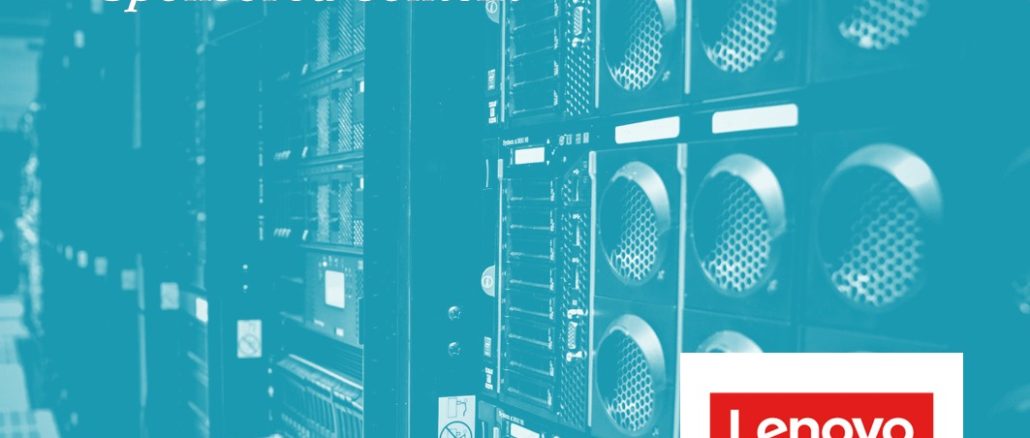
Artificial intelligence is a broad area, covering diverse fields such as image recognition, natural language processing (NLP), and robotics. AI technologies are also developing at what sometimes seems like a frenetic pace, so that it can be difficult to keep up to speed with everything that is happening.
Unsurprisingly, many organizations turn to their IT vendor partners to help them develop and deploy AI solutions to best meet their needs. David Ellison is the senior artificial intelligence data scientist at Lenovo, and his role involves using cutting-edge AI techniques to deliver solutions for customer organizations while internally supporting the overall AI strategy for Lenovo’s worldwide Data Center Group.
Projects that Lenovo has delivered commercially include one to detect manufacturing defects in a factory using convolutional neural networks to extract the features from images and classify them as defects or not. Lenovo has also developed a computer vision system for a racing company to decide whether or not specific cars should be called to the pit for maintenance.
According to Ellison, the major trends in AI for this year and the near future include those applications building on computer vision, the development of data generation and data labelling algorithms for training AI models, and the rapid progress of natural language processing thanks to transformer-based models.
Let’s take a closer look at Lenovo’s overview of some of the major near-term trends in AI.
Computer Vision
In terms of computer vision, developers and researchers are now starting to branch off and look into practical ways of combining this capability with some other area of AI, such as robotics or natural language processing for applications like automated applying captioning of images. This is partly because some of the some of the fundamental problems of computer vision have been proving difficult to solve, and researchers are seeking new approaches to tackling them on top of simply moving the field of research forwards according to Ellison.
“I think we’re running into problems solving some of the core computer vision tasks like 3D projection,” Ellison tells The Next Platform. “When you see a picture, a 2D image of a building, for example, the human mind can extrapolate that into a 3D shape, but it’s very hard for a machine to do that. There’s been a lot of research in that area, but it’s one of the core problems that really hasn’t been solved, and I think people are getting frustrated and are trying something new, or trying to integrate it with other areas and find better uses.”
This is why robotics is currently one of the most widely researched AI areas, Ellison believes, as it gives people a clearly defined goal to aim for. Examples he cited include enabling an AI to use visual sensing to move safely through the space around it – whether that is a robotic arm in a manufacturing plant or an autonomous vehicle using AI to navigate to its destination.
Training Data
People who are already familiar with AI will know that one of the key factors in successfully training a model is data, and lots of it. In fact, the more data it is trained on, the better it will be at delivering the outcome you seek. According to Ellison, computer vision is currently struggling because the datasets used to train the models do not contain enough variety of samples. For example, the Gibson Database of 3D Spaces, a widely used dataset for training models to navigate indoor spaces, includes 572 full buildings composed of 1,447 floors. While impressive, this is unlikely to be a comprehensive set of what an AI system may meet in the real world.
