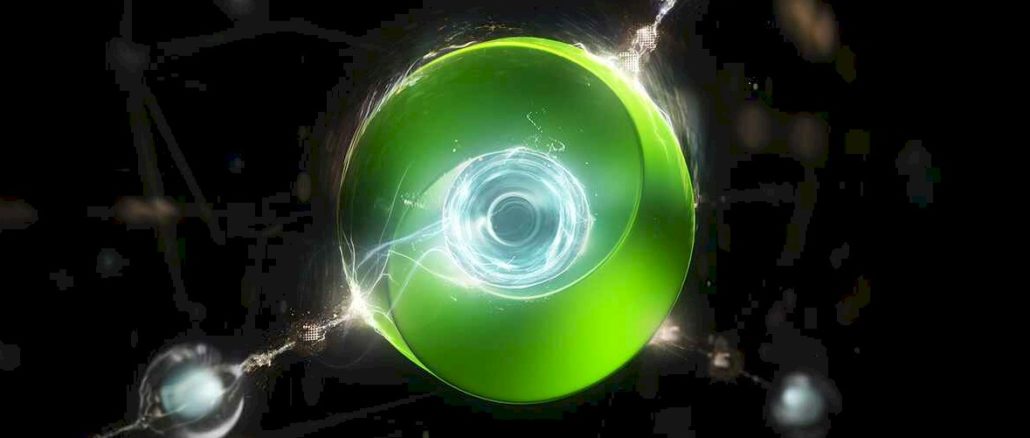
All of the great technologists live in the future. They bring it back to us with the help of countless engineers who derive the specifications from their vision and make ideas into reality and, ultimately, into money to repeat the process again.
Nvidia has been in such a virtuous cycle of creation and expansion for the past decade and a half, and we got the chance to sit down with Jensen Huang, co-founder and chief executive officer at the company, to talk about the state of the datacenter and how the emergence of what Nvidia calls the Omniverse – a kind of mashup of simulation, artificial intelligence, augmented reality, and virtual reality – will change the nature of our world and the computing within it.
Timothy Prickett Morgan: I like real reality and I don’t have a lot of patience for virtual reality and only slightly more for augmented reality. But back at the spring GTC 2021 event, I watched the video of the BMW factory of the future, and the potential of the Omniverse stack you are building finally seemed relevant as far as I was concerned. I finally understood what a digital twin was really about – people talk about digital twins in such a nonsensical way sometimes. I get the idea that everything will have telemetry, and then you can take computers and overlay that telemetry onto the world to do things with that information.
So my first question is: How big is this Omniverse opportunity?
As Ian Buck, general manager of accelerated computing at Nvidia, recently explained to me, HPC at supercomputer centers and AI and data analytics at hyperscalers have all been isolated pockets, but Omniverse, in the largest sense, is the commercialization of all these things, all at once, as one stack. I thought that was profound. With those separate buckets, you have built a $10 billion a year datacenter business that is growing nicely. Will Omniverse grow that Nvidia datacenter business?
Jensen Huang: Yes. You said a lot of things that were all very sensible, and they were all spot on. Here’s the thing: there won’t be one overlay to Earth as you are seeing it. There will be millions of overlays and millions of alternative universes. And people will build some, but AI will build a lot of them. Some of these Omniverse worlds will be models of our own world, which is the digital twin; some will model nothing like our own world. Some will be temporary worlds, while we’re working on a more persistent world – just like we have scratchpad memory in a supercomputer, there will be scratchpad Omniverse worlds.
All of these worlds will be powered by, processed by, an AI computing system that is able to process the things that we know it needs – which are visual information, sensor information, physics information, and automated intelligence – and they will be in datacenters. Datacenters will be, for all intents and purposes, alternative universe engines. And you can imagine us building Omniverse servers and Omniverse datacenters.
