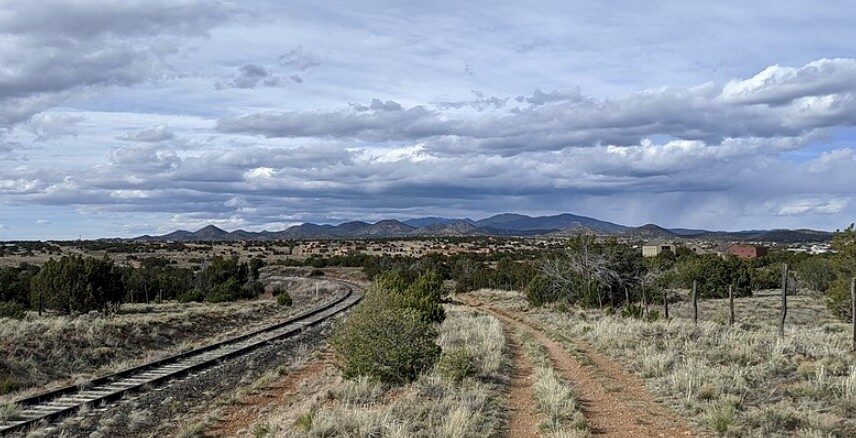
A theme snaking its way through conversations these days about generative AI is the need for open source models, open platforms, and industry standards as ways to make the emerging technology more accessible and widely adopted by enterprises.
There is a growing number of open source models – or models that their developers claim is open source – that are coming onto the scene, from Stable AI’s Stable Diffusion 3 to Meta Platform’s Llama 3 to several from Mistral AI. OpenAI, which started the explosion around generative AI with its release of ChatGPT in late November 2022, open source its GPT-2 model, and Elon Musk is not only open sourcing X.ai’s Grok large language model but is also suing OpenAI – he was an initial investor – for not being open enough.
Hugging Face has become a repository of open source generative AI models and other resources – a sort of GitHub for that world – and Open Source Initiative is developing a definition of open source AI, a community effort with the latest draft being 0.0.8.
“AI systems are growing more complex and pervasive every day,” OSI wrote when defining the need for a definition. “The traditional view of open source code and licenses when applied to AI components are not sufficient to guarantee the freedoms to use, study, share and modify the systems. It is time to address the question: What does it mean for an AI system to be open source?”
Meanwhile, chip makers and other tech vendors are boasting of their open architectures, open platforms, and industry-standard approaches. At Intel’s Vision 2024 event last month, Intel chief executive officer Pat Gelsinger said that “now’s the time to build open platforms for enterprise AI. We are working to address key requirements that include how you efficiently deploy using existing infrastructure, seamless integrated with hardened enterprise software stacks that you have today, a high degree of reliability, availability, security, support issues.”
AI Opportunities And Risks
Red Hat at its Red Hat Summit this week in Denver is doubling down generative AI, with the bulk of its more than two-dozen announcements centering around how it’s infusing its portfolio with generative AI capabilities and how the enterprise trends in AI are bending toward hybrid architectures and open standards, which are in the company’s wheelhouse. The rapid pace of innovation is presenting organizations with both opportunities and risk, according to Steven Huels, vice president and general manager of Red Hat’s AI Business Unit.
There is “a lot of opportunity in the form of open models now that have come along that are rivaling their proprietary or cloud counterparts with accuracy, speed and capability to be deployed across multiple footprints,” Huels said during a call with journalists and analyst in the run-up to the show. “At this point, customers know that AI’s not going to be defined by single footprint. They know they need a hybrid AI story. Again, those open models, they represent an opportunity to move models on-prem, use existing CapEx and control costs. They’re becoming equally viable to their cloud counterparts.”
The industry model has been to send AI workloads to the cloud, where the massive compute power can churn through the data and return the information. For enterprises, that is getting more difficult to do. Moving data back and forth between on-premises datacenters and the cloud can get expensive and, for security, privacy, and regulatory compliance reasons, some data can’t be sent to the cloud, particularly as they use methods like retrieval-augmented generation (RAG) to infuse AI models with sensitive and proprietary corporate data.
