead of a fixed pricing to increase the LTV. That’s it.
I don’t “want” anything in particular, but rather am trying to describe the situation and possible scenarios for future outcomes.
When you test a nuclear reaction you also test it’s impact on materials. Many new materials have been invited as of the last explosion and it might be a good idea to understand how those new substances will react to a nuclear reaction. I do not think it is posible to fully predict how they will react under test without the real thing.