In fact, the first one that Nvidia is going to build that is that a genuinely dedicated to Omniverse worlds is Earth-2, which is going to be a very large supercomputer designed with the primary purpose of simulating climate. It will be our contribution to helping predict the future of climate change so we have a better system to test our strategies for mitigation and early warning systems for adaptation. Given this, we want it to be as regional as possible. As you know, one and a half degrees in average temperature rise is very alarming, but we don’t experience average temperature. We experience hurricanes and tornadoes and violent storms and floods; we experience the Mekong going from one of the largest freshwater ecosystems in the world to becoming mostly saltwater. So what we want to do is create a simulation engine for the planet that is continuously running.
You can imagine, of course, a whole bunch of these Omniverse engines that are simulating alternative universes of all kinds. That’s why it’s so interesting and so exciting. I think that the exciting part of it for me, is that it has tangible and known powerful capabilities to help us solve problems. And the reason what we know that so innately is because we simulate everything in our company. We simulate our architectures of chips, all of our computers. And because we simulate it, we could predict the future, we could see the future before it happens.
Timothy Prickett Morgan: You can see where it’s going to break before it does.
Jensen Huang: That’s right. And so you could you imagine if we could apply this to some of the world’s most challenging problems – to be able to see the future, to understand its limits, to understand the impact of the decisions we make today on the planet three decades, four decades, or seven decades from now. The reason why climate change is so hard to mobilize society is because very few of us get urgent to take action on something that won’t happen for 70 years.
Timothy Prickett Morgan: Well, it is clearly happening now, in my lifetime. We have just replaced the roof and the whole chunk of the house after damage from a Nor’easter that hit us two years ago, just before the coronavirus pandemic outbreak. We see it in our growing season and the unpredictability – almost capriciousness – of the weather here in the Appalachian Mountains. We had tornadoes within ten miles of our house this summer. That ain’t normal here in western North Carolina.
Jensen Huang: Some people have internalized this, and I’m happy you do it. But you could also argue that, you know, weather happens. Storms happen. And so you could rationalize it away. But the thing that is going to really make it tangible is for us to be able to simulate the end of the century and visualize it.
Timothy Prickett Morgan: Do you think people can handle that, really process that? I don’t know that a lot of people trying to live day to day can fully process what the future might look like decades from now. Even if you show it to them, they might get tharn or fatalistic.
Jensen Huang: Well, I hope they will process this. And if they believe in the simulation like our company – we believe in the simulations and we dedicate more resources to simulation than just about anybody – then they can believe in taking the actions to either reinforce the results of the simulation or to the fix the outcome of a simulation …
Timothy Prickett Morgan: Three years ago at SC19, I wrote a title for an essay I have never finished: The Future We Believe In Is The One That We Simulate, and I never could figure out how to end it. I’m beginning to get a feel for it, now …
Jensen Huang: That’s exactly right. That’s exactly right.
Timothy Prickett Morgan: If we believe in the simulations, and also simulate the things that we believe in, then, you know, part of me wants to be hopeful that people know how to handle this information. The other part of me says it shuts people up and shuts people down. I don’t want to get into a deep philosophical conversation – well, I actually do, but not here and now – but I am hopeful, like you, and I am also pessimistic a little bit. Technology usually cuts both ways, which you know as well as I do.
Jensen Huang: Well, I do believe that most people – not everyone, but most people – if they understood the consequences of their actions, they would try to do something about it, and that the real problem is just that we can’t visualize, we can’t imagine, we can’t experience in any way the consequences of our actions so far into the future.
Timothy Prickett Morgan: Well, simulation is a great way to show us, then.
Jensen Huang: That’s what Earth-2 is all about. Earth-2 is probably the most tangible, powerful benefit of digital twins. You could apply that same concept to factories, you could apply that same concept to a fleet of autonomous vehicles connected together in a virtual world that we call Omniverse, and there are digital twins of the robots, and you could imagine designing the factory and designing the robots, then teaching the robots how to be good robots and as you operate them, you improve them. You optimize them all in these virtual worlds and then bring them into the real world. That’s what Omniverse is about.
Timothy Prickett Morgan: Let me circle back on the Nvidia datacenter business. How does this keep the business growing at the current rate? Does it accelerate it further?
Jensen Huang: It absolutely accelerates it.
