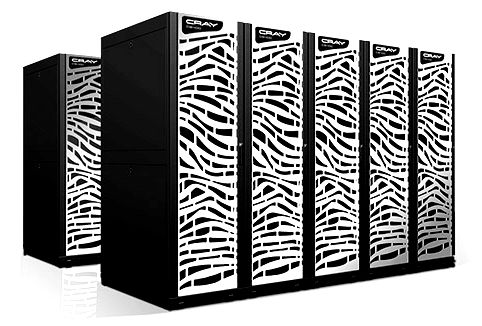
Most companies in the HPC space have made the natural transition to moving into the deep learning and AI space over the last couple of years. As one might imagine, supercomputer maker, Cray is no different and in fact, saw the writing on the wall for this movement well in advance.
While there are plenty of HPC shops that are familiar with high performance hardware, AI is a new frontier in terms of frameworks and workflows. Even though clusters from Cray’s CS line might be standard, pulling together all the elements needed for training and inference takes some rethinking in terms of data and model development, using and maintaining frameworks like TensorFlow for training, and keeping consistent environments across both experimental and production workloads.
With this in mind, this morning at the International Supercomputing Conference (ISC18), Cray announced yet another step toward on-boarding AI applications on its systems with the Cray Urika AI and Analytics software suite for its CS line of supercomputers.
The company also made updates to its AI software frameworks to fit with the Cray Accel AI reference configurations which will allow users to run in both prototype and production modes with packaged support for its ClusterStor strage platform along with the new Urika CS AI and Analytics software environment.
The AI and Analytics suite includes tooling for both data analytics (which is how the Urika line developed at Cray initially before it stopped being a hardware appliance for graph analytics and now exists solely in software—the Cray graph engine–primed for Cray systems) and AI. This means it has been engineered with Spark at the center and languages for data analysis like R and Scala along with libraries to push analytics into machine learning territory like MLlib.
The Cray Urika-CS suite includes the Cray Distributed Training Framework – originally developed for Cray XC Series supercomputers running Cray’s Urika-XC AI and Analytics suite. The company says this simplifies and reduces the time associated with configuring and running TensorFlow-based distributed neural network training and can reduce the time required to train deep learning models by leveraging the supercomputing infrastructure available on Cray CS series systems.
Cray has also developed its own Programming Environment Machine Learning plugin (CPE ML). Once exclusive to the Urika-XC software suite, this plugin — delivered in conjunction with TensorFlow that leverages Cray’s custom Aries interconnect to allow deep learning scaling to more than 500 nodes on both CPUs and GPUs on the high-end XC line. For the CS line of systems using this software suite. Cray the CPE ML plugin can bring down complexity by doing things like automatically defining which nodes to use, removing the burden of determining how many to use and where to put them.
On the hardware front, the reference configurations Cray has selected are not surprising for the workload and speak volumes about what the company thinks production customers will be looking for when it comes to high performance clusters. These reference configs come standard with CS500 GPU nodes in a single-rack config with either Intel Skylake or AMD Epyc or ThunderX2 as processor choices (wow) along with Pascal GPUs and Cray’s own all-flash arrays, the ClusterStor L300F and L300N, a Lustre-based flash products.
Cray’s entry to the all-flash Lustre array business this week. As the company noted, it is a flexible storage system that will offer heterogeneous building blocks and support for new Lustre 2.11 functionality. Packing 24 SSDs into a 2U enclosure for AI users who are often running large volumes of small I/O workloads. The existing L300 model is an all-HDD Lustre solution, well suited for environments using applications with large, sequential I/O workloads. The L300N model, by contrast, is a hybrid SSD/HDD solution with flash-accelerated NXD software that redirects I/O to the appropriate storage medium, delivering cost-effective, consistent performance on mixed I/O workloads while shielding the application, file system and users from complexity through transparent flash acceleration.
Even with the software story fleshed out, it is difficult to tell how customers will make big AI cluster purchases. For true specialization of performance, Cray’s XC line of high-end supercomputers might be worth the spend, especially when bolstered with the software stack, GPUs and speedy interconnect needed for some AI workloads. The CS line of standard clusters will have to compete against the much broader server market, which means Cray will have to keep innovating in software to keep ahead of its competitors—something newer for a company that has differentiated based on its hardware assets (interconnects in particular). Nonetheless, the reference config announcement today shows where demand is—or might be-heading as we round into the second half of 2018.
Be the first to comment