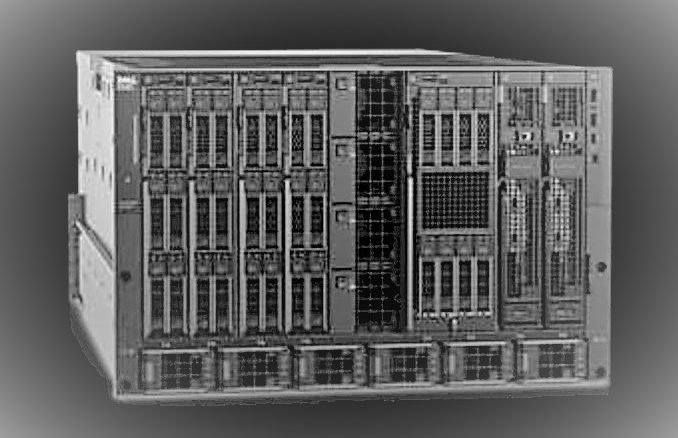
The IT industry is all about evolution, building on what’s been done in the past to address the demands of the future. In recent years, that evolution has been accelerating as organizations try navigate their way in a world that is about hybrid clouds and multiclouds, the Internet of Things (IoT) and artificial intelligence (AI), the proliferation data and the trend toward greater mobility, and the rapid proliferation of data and the extension of hardware and software out to the edge.
Architectures over the past decade or more have shifted through such movements as virtualization and hyperconverged infrastructure (HCI) and now are moving more into areas such as software-defined and the cloud, according to Ashley Gorakhpurwalla, president and general manager of Dell’s Server and Infrastructure Systems business. It is also beginning to split in two directions that, while distinct, will both be used by enterprises, Gorakhpurwalla tells The Next Platform.
“We’ve been on a journey for a while, where ten years ago, your server architectures were general-purpose; they’d been designed really to make calculations and then store it,” he says in a recent interview. “That’s what we do to compute. People started to stay, ‘Well, what if I don’t want to set up my own infrastructure and be responsible for it?’ They said, ‘I might have to put it in someone else’s infrastructure and let them do that.’ They really had these binary choices. It was either roll your own – in some ways, we tried to help them with certain aspects of automation and management and monitoring, but it was usually at the compute level and the storage level and the networking level and data protection – or they had ‘I guess I have to let someone else take care of it and I lose control,’ or there are other tradeoffs. It was more expensive, or whatever it was.”
Moving ahead, HCI and related technologies began to appear, converging compute and storage, delivering greater automation and a more scale-out architecture. It wasn’t always an easy solution, but there were more choices, Gorakhpurwalla says. Companies were no longer had to go into someone else’s datacenter – in the cloud or with a managed services provider – to reduce the time and expense needed to run the infrastructure in-house. The chore of running infrastructure on premises was eased to some degree, with some of it being automated, provisioned on day one and maintained on day two, enabling organizations to “live a little bit higher on the stack and that’s a good tradeoff,” he says. “I’m going to live with that tradeoff.”
There also came changes in the architecture around lifecycle management and security and storage started to become a paramount architecture change. With HCI converging compute, storage and networking, the localized storage layer became important to the application and to the datacenter environment.
“Networking became less of a north-south, where you went all the way up the architecture and then all the way back down,” he says. “You couldn’t pay that penalty anymore because of where storage was. So the architecture did change. We were imbalanced because we had more CPU than we had I/O.”
Things in the datacenter are continuing to change, Gorakhpurwalla says.
“You start to see that there’s two big forks in the road,” he says. “There are those who believe that the server architecture – which was all about calculations and storage and data, but we got better with monitoring and localizing and performance – is going one of two ways, and I think it’s a patchwork of both. One is much more software-defined and can we afford to have an architecture built around one node at a time and all the maintenance and lifecycle management and the capability and the stranded resources that may happen from one node at a time. Usually if you play that our far enough in the future, people will talk about composability or disaggregation of resources or resource aggregation or software-defined.”
The other path is what he calls domain-specific architectures.