Our vision of the future of datacenters is that a datacenter is a computing engine that processes applications that are gigantic in scale but has combinations of everything from physics simulation to artificial intelligence to computer graphics. And when you think about that datacenter, and who would be best at it building it, that would be us. And, and it is also exactly the reason why GPUs are really quite the perfect engines for this Omniverse world because you have to do physics, you have to do AI, you have to do computer graphics – and you have to do it at extremely large scales.
With the problems that we are solving today, everything from fractionalizing the computer so that we can scale it out to being able to scale it up to do some problems – you simply can’t break down some problems so you have to scale up – to having millions of people inside that cloud native environment that is still a supercomputer. We are trying to solve all these problems that lead to this future world. Some people think it is interesting that Nvidia wanted to turn a supercomputer into a cloud native system. For what reason? Well, you have to share Omniverses with millions of people. This isn’t just the place that you go to by yourself. And why are we working on AR and VR – Nvidia doesn’t make displays. Well, I want to make it possible for you to tunnel into and out of Omniverses. AR is how the AI comes out of the Omniverse into our world, and VR is a wormhole that we use to go into the Omniverse.
Timothy Prickett Morgan: That’s funny. You are coming full circle. You’ve turned the world into a video game of sorts.
Jensen Huang: Yeah, of sorts. There are some fundamental differences …
Timothy Prickett Morgan: You look perplexed. I didn’t mean that as an insult …
Jensen Huang: No, no, I didn’t take it as an insult. I just wanted to think for a second and I know you enjoy the technical background a bit. So let me explain.
There’s a fundamental difference between Omniverse and video game engines. And the fundamental difference is that everything is generated in real time in Omniverse. You can’t pre-bake stuff like you can in a video game. For example, if you take a look at a video game, today, you’ll probably notice that the download is like 500 GB, 600 GB, 800 GB now. And the reason for that is because most of the textures and most of the lighting was pre-baked, meaning rendered offline. People are surprised by this. Almost everything in a video game today is rendered offline, like a movie. And then we have a compositing dynamic layer, the lighting layer, that allows us to add the texture in addition to the render because the lighting equation is additive. We can add specular, dynamic lighting to it, and it will make you feel like that entire area is dynamic. But the fact of the matter is, in a video game, the sunlight doesn’t change very quickly. And they constrain the video game environments. For example, when a building collapses it does not expose the outside instantly. And the reason for that is because if they do that, then the ambient component, the global illumination component, of lighting, completely changes. And the games cannot handle that in real time.
In the case of Omniverse, because we literally build everything up from the ground up, and there is no way to pre-compute the light. In Omniverse, literally everything is done in real time. That is an incredible miracle. When you drop a ball, it lands on the ground and bounces off the ground. If it’s a metal ball, or if it’s a rubber ball, because of the physics engines, it does the right thing. Physics is simulating in real time, collision detection is done in real time – nothing inter-penetrates. And all the lighting is done in real time. That’s the insane part of Omniverse.
Timothy Prickett Morgan: That’s an important distinction, and Nvidia is more than happy to sell all of the compute power needed for this.
Jensen Huang: First of all, it’s really about inventing this future and then the business comes second. You never know what people want to buy or not.
We believe that the immediate application of Omniverse is to be able to simulate digital twins for all of the things that you and I just talked about, or to connect 3D worlds together. So let me give you an example. When you are in the Adobe world, that’s a virtual Adobe world, they have their own data set and data structure, people who are designing an Adobe design in Adobe. There are Dessault Systemes worlds and PTC worlds and so on. Just like websites are connected with HTML, if things are connected through some standard, I can share something with you through a common portal, which in this case is based on Omniverse and what we call Universal Scene Description, or USD. And this common portal lets all of us see what’s inside and see our contribution to that world. We could actually be building this virtual world together, see it being built up from the ground up. It’s like Google Docs, but for 3D.
Timothy Prickett Morgan: Well, we’ve seen it in the Iron Man movies, so let’s get on with it.
Jensen Huang: That’s right, exactly. Somebody could be in VR, somebody could be in AR.
Timothy Prickett Morgan: Let’s talk strategy here. Omniverse Enterprise has a list price of $9,000 per seat, and seeing software with a price from Nvidia is not the usual thing. Nvidia does a lot of software development, but I don’t know how much of the company is actually dedicated to software versus hardware.
