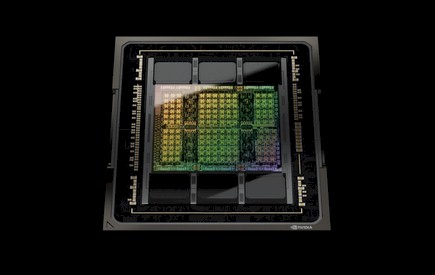
If you want to get the attention of server makers and compute engine providers and especially if you are going to be building GPU-laden clusters with shiny new gear to drive AI training and possibly AI inference for large language models and recommendation engines, the first thing you need is $1 billion. After that, things will be easier for you when it comes to building out your next platform.
If you don’t have $1 billion, then you will be fighting to beg, borrow, and steal rent capacity on older GPU systems that can run AI workloads reasonably well and waiting for your turn to get access to capacity for newer and more powerful GPUs on the clouds or for your order to be fulfilled from an ODM or OEM, which will probably take longer than you are used to.
To be fair, you can probably get pretty good service for a deal that is $250 million or more. It is not like you are a total loser if you only have a few hundred million bucks to spend. Or, given the number of AI startups out there with a similar cash pile and a dream, maybe you will be. The good news is that AI is one of the few areas where venture capitalists, hedge funds, and independently wealthy investors are still willing to pump in some cash to get in on the AI action. So if you have a great pitch, and an angle of your own, then you have a shot at the bigtime.
Inflection AI, a startup that has just raised $1 billion in capital in its second round of funding and that was only founded in March 2022, is setting the new bar for how far an AI startup has to scale in terms of money, machines, and software stacks to increase the odds for competing in – much less surviving in – this Dot Cog Boom.
And it is not just raising money, it is building a massive system of its own, based on Nvidia “Hopper” H100 GPUs and Quantum 2 InfiniBand networking, that will be among the most powerful systems on Earth.
You better bring your A game if you want to play AI.
LinkedIn Like Crazy
Inflection AI has three famous founders and, at least according to profiles on Crunchbase, a fourth co-founder who appears to be in important companies in the AI field who was not identified on its site, just like he was not identified as one of OpenAI’s co-founders either. This person – Carlos Virella – does not have much of a vapor trail, and could be a Forrest Gump-style send-up for all we know. But the other three founders of Inflection AI – one of whom was also a co-founder of OpenAI – are revealed. These three are hooked into the Web 2.0 startup community and were a big part of the AI revolution that started a decade ago, and the combination of the two are the reason that Inflection AI has launched the personal AI service called Pi.
Reid Hoffman, who is well known as one of the co-founders of the LinkedIn corporate network, started becoming a player in Silicon Valley when online auctioneer eBay shelled out $1.5 billion to buy online payments processor PayPal in August 2002. Hoffman was on the board of directors of a company called Confinity when it was founded in December 1998 and was named executive vice president in charge of all external relations with the company in January 2000. Three months later, Confinity merged with another company called X.com. Elon Musk was a co-founder of the latter, and Peter Thiel was a co-founder of the former, and when the resulting PayPal went public in February 2002, raising $61 million and representing one of the few bright spots during the Dot Com Bust. Hence the eBay acquisition only six months later.
In January 2003, Hoffman co-founded LinkedIn and was its chairman and chief executive officer and started running its products as well as being a partner at Greylock Partners in the fall of 2009. In June 2016 Microsoft spent $26.2 billion to buy LinkedIn, and Hoffman was the controlling shareholder and made $2.84 billion himself on the deal. Hoffman is still at Greylock, but it is his personal money that is helping fund Inflection AI alongside an investment from Microsoft co-founder Bill Gates, former Sun Microsystems and Google executive Eric Schmidt, as well as Nvidia and Microsoft and a small GPU compute cloud builder called CoreWeave. Hoffman is on the board of directors of OpenAI and Microsoft, and stepped down from OpenAI back in March because of a conflict of interest with Inflection AI. It is hard to believe Hoffman will be on the Microsoft board for much longer given the tight partnership the software giant has with OpenAI, but then again, Microsoft is investing in Inflection AI as a hedge it looks like anyway. So maybe there are too many conflicts of interest and they cancel out like a double negative.
Karén Simonyan, chief scientist at Inflection AI, and Mustafa Suleyman, its chief executive officer, are long-time AI researchers who have built successful companies and sold them.
