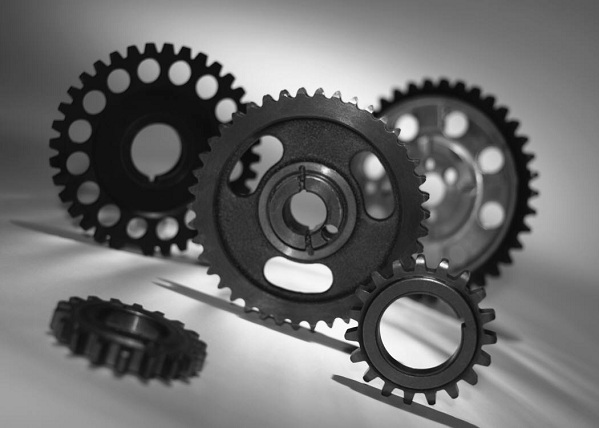
There is increasing pressure in such fields as manufacturing, energy and transportation to adopt AI and machine learning to help improve efficiencies in operations, optimize workflows, enhance business decisions through analytics and reduce costs in logistics.
We have talked about how industries like telecommunications and transportation are looking at recurrent neural networks for helping to better forecast resource demand in supply chains. However, adopting AI and machine learning comes with its share of challenges. Companies whose datacenters are crowded with traditional systems powered by CPUs now have to consider buying and bringing in GPU-based hardware that is better situated to handle machine learning inference work, and they have to find new employees in a relatively shallow pool of available AI talent.
None of this is easy, but the trend is irreversibly toward AI, machine learning and deep learning, so decisions need to be made, according to Karim Beguir.
“When you are speaking large industrial operators, it’s very important to be able to listen to them, to understand their needs,” Beguir, co-founder and CEO of startup InstaDeep, told The Next Platform. “They already have significant investments in the technology they have, particularly around data, and that’s a legacy of many big data projects. … All the signals are pointing that this is the future and that any significant industrial operator cannot ignore the recent progress in machine learning and AI, which is accelerating quickly.”
InstaDeep leverages the DGX-1 AI appliance from Nvidia and collaboration with Google to help enterprises develop customized applications that incorporate such technologies as predictive analytics, computer vision, deep learning and augmented and virtual reality. The company’s customers include those from such industrial fields as energy, mobility and logistics, giving InstaDeep first-hand knowledge of the hardware and software challenges they face when considering AI and machine learning.
“In general, the types of technologies we’re talking about, which are actually machine learning, run on a different type of hardware – GPU-type hardware,” he said. “For example, Nvidia has been one of the promoters of this type of hardware solutions. We’re also seeing other competitors entering the arena, such as Google with their TPU, which is optimized hardware for machine learning. Intel as well is competing in that sector, so from the hardware side, you have new players that are pushing hardware that is adapted to machine learning. So on existing sites for customers in logistics, there is definitely an interest in machine learning-based, artificial intelligence-based solutions, but it is still at the level of curiosity.”
As Beguir points out, this is because this is really an emerging field, so we are relatively early in the process of developing applications. AI and machine learning have been a lot more visible and prominent in visual applications such as recognizing objects and pictures. It is now more visible in such technologies as self-driving cars, but in the area of logistics, it’s still a relatively new thing. However, we’re seeing some very smart players [in logistics] being curious about these types of technology and what they can do, even though these are the early days still.”
For many companies, machine learning can help speed up heuristic functions – the ability to sort through large amounts of data to find the best option to solve a particular problem – through automation. Humans are being many of the heuristic algorithms created in various verticals, Beguir said. With machine learning, people can build the systems, and then the systems can develop the algorithms.
“The current state-of-the-art algorithms have been designed by human experts with a lot of experience in operations research that have developed heuristics to provide solutions in terms of algorithms,” he said. “Heuristics are needed because this is a class of problems that are called ‘empty heart’ problems, meaning you would need tremendous amounts of time to explore all possible paths, and in certain cases it’s absolutely impossible to explore all possible paths due to a combination of really explosive amounts of solutions. You need heuristics to be able to explore the domain space in decent time and come back with acceptable solutions, which are always approximations. The promise of machine learning is to go build that and have systems build the heuristics themselves.”
Google’s TensorFlow platform, which is particularly good at such tasks as pattern recognition, can play a role in the process, the CEO said.
“TensorFlow is very good at pattern recognition, so TensorFlow in a sense is a very good way to deploy a technology called deep learning, which is a subset of machine learning, which is the one about pattern recognition,” he said. “How that relates to what we’re talking about is previous types algorithms have had heuristics that are designed by human experts, so in a sense, the people that are doing things in pattern recognition are human experts. That means taking a problem such as the traveling salesman problem – a classic problem of mobility – a human expert would look at possible solutions and build in intuition on what could be done on the algorithmic side to offer solutions in a reasonable time. That process of having the human experts build up that heuristics and then test it and develop it into codes is being disrupted by having the system now building their own heuristics, which are specific to the problem at hand. It’s really a conceptual revolution in the way logistics and operations are going to operate because it is essentially the machine figuring out the patterns and then building a good algorithm for finding out the solutions.”
A challenge for many industrial companies is finding the talent with experience in such technologies as Nvidia’s CUDA platform for GPU-based computing and TensorFlow. How prepared they are for AI and machine learning varies, which is why companies like InstaDeep are cropping up to provide the expertise to organizations that might otherwise have trouble finding it.
“This is a new field, so what we are seeing from the larger industrial customers to whom we speak is definitely some interest in looking into the sector,” Beguir said. “In terms of preparedness, I would say we’re still in the early stages so people are still really looking what’s happening, particularly in the areas of the GPU space. People are becoming increasingly aware but not necessarily deploying them in practice yet. … There is a relative lack of familiarity about how to do this process and it is good to talk to experts in that field and it is a role to actually help our industrial customers make that transition, explain to them the potential there, but also make it a reality through practical projects and deployments of these types of technologies. … On the talent side it is an open question [about whether companies should] hire people to do these kinds of things. There is a lot of demand for AI talent and it is very hard for industrial operators to build those kinds of teams internally.”
As with any emerging technology, there also is the question of ROI. When IT professionals approach their business executives with the idea of embracing AI, machine learning or similar new technologies for logistics, the question eventually comes down to cost and the return on investment in such areas as hardware, software and talent.
“What is very clear in what we see and also experience in other sectors is that, yes, it does make … sense to adopt these types of technologies because what we are seeing on the machine learning side is that it has set new standards in pretty much any in any industry in which there has been serious development,” he said, pointing to the fast pace of innovation in the autonomous vehicle space. “But it is not a particular industry. It is fundamentally in the nature of these technologies that you would get state-of-the-art results if you have enough data and enough development of the hardware and on the algorithmic side. In a sense, it is the way history is pointing and this is what’s been happening in other sectors, so I can confidently say that the same scenario will play in the field of logistics and optimization.”
I have been in the logistics and supply-chain industry field for years – and recently got onboard Deep Learning and Machine Learning. I feel that the industry has yet to adopt these new technologies beyond forecasting – so glad this article came out. At the same time, I was hoping there were more examples of how these technologies are being used – the article is too general as it stands now. Hope to see more in the future.
Typo: “empty heart” ==> “NP-hard” (https://en.wikipedia.org/wiki/NP-hardness)