Jensen Huang: Three quarters of the company is in software.
Timothy Prickett Morgan: OK, that’s big, but that is certainly not how you allocate revenue. You remind me of the classic IBM from the late 1960s, when the mainframe hardware had a price and the software and services came with it. But over time, as Moore’s Law made hardware less expensive and IBM got competition, software and services got prices and capacities went up, but the overall price of the full systems did not. I just wonder if Nvidia will go down this same road.
Jensen Huang: I have never, not even one time, thought that we weren’t being paid for software. Nvidia has always been paid for software. It just so happens that you got it in the device, and that is no different than an Apple iPhone, and look at the richness of the software that comes in that device.
The only question for Nvidia when it comes to software pricing is this: What’s the best way to exchange value with a customer? And that is really a question that is actually very simple: What’s the easiest way for the customers? If you look at the way we distributed our products in the past, our products found the end customers through a layer of distribution because our technology was integrated into a PC or a game console or a server.
When you are in the hardware business, you build the hardware, you sell it, you’re done. And ideally, the customer never calls you again – that’s the definition of good hardware. The definition of the good software is that you keep upgrading it, and you do it for as long as you shall live. At Nvidia, I say, whatever we do here, whatever decisions we make, just remember, you will honor and live with this promise for as long as we shall live. Literally.
Our GeForce customers are so blown away that we continue to upgrade our software, year after year after year. If you look at the CUDA customers that are so blown away on CUDA 12, but they paid for our graphics card years ago. And they are blown away by the fact that we keep upgrading and accelerating software stacks for years and years afterwards.
When you buy a computer from us with all of our technology in it, and magically years later, it gets faster just with software downloads. Clearly, the value that we deliver is largely in software. You bought the hardware and got its initial performance, but you could get 3X, 4X, 10X performance increase in the life of that hardware without ever changing the hardware and all through software updates. So the value of our products is, therefore, obviously largely in software. In every single respect, software is how we see the world, and the strategies that we execute, and the culture we create in the company for 28 years, has been that we are a software company.
Now the other question is: How do we get paid? That’s fundamentally, in my opinion, a completely different thing. And I keep the two concepts – how do I make money and what do I stand for – completely distinct. And so, how do we make money? Well, whatever is fair for the market and whatever is the easiest path to the market. You could argue: How can Nvidia afford to do so much software if it is selling GPUs? Especially when Nvidia is only getting paid a few thousand dollars for a chip. How does that possibly make economic sense? And the answer is it doesn’t – unless you have volume. So instead of slicing the world vertically, we decided that we wanted to slice the world horizontally – because by being a platform company and thinking horizontally, we have the benefit of increasing our installed base slowly, developing software that benefits the market slowly, developing value and the whole stack, and all of the ecosystems that go along with each domains slowly. As you know, the UDA in CUDA, the Unified Device Architecture, was invented in 1995. It is an architecture that was created before many of our current employees had graduated from high school or were even born. We decided that we would rather advance a new computing model, and you can’t do that as a vertical sliver, you have to do it horizontally. Thus, this is our business model.
Timothy Prickett Morgan: How do you square that with the way enterprises want to allocate hardware and software spending? As I said, Omniverse Enterprise has a support contract and a license fee.
Jensen Huang: In the case of enterprise, the customer wants something very different now, and this is an important point. Enterprises want a promise that says: If we need you to live to a particular rhythm of updating, or even fork off to fix one of their specific problems, or to provide backwards support for a build of software that they have committed to because they have built all of their other software on top of it, then Nvidia needs to stay with that build, even in future generations of architectures that are inconsistent with Nvidia’s natural rhythm.
Timothy Prickett Morgan: People make decisions on platforms for ten years, and then they end up using these platform for two decades, sometimes three decades, sometimes more.
Jensen Huang: That’s right.
Timothy Prickett Morgan: It’s very hard for people to get their brains wrapped around, but I have been around long enough to see this persistence that is not resistance, but investment and safety.