“There’s rapid evolution across all the platforms and the type of tooling that’s being made available,” Huels said. “There’s always a new methodology that’s being presented for customers to be able to infuse their data into different models. Customers are looking to be able to perform inference across cloud, on-prem and edge. As these models have gotten more efficient, processors are starting to pick up in the capabilities they have, and hardware at the edge is becoming more available. This isn’t going to be centrally datacenter-driven for their entire AI workload. They may train on the datacenter, but then they want to be able to deploy across multiple platforms.”
Like other platform vendors, Red Hat over the past few years has been integrating AI capabilities into its products and services, such as Ansible. However, at Red Hat Summit, the company is looking to showcase what it already has and what is coming. Red Hat is expanding the use of its Lightspeed AI assistant tool, which was first introduced last year with the Ansible automation platform and is now coming to both OpenShift container platform and Red Hat Enterprise Linux (RHEL) operating system, with future plans to extend it to other products, according to Gunnar Hellekson, vice president and general manager of the RHEL Business Unit.
“The goal here is very similar to what we’ve already delivered with Ansible lightspeed; it’s to provide a generative AI assistant integrated into the OpenShift web console so that users can use simple language to get answers to questions related about OpenShift, all of the other pieces of technology that are associated with an OpenShift subscription, [and] help with troubleshooting,” Hellekson said. “We’re still in the planning stages with, Red Hat Linux Lightspeed. We are developing the technology. But overall, our goal is to really to increase productivity and efficiency, to help customers adopt AI.”
With the latest version of the OpenShift AI, a hybrid and open AI and machine learning platform, organizations can deploy models to the resource-constrained and air-gapped edge environments via a single-node OpenShift, and improved model means that enterprises can run both predictive and generative AI on a single platform, which helps cut costs and simplify information, according to Sherard Griffin, senior director of global software engineering at Red Hat.
“This allows you to do not just deployments of LLMs into massive scale, but also being able to handle natural language processing and other tasks,” Griffin said. “We get those out-of-the-box capabilities that allow the customers to pick and choose whether you’re running vLLM for runtimes in one place or maybe you have a custom runtime that’s optimized for specifically your use case. You have that flexibility to really quickly and seamlessly choose the right runtime for your models, whether it’s predictive or GenAI.”
Red Hat also is rolling out Podman AI Lab, making it easier for developers to build, test, and run generative AI-based software in containers on their workstations using a graphical interface, adding to the industry-wide push to enable AI applications to run locally on devices rather than having to send the workloads to the cloud. It’s part of emerging edge AI trend to better AI-enable Internet of Things (IoT) and other edge devices to reduce latency and save money.
Podman AI Lab is an extension of Red Hat’s Podman Desktop, an open source graphical tool for developers who want to build applications in containers and on Kubernetes.
Other new offerings include image mode in RHEL for deploying the platform as a container image and automated policy-as-code coming to Ansible to enforce policies across hybrid cloud environments, which will help organizations adapt to the changes brought by AI. Red Hat also is unveiling a range of AI-infused partnerships with the likes of Run:ai, Pure Storage, Stability AI, and Elastic for RAG use cases.
All of these developments add fuel to Red Hat’s argument that AI workloads will gravitate to open and hybrid environments, Huels said.
“A lot of predictive AI has been out there for the last 30-, 40-some odd years, and that was largely dedicated to specific departments,” he said. “In the move toward generative AI, customers are now expecting everyone across their organization to interact or be impacted and involved with AI. This means they do not want a siloed system. They want an enterprise-wide system and because nobody’s just getting unlimited headcount and budget, they want that enterprise system to be able to coexist and integrate with their existing AI investments. They’re looking at what can be their enterprise AI platform. This means to having predictive AI workloads work right alongside with GenAI workloads, knowing that there’s a difference in the type of footprint and requirements and resources they have. They still want to be able to govern and control a lot of the underlying platforms and resources that are being used for AI.”
… and (just announced) RHEL is the OS running the swashbuckling old-spice stronger swagger of El Capitan ( https://www.redhat.com/en/about/press-releases/red-hat-helps-power-future-supercomputing-lawrence-livermore-national-laboratory ) … this instead of the more traditional flair and extravagance of a big wig and feathered hat ( https://www.worldhistory.org/article/1839/pirate-clothing-in-the-golden-age-of-piracy/ )! q^8