Simonyan was at the University of Oxford when he created the VGGNet image processing framework in 2013, which was commercialized through a company called Vision Factory AI and quickly snapped up by a secretive AI startup called DeepMind Technologies, where Simonyan became principal research scientist. DeepMind was founded in June 2010 and Suleyman was chief product officer and then head of applied AI when Google entered the scene and snapped up DeepMind shortly after DeepMind bought Vision Factory. Facebook – it wasn’t called Meta Platforms yet – had tried to buy DeepMind in 2013, and Google paid somewhere between $400 million and $650 million, depending on which rumor you want to believe, for DeepMind’s reinforcement learning AI technology, which was famously used to master video games among other things. (Musk and Thiel invested in DeepMind, of course.) The DeepMind team also created the Chinchilla large language model that has set the pace for a number of years innovation in this area before being eclipsed by the GPT-4 model of OpenAI/Microsoft.
If you are trying to keep track of the connections, Suleyman has been a partner at Greylock since January 2022, and it is easy to see that Hoffman and Suleyman cooked up Inflection AI in the hallway. Greylock was a key investor when Inflection AI raised $225 million back in May 2022 when Microsoft, Hoffman, Gates, and Schmidt kicked in some dough alongside Mike Schroepfer, Demis Hassabis, Will.i.am, Horizons Ventures, and Dragoneer. Nvidia and CoreWeave were new investors when the second bag of money for Inflection AI, weighing in at $1.3 billion, was raised at the end of June.
AI At An Inflection Point
Inflection AI has the goal of making its Pi – short for personal intelligence – AI assistant available to everyone on the planet. It would be interesting to calculate just how much computing oomph such an endeavor would take and how it could possibly be affordable given the high cost of running LLMs to answer silly questions. But set that aside for a moment.
To achieve its goal, Inflection AI has done three things: Have co-founders who know how to do AI and who have big bucks and friends with big bucks as well as ambitions to get rich on AI. And so, Inflection AI has built its own LLM – the first iteration is called Inflection-1 – and, like OpenAI, will be investing a fortune in the hardware necessary to drive the accuracy of that model and scale it across an ever-larger number of users of the Pi service.
This is going to take an enormous amount of money, considering the high cost of compute engines for training LLMs. Hence the big raise in June.
Working with Nvidia and CoreWeave, Inflection AI ran the MLPerf reference training benchmark in 11 minutes on its Inflection-1 LLM spanning 3,500 Hopper GPUs. We don’t know how many GPUs were used to train the Inflection-1 model that underpins the Pi service, but it is probably a larger number than this and it was probably using Nvidia “Ampere” A100 GPU accelerators if we had to guess.
What we do know is that Nvidia and CoreWeave are working together to create an AI cluster in the cloud that will have over 22,000 H100 GPU accelerators training what we presume will be the Inflection-2 LLM. Inflection AI boasts that these 22,000 H100 GPUs would deliver “a staggering 22 exaflops in the 16-bit precision modem and even more if lower precision is utilized” and goes on to say that if the High Performance Linpack (HPL) matrix math test was done on it, like an HPC supercomputer, it would rank second on the current Top500 list.
It’s time to fire up the Excel spreadsheet, people.
If you do the math on 22,000 GPU accelerators using Hopper PCI-Express cards, which are commonly used by the hyperscalers and cloud builders to create their clusters – rather than the HGX-style boards with NVLink Switch interconnects between a pod of four or eight GPUs and using the SXM5 variants of the Hopper GPUs – then at FP16 precision on the Tensor Core matrix math engines, you get 16.9 exaflops without sparsity support and 33.8 exaflops with sparsity support turned on. You also get a cluster rated at 572 petaflops using the FP64 double precision vector math units on the H100. That doesn’t match the 22 exaflops at FP16 precision that Inflection AI is talking about in its announcement.
And here is where it gets interesting. If you do the math on 22,000 H100 SXM5 GPU accelerators that are going to use NVLink Switch interconnects within the server enclosure, then you get a cluster with an aggregate peak performance of 21.8 exaflops at FP16 precision with sparsity not activated. (Which tells you something about the Inflection-1 LLM perhaps in that it may not be using a lot of sparse data.) That rounds up to the 22 exaflops Inflection AI is talking about, and that also yields a supercomputer with 748 petaflops of peak FP64 vector performance and 1.47 exaflops with FP64 running on the Tensor Cores matrix engines. (As far as we know, not a lot of HPC applications have been ported to the Tensor Cores.)
Assuming a 65 percent computational efficiency on the Linpack benchmark, this cluster would be rated at around 486 petaflops on the Top500 rankings, which would place it just above the 442 petaflops rating for the “Fugaku” supercomputer at RIKEN Lab in Japan, ranked second in the world, and considerably below the 1.19 exaflops rating for the “Frontier” supercomputer at Oak Ridge National Laboratory in the United States, which is ranked number one for the moment. The “Aurora A21” system at Argonne National Laboratory is expected to bet a just over 2 exaflops peak when it is delivered later this year using Intel CPUs and GPUs, and the “El Capitan” system at Lawrence Livermore National Laboratory also coming later this year will be well above 2 exaflops peak. using AMD’s hybrid Instinct MI300A compute engine. And both should be well over three times as powerful at FP64 measures compared to this Inflection AI rental on the CoreWeave cloud.