Jensen Huang: And enterprises want to pay you, and they want to enter into a service level agreement that’s very specific, and it captures their rhythm and their way of doing business. And Nvidia will support us in this way even though it’s inconsistent with the natural way that we do our engineering. For enterprise software, you need to have that kind of relationship with customers, and they need to know that when they pick up the phone, you will drop everything because they have a business that is literally built on top of your software. They are not just playing a video game, it’s not an inconvenience to them. And by you providing that level of promise, they can then transfer your promise to their customers.
We started coming down this path of taking what is code that is downloadable from the web, moving fast and innovating as we create it, and making an entire system and codifying on top of enterprise method and enterprise sensibility. And that is how we got the Nvidia AI Enterprise stack. And now we have maintaining, scheduling, and innovating on that stack, providing backwards compatibility of it, and also how we fork it – all enterprise grade.
Timothy Prickett Morgan: And now there is a two-year cadence in place for GPUs, DPUs, and soon CPUs as well that enterprises can count on.
Obviously, AMD is much more competitive with its “Aldebaran” Instinct MI200 series GPU accelerators than it has ever been. It is really two GPUs, not one, and I reminded everyone that AMD had “pulled a K80” by putting two GPUs on one device, but nonetheless, this GPU has won two exascale-class systems and many more smaller systems.
I realize that there will not be new GPU announcements from Nvidia until next year, based on the cadence, but what is your response to this competition from AMD, and soon, to a lesser extent, from Intel in the GPU compute arena?
Jensen Huang: First of all, we have competition all the time. So it is not true that this is the first so-called Nvidia killer that has come out. Every year there’s an Nvidia killer and people call it that.
Timothy Prickett Morgan: I mean in the upper echelon HPC and AI supercomputer space. For the past decade and a half, when it comes to GPU-accelerated supercomputers, you have been the whole game.
Jensen Huang: Actually, I think this is the absolutely easiest space, and let me tell you why. The reason for that is because an HPL machine needs two things – just two things. HPC centers order everything years in advance, so they have no idea what performance will be for any given device, but here’s the equation for you …
Timothy Prickett Morgan: Go ahead …
Jensen Huang: The number of peak FP64 flops and memory capacity, put those two things put into one bucket. And in the other bucket, put in dollars. That’s it. That’s the equation. And you know that …
Timothy Prickett Morgan: And so AMD decided to jack up the flops and slash the price? That’s what I think happened …
Jensen Huang: The question is how do we see the world, and the reason why competition is so intense for us. And it’s seriously intense. It’s not minor intense. It’s seriously intense. Accelerated computing is not for the faint of heart. So let me just prove it.
You can build the world’s best fricking everything-anything chip, you stick it into the computer, what will you accelerate? Absolutely nothing. Isn’t that right? Accelerated computing is insanely hard. And the reason for that is Moore’s law is insanely good. No one has ever looked at Moore’s Law, even at its reduced rate and said over the course of time, that is not one of the most formidable technology forces in the history of humankind. And yet, in order for us to succeed as a company, we have to deliver results well above Moore’s law.
Timothy Prickett Morgan: Google told me many years ago how they have to beat Moore’s Law every day to stay in business …
Jensen Huang: By enormous amounts. How do you do that? There is no compiler to help you do that. Somebody has to go and refactor the entire stack. And so we have to first understand the application, its algorithms within it, come up with new libraries, come up with new systems. And when you’re done, you get one application. That’s it. The world has tens of thousands of applications, right? It’s just unbelievable. And you might say with deep learning, that one algorithm, we finally got it. Now everything can be faster. But it’s completely wrong. Computer vision has a completely different architecture. Every architecture is different. Every algorithm is different. And you have to understand the application, refactor the entire stack, one at a time. But you have to work with developers, you have to convince them to accelerate their application. It is just so hard.
And that’s the reason why, in the history of time, there’s never been another computing architecture aside from CPUs until now, with accelerated computing. And the reason for that is all the reasons that I said. It’s just insanely hard to refactor the entire stack on the one hand, not to mention convincing the person who owns the application to do it in the first place. They will just wait for Moore’s Law. It’s so much easier just to buy a few more nodes.