“Today’s poster child for a domain-specific architecture would be a machine learning training architecture,” he says, adding that it’s “much less dependent on the old way of doing things, which was calculate some data on your host processor and throw it down to a subsystem. Now it’s about the host processor maybe just setting something up and then it’s all on the accelerator. It’s all going perhaps directly through to some new architecture of persistent memory. They are diverging away, where we’re heading toward a future of being able to redefine how we utilize our resources and be very software-defined and another one where our architecture had been about compute it and store it. Now it’s going to be about, actually, that data you stored all those years? That’s the value.”
The massive amounts already being generated, collected, stored and analyzed now are only going to grow in the future as more intelligent devices become connected and start creating even more data. The challenge is trying to not only analyze all the data to find the relevant information and insights that will drive business decisions that lead to more efficient operations and cost savings for the organization and better products and services for customers, but also to do so in as close to real-time as possible. That need for speed is what is fueling the push to bring more compute, storage, networking, analytics and AI and machine learning capabilities out to the edge, closer to where the data is being generated and where much of the effort around architectures and infrastructures is being directed.
According to Gorakhpurwalla, there are a number of technologies that will cross over from the two kinds of architectures, the software-defined approach and domain-specific model.
“In my future of composability, disaggregation, and resource utilization, we need a protocol and a transport layer that can actually pull off having resources not stranded or bound to a processor, but capable of being utilized by many,” he says. “That’s why we started the work on Gen-Z a few years ago.”
Gen-Z is an emerging networking fabric protocol that is promising higher throughput and lower latency. The aim is to drive faster connections between chips, accelerators, main memory and fast storage, fueling the development of more powerful servers that can pack even more cores and accelerators and process more data. Dell has been a vocal proponent of Gen-Z and in a demo at the Dell Technologies World show in early May, the company showed off a PowerEdge MX server – a cornerstone of Dell’s Kinetic composable infrastructure efforts – running Gen-Z within the infrastructure framework.
“This is starting now to make things at the end of the wire just as important as the processor and we have made them first-class citizens of the architecture,” he says. “But Gen-Z is also going to be incredibly important in machine learning and being able to put architectures together that are big enough to do things that we haven’t even dreamed of, in terms of training and learning.”
Technologies like Gen-Z will help fuel changes in the infrastructure that are needed to address modern workloads. Traditional architectures featured servers with software where the data was brought in, software rules were written and then answers from the data were returned based on those rules, Gorakhpurwalla says.
“What we’re thinking about going forward is an architecture that’s different,” he says. “I bring data to you, but probably to an accelerator. I can tell you the answers and what I want back from you is the rules. That’s the machine learning training model. Then I go to deploy that in inference engines across the edge – under the private edge or the service provider edge – and then those architectures extended either in a cloud operating model, my datacenter, where the data is being held, protected, stored and utilized and then [there is] action at the edge and more data coming in.”
There’s a role for each path in the datacenter and for systems in each path, he says, pointing to the MX server, which is both a highly secure and automated high-performing blade architecture and it also will play a key role in such future architectures as composability.
“You can see where we designed it for a future that is about disaggregation, about Kinetic architectures, about Gen-Z,” he says. “If you put a midplane in there, you know you’re about to invest in developed protocols and transports that don’t exist today but will in the future. And you set up the fabrics to be smart and ready for a future in which you can bind all of this together. That’s an example of how we’re going to see these future technologies start to incorporate themselves for these next few generations. But we need software to catch up. We need everything in software – the hypervisors, the kernels, the management frameworks – to take advantage of some of these technologies we’re putting in place.”
Enterprises will be adopting both paths and Dell is looking to leverage its broad capabilities to address each. For example, at Dell Technologies World, the company in tandem with VMware unveiled Dell Technologies Cloud, which includes an on-premises cloud platform and a datacenter-as-a-services offering. At the same time, Dell announced the DSS 8440, a 4U, two-socket server optimized for machine learning workloads and featuring not only Intel Xeon CPUs but also from four to 10 Nvidia Tesla V100 Tensor Core GPUs, NVM-Express and SATA drives and a high-performance switch PCIe fabric.
The system initially is aimed at training workloads, though the ability to run inference tasks will come down the road.
Be the first to comment