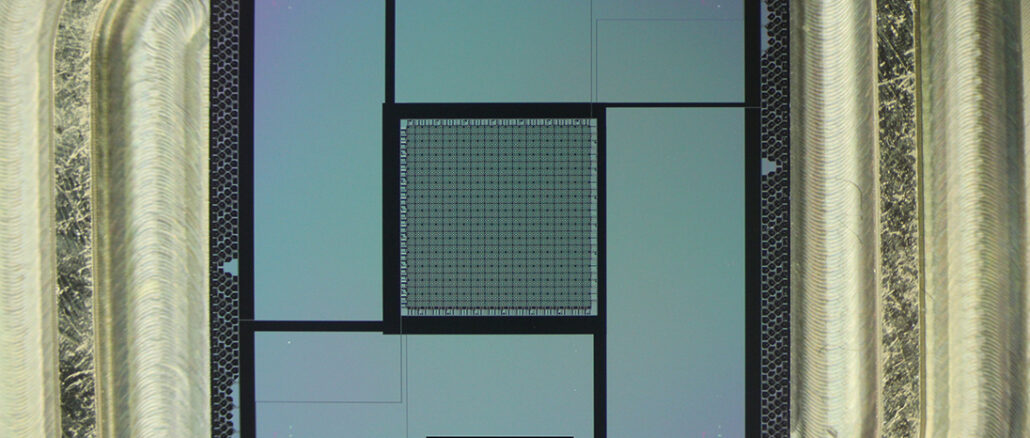
There is a seemingly endless list of problems to be solved and issues to be addressed in vendors’ steady march toward quantum computing. Researchers in Europe say they recently solved a “decades-old controversy” and used GPU-laden supercomputers running simulations to do it.
In the research published earlier this month in the science journal Nature, the scientists looked into a highly specific and complex but important puzzle involving quantum annealing, a method of quantum computing that for more than two decades has been leveraged primarily by D-Wave Systems to develop what right now are the only commercially available quantum computers on the market.
Almost every other vendor delving into quantum computing – and that includes such companies as Microsoft, Google, IBM, Honeywell, Atos, and Amazon, to name a few – is pursuing superconducting gate-model system. D-Wave early on in its existence latched onto annealing technology, which company executives said allow the vendor to more quickly get systems into the market.
D-Wave announced in 2021 that it is building its own gate-model quantum system, its annealing systems remain the cornerstone of its operations. The company this year unveiled its Advantage2 quantum processing unit (shown in the feature image above) that is now available in its Leap cloud service. In addition, D-Wave has its new fast-anneal protocol in all of its QPUs in Leap and, at its user conference last month, introduced its latest quantum-classical hybrid quantum solver to help solve increasingly complex nonlinear optimization problems.
The quantum annealing method isn’t one that can be used to solve complex computing problems, but it is particular good at address optimization issues in everything from workforce scheduling to supply chain management.
Looking For The Stable State
The problem the European researchers wanted to address focused on how the properties of magnetic particles arranged in a two-dimensional plan can suddenly change their behavior.
According to Esperanza Cuenca Gómez, developer relations manager for quantum computing at Nvidia, quantum annealers work by methodically decreasing a magnetic field applied to what she called a “set of magnetically susceptible particles. When strong enough, the applied field will act to align the magnetic orientation of the particles – similar to how iron filings will uniformly stand to attention near a bar magnet.”
If scientist can vary the strength of the field slowly enough, those magnetic particles will arrange themselves in a way to minimize the energy of the final arrangement, Gómez wrote in a blog post.
“Finding this stable, minimum-energy state is crucial in a particularly complex and disordered magnetic system known as a spin glass since quantum annealers can encode certain kinds of problems into the spin glass’s minimum-energy configuration,” she wrote. “Finding the stable arrangement of the spin glass then solves the problem.”
Knowing this, scientists can create better algorithms for addressing highly difficult problems. They can mimic how nature deals with complexity and disorder, which is important for enabling quantum annealing and the applications it runs to solve computationally complex problems that can’t efficiently be solved by classical computers in a range of areas, like logistics and cryptography.
According to Gómez, the research also highlights a key difference in quantum computing methods.
“Unlike gate-model quantum computers, which operate by applying a sequence of quantum gates, quantum annealers allow a quantum system to evolve freely in time,” she wrote.
Understanding The Phase Transition
The researchers, led by Nobel laureate Giorgio Parisi, wrote that their work is a key step forward in understanding how to build quantum annealing systems that can more quickly solve computational problems that would take classical systems significantly longer to address.
“Just as in metallurgic annealing, in which a ferrous metal is slowly cooled, quantum annealers seek good solutions by slowly removing the transverse magnetic field at the lowest possible temperature,” they wrote. “Removing the field diminishes the quantum fluctuations but forces the system to traverse the critical point that separates the disordered phase (at large fields) from the spin-glass phase (at small fields).”
What’s been missing a fuller understanding of the phase transition.
“All hopes of achieving an exponential speed-up, compared to classical computers, rest on the assumption that the gap will close algebraically with the number of spins,” they wrote. “However, renormalization group calculations predict instead that there is an infinite-randomness fixed point.”
Researchers Lean On GPUs
A debate among scientists centered on questions around closing the energy gap that separates the ground state from the first excited state. They used simulations running on Nvidia GPUs to solve that debate, noting that they found that both sides of the debate held some truths.
According to Nvidia, the research team used 2 million GPU computing hours at the Leonardo facility at the CINECA HPC center in Bologna, Italy, and almost 160,000 GPU hours on the MeluXina GPU cluster in Luxembourg. Another 10,000 GPU hours were run in the Spanish Supercomputing Network – a distributed computing environment of 12 interconnected supercomputers coordinated by the Barcelona Supercomputing Center – and the researchers also accessed the Dariah cluster, in Lecce, Italy.
The systems were used to simulate the behavior of a quantum annealing system.
The research comes as dozens of tech companies are developing quantum computing systems and components, with the idea that much quantum computing will be done in the cloud as a service running in hybrid environments, with quantum systems picking up the more complex computational workloads that are beyond what classical systems can do.
Within the growing quantum computing field – some projections have the global market size growing from $885.4 million last year to more than $12.6 billion by 2032 – there will be room for both annealing and gate models, according to D-Wave president and chief executive officer Alan Baratz.
“Optimization is a really important problem class,” Baratz said during D-Wave’s conference in June. “It’s low-hanging fruit for the use of quantum today. D-Wave’s annealing quantum computers are excellent at solving this class of problems. Annealing quantum computers cannot solve all problems. For example, they’re not particularly good at quantum chemistry or designing longer lasting batteries. Gate-model systems are much better for applications like that.”
Nothing like a cool game of Ising spin-the glass bottle to quench one’s transverse field Hamiltonian disorder on a hot summer day I say. Renormalization group outings, roasting quantum marshmallows on the critical point campfire, with those Griffiths–McCoy singularity singing skills, will never have quite the same-parity gaps again! 8^p
This research is a great example of how simulations on powerful machines like GPUs can help us understand complex quantum phenomena. It’s fascinating to see that both sides of the debate about the energy gap had some validity.
This bodes well for the future of quantum computing, with different approaches like annealing and gate-model potentially complementing each other. I’m curious to see how D-Wave leverages this new understanding to improve their quantum annealers.
Thanks for sharing this informative article!