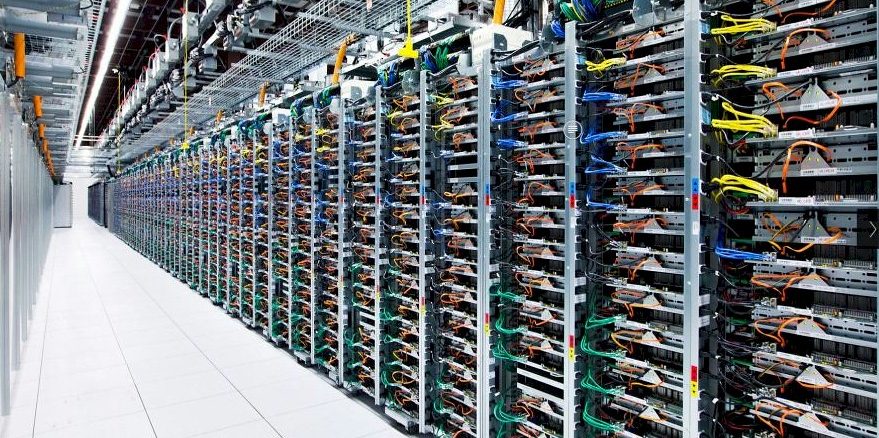
To Gerrit Kazmaier, the distinction between managed databases and data lakes has never made much sense, and it makes even less sense today as data is piling up like soaring mountains being pushed up by tectonic forces.
“That distinction was never useful,” Kazmaier, general manager of databases, data analytics, and Looker at Google Cloud, said this week at a virtual meeting with journalists and analysts. “It was a technical necessity because the data volumes have just kept growing and it was becoming too complicated and cost-prohibitive to manage them in traditional data storage technologies.”
As the amount of data increased, organizations turned to data warehouses. As the volumes expanded even more – and with higher percentages of unstructured data – they started bringing in data lakes to completement their data warehouses.
“That created an obsessive need to store high amounts of data in different warehouses at scale at a relatively low cost,” Kazmaier said. “This was the entry of the data lake movement. But it came at a big price. It came at a big price of consistency, security and manageability for all of these organizations who tried to innovate on top of the data but found it to be at the end of the day, just a data swamp.”
It also created separate silos of data within the IT environment that enterprises had to manage, a headache that other vendors, from Hewlett Packard Enterprise and Dell Technologies to Pure Storage and Hitachi Vantara, are trying to solve. Earlier this year we wrote about a startup called Onehouse that emerged from stealth with a plan to leverage the open-source Hudi to bring database and data warehouse functionalities to data lakes, creating lakehouses that can house and manage structured, semi-structured, and unstructured data.
Google Cloud is looking to do something similar. At its Data Cloud Summit this week, the company is unveiled BigLake, unifying data warehouses and data lakes to enable organizations to store, manage and analyze their data via a single copy of data without having to duplicate or move it or to worry about the underlying storage format or system.
BigLake extends Google Cloud’s BigQuery data warehouse functions to data lakes on Google Cloud Storage, uses an API interface for greater access control on Google Cloud and open formats, such as Parquet, and open source processing engines, like Apache Spark. It eliminates what Kazmaier called the “artificial separation between managed warehouses and data lakes.”
BigLake, which is available in preview, is one of a number of new offerings and enhancements that Google Cloud is rolling out at the event that leverage the work the organization has done over the years with such data tools as BigQuery, Vertex AI – a collection of services for enabling enterprises to build and manage machine learning workloads – the Spanner distributed SQL database management and storage service and the Looker business intelligence platform.
All that along with new offerings like the Database Migration Program and updates in its partnership programs are aimed at enabling enterprise to more easily derive greater business value from the mountains of data they are creating. Google Cloud is the world’s third largest cloud provider with about 10 percent of global revenues, lagging behind Amazon Web Services (with about 33 percent) and Microsoft Azure (about 22 percent).
Addressing data challenges – not only storing and managing it, but also moving, processing, analyzing and securing it – can help Google Cloud continue to accelerate its multi-year effort to gain a greater presence in the enterprise. Market research firm Statista is forecasting that more than 180 zettabytes of data will be created in 2025.
“Data is pretty much on top of the agenda of every C-suite on this planet,” Kazmaier said. “We believe that to transform, you actually cannot apply outdated technologies, outdated architectures and outdated ideas to unlock the unlimited potential data truly holds. … Data today is multi-format, it is streaming and at rest, it is across datacenters and even across clouds today. A data architecture needs to bring all of that together.”
Google Cloud was able to build what it had already done in the data storage area with services like BigQuery to build out BigLake.
“We have tens of thousands of customers on BigQuery and we have invested a lot in all the governance, security, and all of the core capabilities,” he said. “We’re taking that innovation from BigQuery and now extending it to all the data that sits in different formats, as well as in lake environments, whether it’s on Google Cloud with the Google Cloud storage, whether it’s on AWS or it’s on Azure. We take the innovations and expand them out onto other data lake environments.”
Along with BigLake, Google Cloud will soon enable data engineers to track changes within their Spanner database in real-time. Spanner change streams, which is coming in the near future, tracks inserts, updates and deletes across the database. The changes can be replicated to BigQuery to drive analytics and stored in Google Cloud Storage for compliance.
Vertex AI Workbench, which is available now, creates a single interface for data and machine learning systems, giving users a common toolset for data analytics, data science and machine learning and for accessing BigQuery directly. Workbench also integrates with Serverless Spark and Dataproc and enables organizations to build, train and deploy machine learning models five times faster than traditional systems, said June Yang, vice president of cloud AI and analytics services on Google Cloud.
In addition, Google Cloud has Vertex AI Model Registry, a service in preview that makes it easier for data scientists to share models and for developers to more quickly turn data into predictions.
Connected Sheets and Data Studio for Looker are part of a process at Google Cloud of pulling more closely together its business intelligence services portfolio.
“We are bringing these two worlds together,” said Sudhir Hasbe, director of product management at Google Cloud. “Now you can use the self-service power of tools like Data Studio or Tableau and use the central model of Looker semantic layer, where you can define your metrics in one single place and all the self-service tools will seamlessly work and engage with that. This will enable organizations and power users to have self-service tools, but also centralize metrics and have a common understanding of the business across the organization.”
Be the first to comment