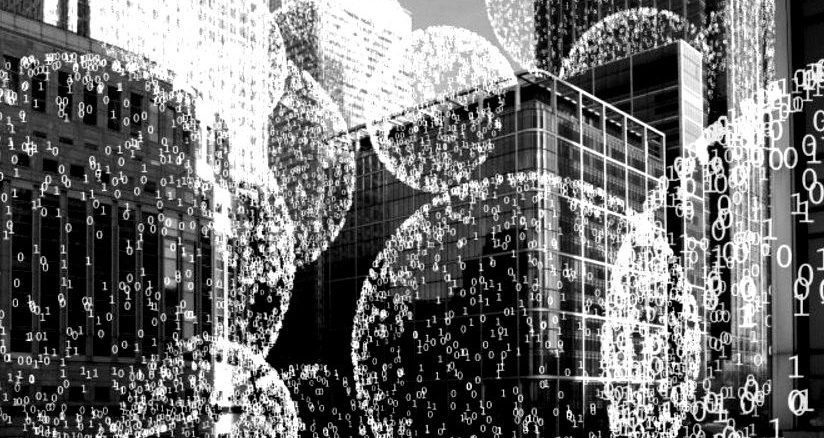
It is a renaissance for companies that sell GPU-dense systems and low-power clusters that are right for handling AI inference workloads, especially as they look to the healthcare market–one that for a while was moving toward increasing compute on medical devices.
The growth of production deep learning in medical imaging and diagnostics has spurred investments in hospitals and research centers, pushing high performance systems for medicine back to the forefront.
We have written quite a bit about some of the emerging use cases for deep learning in medicine with an eye on the systems angle in particular, and while these have been limited to areas like imaging in particular, deep learning use cases are growing. Like other areas in healthcare, deep learning can play a role in the field of pathology, where pathologists try to find the needles of cancer or other diseases in the haystacks that are huge images of samples that need to be scanned and studied.
As the global population continues to age, more cases of cancer are arising – every third person is affected, and in 2012 there were 14 million new cancer patients. By 2030, more than half of the population will be impacted by cancer, so the number of samples that must be screened are growing in both health clinics and research labs. Meanwhile, the number of pathologists is not keeping up with the increase in samples; for example, in China, there currently are about 20,000 pathologists in a country that needs as many as 100,000 given its population size.
Helminen. CEO of Fimmic Oy, a company founded in 2013 and based in Helsinki, Finland, and spun out of the Institute of Molecular Medicine in that city. says his company has a front-row seat to the struggles in pathology, which include not only the growth in samples and the need for more pathologists but also the manual and subjective nature of many of the processes that she said in a recent talk increases the risk of misdiagnoses and incorrect medicine being prescribed.
“There is both the need to improve the efficiency of the workflow and the consistency of analysis in this field,” Helminen said. “The first thing to do to improve the situation is to digitize these samples, and this is an increasing trend in this field today. Digital samples are much easier to archive and retrieve from the archive [and] send to remote consultation, but the most significant improvement is the ability to use machine vision and deep learning [and] artificial intelligence today to help the experts in the analysis.”
Fimmic, which has customers in Europe, North America and the Middle East, three years ago launched WebMicroscope, a web-based microscopy software platform that enables users to store, share and analyze massive digitized microscope samples that include tissues and biopsies. Through the platform, pathologists, medical researchers, drug developers and others can not only analyze and manage the data but also share results with others. In March 2017, the company rolled out the latest generation of its platform that included deep learning and AI capabilities designed to automate many of the complex manual tasks – such as object quantification, tissue classification based on morphology and rare target identification – that used to take hours or days to perform. Some of those tasks now only take minutes.
The WebMicroscope platform not only saves time on these tasks, but with AI and deep learning techniques, can also reduce the number of steps needed in the analysis of the samples. That helps save money, as does the web-based nature the platform, which means firms don’t have to pay for the infrastructure to store the huge images, many of which reach giga-pixel sizes.
With the WebMicroscope platform, the software is sold as a service, with customers having their own dedicated, password-protected accounts. They’re charged based on usage – essentially the more image they store and analyze, the more they pay. For analysis, customers outline what they’re trying to do and send a few slides and images for evaluation. The WebMicroscope Deep Learning AI software is trained to detect and quantify the targets of interest and, after the evaluation results are confirmed, the rest of the images are uploaded for analysis and the customer can manage the images and results through their accounts.
“We’re providing fast and easy access to our customers to these intelligent image analysis tools,” Helminen said. “In the workflow, the customers have the images, they do the scanning on site, and after they have the image, they can upload them to the WebMicroscope cloud. Once the images are there, they can be viewed with any browser, any devices, anywhere they might be with an internet connection.”
The CEO laid out several examples where WebMicroscope armed with deep learning capabilities can more quickly and accurately get results from samples, and how customers can then display the results. In the area of quantification, Helminen showed an example involving testicular cancer and the quantification of a tumor infiltrating lymphocytes.
“This relates to a very hot topic in the drug discovery and development field related to immune oncology drugs and the idea is to find out with this quantification whether the patient would benefit from the treatment with this type of immune oncology drug,” she said. “What we’ve done here is trained our neural network to learn what an immune cell looks like and then automized this whole process. In this particular application, there can be so many results, about a million immune cells in these areas, so we’re not only talking about original large images, but also the challenge of handling a huge amount of results data.”
“The fact that we can do this from the basic … staining is something unique and something that deep learning can bring to this market,” Helminen said. “We can remove additional steps when we can do this segmentation from the basic staining and this saves time and money in the laboratories.”
The key to all of this is that with deep learning and AI capabilities built into the WebMicroscope platform, “anything the human being can see underneath the microscope we can train neural networks to see,” she said. “The aim is to spread the software very quickly to the scientific network so that everyone has the ability to start using these image analysis tools and not to be restricted with expensive on-premises installations, which can take time to get into the institute.”
Currently Fimmic’s software is sold with a research-only status, though the company will eventually move into the clinical sector, the CEO said. The future of pathology is digital with AI and machine learning.
“Very soon I’m sure we will be able to estimate a prognosis based upon the morphology of the tissue samples and we will be able to someday suggest a treatment based on mining the big data of patients with similar symptoms, similar genetic profiles, similar morphological data, and all of this is for the benefit of the patient as it brings a faster diagnosis and cure,” Helminen said.
WebMicroscope’s approach is one of many emerging use cases that we expect to see more of in the healthcare space. These will drive the purchase of systems that might not be on the radar of hospitals that were trying to minimize their cluster investments versus build them out.
Be the first to comment