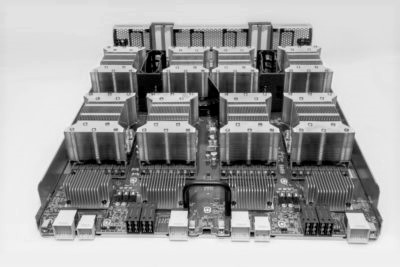
At well over $150,000 per appliance, the Volta GPU based DGX appliances from Nvidia, which take aim at deep learning with framework integration and 8 Volta-accelerated nodes linked with NVlink, is set to appeal to the most bleeding edge of machine learning shops.
Nvidia has built its own clusters by stringing several of these together, just as researchers at Tokyo Tech have done with the Pascal generation systems. But one of the first commercial customers for the Volta based boxes is the Center for Clinical Data Science, which is part of the first wave of hospitals set to use deep learning for MR and CT image analysis.
The center, which is based in Cambridge, Massachusetts, has secured a whopping four DGX-1 Volta appliances, which sport the latest GPUs with eight per node with the NVlink interconnect. The Next Platform talked with Neil Tenenholtz, senior data scientist at the center, about where deep learning will yield results for hospitals and medical research and about their early experiences with the four machines.
Ultimately, the advanced models based on TensorFlow that are trained on the DGX machines could do much of the work of radiologists. Prior to trained models like those teams at the center are working with, there were 2D slices that could be compared and analyzed individually. Using a multimodal approach with 3D images with different features selected for these models, the output of the models is closer to the big picture a radiologist needs to arrive at a diagnosis.
“With a lot of these modalities, like MR for instance, there are multiple pulse sequences that can be applied. So when a radiologist looks, it’s not a single element they are looking it, it is all the pulses that can highlight different features like fluids or solids. So instead of looking at two-dimensional data, it is more like four dimensional. That amount of data grows dramatically though,” Tenenholtz says, and while the datasets are not on the order of something like ImageNet, they do require a powerful system that has the compute, memory, and data movement balance to train at scale.
Soon, Boston-area radiologists will have AI “assistants” integrated into their daily workflows, helping them more quickly and accurately diagnose disease from MRIs, CAT scans, X-rays and more. The trained neural networks residing on DGX-1 systems in CCDS’s data center are in a constant state of learning, continually ingesting countless medical images worldwide. Because trained neural networks can provide superhuman pixel-by-pixel image evaluation and analyze scores of other data with incredible speed, doctors can make more accurate diagnoses and treatment plans.
The center’s DGX appliances are just being used for image processing now but there are projects on the horizon for including natural language processing and text-based models within the hospital. The center is also lending out their DGX appliances for partner centers that are just getting their feet wet with deep learning in a clinical setting. Prior to getting the DGX appliances, Tenenholtz says teams had smaller clusters with GPUs but running most of their workloads for image processing on a single GPU was too memory-limited to be useful. The shared memory of the DGX (as we describe here architecturally in its initial Pascal GPU incarnation) makes this a huge improvement, he says—one that open doors in a big way for hospitals and medical researchers.
Even though deep learning is getting a great deal of traction in medical research, particularly in medical image analysis, the academic work is not quite bleeding over into the real world just yet. Tenenholtz says there are still many hospitals that are new to using GPUs and with deep learning still in its beginning stages, it could take a while yet. Even still, he says hospitals are trying to optimize everything about their operation—from a center and patient perspective. And from a data science perspective, he sees a broad set of deep learning applications on the horizon for hospitals in the coming years.
The next step for his team at the center is to keep refining the TensorFlow based work on the DGX appliances (these are not networked together, they run individual workloads for now) and begin integrating more disparate data types. “Right now we are just doing analysis with imaging data, but we are heading toward integrating electronic health records and other information into very heterogeneous models. This means we need a lot of compute and a lot of memory, but this is where the industry is moving. It is moving along with us in terms of larger clusters with more memory and faster interconnects.”
Forgive my density. The hospital got the first four machines and it is news. Just how good (or bad) are the yields on Volta? This product was announced one or two quarters ago!
Four DGX-1 Voltas is enough to do serious personalized medicine, if they get the software in place.
Hopefully they have a well secured network if those machines are going to have medical records or other patient info that could be tampered with in such a way that it affects the outcome of treatment. Hospitals seem to be notoriously bad at security.