To do better than that, you have to go full stack, you have to go domain by domain, and you are going to have to develop a lot of software. You are going to be working on a lot of solvers, hacking away at it like we are. And then of course, after almost 25 years, the architecture becomes trusted everywhere. And so this is where we feel quite privileged. But nonetheless, the ultimate competitor is doing nothing and waiting for Moore’s Law. We are a $10 billion datacenter business, which is maybe five percent of datacenters. That’s another way of saying that 95 percent of datacenters are all CPUs. And that’s the competition.
In this new world of computing, most of the hard problems that you want to solve are not are not 50 percent away or 2X away. It’s a million times away. For the very first time in history, the confluence of three or four things, you know, have come together that makes it possible for us to go after the 1,000,000X. The climate science community will tell you that at a reasonable scale to succeed, we are probably somewhere between 100 million times or a billion times of computing away from solving the problems. Because every time you increase the resolution by half, the amount of computation explodes – because it’s volumetric. And the amount of physics that comes into the domain of simulation explodes. We are talking about 1,000,000,000X computing problems.
But the thing that’s really quite amazing is that GPUs led to the democratization of deep learning, which led to physics-informed neural networks. Can you imagine a neural network that obeys the laws of physics? It learns physics from principle equations and by observing nature, but whenever it predicts, the loss function is governed by the laws of physics, so it doesn’t leave the boundaries up. It obeys the laws of conservation of energy …
Timothy Prickett Morgan: Maybe we already live in a universe just like that …
Jensen Huang: I know, I know. So here’s the amazing thing. If I can get a neural network to obey the laws of physics, I know one thing about neural networks that I can do very well, which is I can scale it up incredibly. This algorithm, we know how to scale it. So GPUs made it possible for us to do physics-informed neural networks, which allows us to then scale it back up with GPUs. GPU acceleration gave us 20X to 50X on physics. Now, all of a sudden, this neural network, because it’s multi physics, all of the partial differential equations are all learned away. All you have left now is neural networks, and we have seen examples 10,000X to 100,000X faster – and I could keep it synchronized with my observed data that I’m getting every day from the real world. And then on top of that, because I can parallelize it, I can now distribute it across 10,000 or 20,000 GPUs and get somewhere between 100,000,000X to 1,000,000,000X. That’s why I’m going to go build Omniverse. The time has come where we could go create these incredible digital simulations of the world. We are going to give our ourselves a leap and this will change computer science. I am completely convinced this is going to change scientific computing altogether. This is really about how computing has fundamentally changed because the computer science changed the algorithms and now the algorithms are coming came back to change the computer science.
Related Interviews:
One On One With Jensen Huang: Nvidia, The Platform Company (video)
Nvidia Plus Mellanox: Talking Datacenter With Jensen Huang
Can’t wait for the Omniverse. All this open flow of people and ideas has worked really on the Internet. I can only imagine how much, erm, more horrifyingly bad it will be in virtual reality.
Wow! Just doesn’t cover it! What a great interview, literally mind blowing!
Mr. Huang explains, very timely, “This is how I look at Nvidia from a revenue perspective on ever evolving business transition; components, systems, licensing, platform as a service, software as a service. The money is too the right where Nvidia in relation component revenue today gives a lot of software away.” I stand corrected on giving software away? Mike Bruzzone, Camp Marketing
Nov 16, 2021. 08:54 PMLink
Nvidia: We Have A Problem – Business Quant
At its hard core, observing the evolving deployments of a software company, Nvidia has achieved the physical status of mass attractor. Mike Bruzzone, Camp Marketing
It is mind blowing, if he delivers what he is saying, ie get 1BX acceleration by paralleling 10k-20k GPU; Nvidia is doing what Wintel was about to do 30 years ago, and its stock price definitely reflects that.
One thing I can apply the omniverse to is car crashes. I emailed Tesla last year when I was on a bus going down Highway 1 next to the cliffs and said “it’d be great if this bus I’m on had some sort of AI that could prevent it from going over the cliff if the driver, God forbid, passed out right now, in that case instead of driving right off the AI took over and gently pushed to the brakes until the bus stopped”