Which brings up an important point. For a cloud machine to be considered against an on premises machine, you have to have it running all of the time for a single customer. We think this will be the case for the Inflection AI machine, and there is even a chance that the company will run Linpack on it just to make a point and actually get on the Top500 list in November. But if Inflection AI is not using the machine almost continuously – we want to say absolutely continuously like the machines at the world’s HPC labs are used, but maybe saying 75 percent or 80 percent of the time is enough – then it should not be counted as a distinct machine in its own right.
And that brings us to the next point. What does this massive amount of capacity cost? Let’s take a stab at this starting with the cluster using the H100 PCI-Express cards. Assuming these H100 PCI-Express cards cost around $20,000 a pop, that is $440 million at what we think is close to current street price. (It is hard to say what it really is.) It takes 2,750 nodes to house all of those GPUs with eight per enclosure. With a pair of beefy CPUs, 2 TB of memory, and 34.5 TB of NVM-Express flash for data and operating system storage (a total of ten drives, two skinny ones for the OS) will probably run somewhere around $150,000 each. That is another $412.5 million. InfiniBand networking, as Nvidia is fond of saying, is another 20 percent on top of this, or $213 million, which drives it up to $1.07 billion.
Now, let’s look at the SXM setup. Same 2,750 nodes, but this time you have a GPU that might cost around $30,000 a pop, so 22,000 of them is $660 million. The nodes have another $25,000 or so in additional NVLink Switch costs inside each chassis, so each node costs around $175,000 each, for an additional $481.3 million. The clusters would have the same $213 million or so in InfiniBand costs outside of the chassis. Now you are up to $1.35 billion. This looks like the scenario Inflection AI is talking about for the cluster it is building with CoreWeave and Nvidia.
This is a lot more money than the US government is shelling out for its exascale class supercomputers, which cost on the order of $500 million to $600 million at a significant discount off of list price, with non-recurring engineering (NRE) costs added in.
The cloud overhead, which includes system management, real estate, and electricity costs could easily add another $200 million, plus some 5 percent profit margin for CoreWeave would boost the cost to $1.63 billion. That’s a little bit more than the $1.53 billion that Inflection AI has raised so far from investors. Luckily, with the cloud, it doesn’t have to pay it out all out once, like a giant reserved instance. Or, maybe it does and that is why the price won’t be higher than this. (can you imagine paying on demand for such a cluster?)
What is the price of a Pi subscription, and how many users does it have to have – and how quickly – to pay for this? Bill Gates has enough money to not have to care, and so does Nvidia and Microsoft. Maybe Reid Hoffman, too. But you can rest assured that all of this math has been done.
All we know is that the monthly cost of running the training and inference for the Pi service has to eventually be a lot less than the price of the Pi service to end users for any of this to work out.
Up next, we are going to take a look at the performance of the Inflection-1 LLM compared to its peers.
Nice article! A billion dollars is a high-entry price-point and it is quite impressive that it can be provided by VCs (very deep pockets I guess) when individual States (eg. UK, in the El-Reg piece about them possibly rejoining EU science efforts) might struggle to compete. On the other hand, United States (of America, and “almost” of Europe) seem to have budgets appropriate for this type of activity. Speaking of which: “Happy Independence Day” for the U.S. — the 1776 Revolution (against the Brits) was helped by Louis the XVI (of the Bourbon house in France), after whom Bourbon Whiskey is named as a tribute. Inspired by this, France had its own Revolution 13 years later (1789), and ipso-facto guillotined Louis and his Marie-Antoinette — this will be celebrated in a couple of days (July 14). It however took until 1871 (or 1875) for the French Republic to become a Democracy, which happened after the “Paris Commune” (Emperor Napolean was no democrat).
$500M for an ExaFlopper (at 20MW) looks like a great deal, scaling down to $500K for a PetaFlopper, that compares favorably to 2008’s Roadrunner that did a PF for $100M (at 2.4MW)! Outfits like Inflection could (theoretically) rent-out the equivalent of 572 simultaneous Roadrunners, to their clients … (wow!).
When you say it that way, it sounds like a deal…. And based on what the AI machinery costs, it is!
… and you can solar-power a PetaFlopper today (O’Henri’s Xeon+H100 cranks 2.9 PF at 44 kW = 4 houses of photovoltaics)!
You always have such an interesting write-up, thanks Mr Morgan. The math gets a little easier when one considers Nvidia likely bartered for equity . . . H100s are worth quite a bit more than their weight in gold I hear. I also wonder if Ms. Grace and/or her offspring make an appearance in the Inflection AI solution — I don’t think we’ve seen the full potential of the Hopper silicon realized yet.