A similar problem occurs when objects are in different orientations than the one the computer vision model has been trained on. In the ImageNet dataset, widely used for AI training, “everything is like, a chair is just a picture of a chair in the middle of a room face on to the camera. If you turn that chair over on its side, suddenly the computer vision applications don’t recognize a chair,” Ellison said.
That shortcoming was the inspiration for a new dataset called ObjectNet, which shows everyday items in different configurations, such as a chair turned over or upside down. This training set is being used to address some of the shortcomings of computer vision, such as the inability to recognize objects that are in an unusual orientation or that are partially obscured.
But the problem of getting large and diverse enough datasets for AI training persists, and this leads to another major trend that Ellison has identified, that of using AI to help produce the datasets in the first place.
Self-Supervision And Synthetic Data
Building a dataset calls for a lot of manual labelling of data from human operators, and so a growing number of research projects now centre on self-supervision algorithms that can take data that has been gathered and have the computer automatically label the data.
“A prime example of this is a robot with a computer vision application and a proximity sensor. The computer vision is able to see further than the proximity sensor. But as the robot is moving forward, things that appear in the computer vision will eventually appear in the proximity sensor as objects,” Ellison explains. Taking that sensor data and looking back in time to when the object was first seen, it can then be labelled.
Data points that are very unlikely for an AI system to encounter once deployed, and so may not be represented in the training dataset, are another challenge for developers. These edge cases nevertheless need to be taken into consideration if they represent real-life scenarios, to ensure that the AI model deals with them correctly.
“The key example there is self-driving cars. How many times are you going to have in your dataset driving up a mountain in the middle of a snowstorm at dusk? You’re not going to have many of those circumstances in your dataset, and so they have to essentially do simulated data,” Ellison said.
In other words, the edge cases are tackled by using synthetic data that is created using a variety of methods, such as generative models. But this raises the question of how data scientists or developers can be sure that such synthetic data is an accurate representation of what the AI would encounter in real life.
“That is a major issue,” concedes Ellison, but the solution so far has been to just generate more data, and hope that this will fill in those use cases with enough synthetic data. However, approaches such as neural network autoencoders or more sophisticated generative adversarial networks (GANs) are also being used, where one network creates the synthetic data and a second one is used to judge the quality of that candidate data.
“So it’s kind of training on itself whether it thinks that example is realistic enough. You train both neural networks simultaneously, one generating those examples, one judging those examples, and you hopefully end up with something that is much more realistic,” Ellison said.
A good example of where GANs have already been used to generate data is in ‘deepfake’ technology, which has been used to create realistic looking human faces, or to alter a video so the person in it appears to be speaking the words from a separate audio track. This demonstrates the level of sophistication that such models have reached, according to Ellison.
Transformational Natural Language Processing
Meanwhile, one of the success stories in natural language processing over the past couple of years or so has been transformer-based deep learning models, and Ellison believes that these will continue to dominate. This is because they have been developed to be able to recognize dependencies and connections between sentences in speech, whereas the recurrent neural networks (RNNs) used in earlier models are inherently sequential in nature and tend to lose the context of words.
“If you look at a transformer it’s got this masked multi-head attention layer and add and norm layers and a feed forward layer, but it doesn’t have all those feedback mechanisms that RNNs have that really slow down the processing, so they are able to look at a larger area.”
This has an effect on the model’s ability to interpret meaning, according to Ellison, with RNNs having a problem with identifying the same entity in multiple sentences.
“RNNs are pretty good at determining the subject in a sentence like ‘Tim moved the chair’, but if you follow up with ‘he has red hair’, we know that ‘he’ here is still referring to Tim, but an algorithm has a hard time making that leap between those two sentences. Recurrent neural networks have had a problem doing that.”
This architecture has led to transformers playing an important role in many of the recently developed NLP models such as Google’s BERT and OpenAI’s GPT-2, as well as Facebook’s RoBERTa and Microsoft’s MT-DNN, which are showing great promise in NLP tasks such as such as document classification, sentiment analysis, question answering, and sentence similarity.
Good article. Well articulated
Good