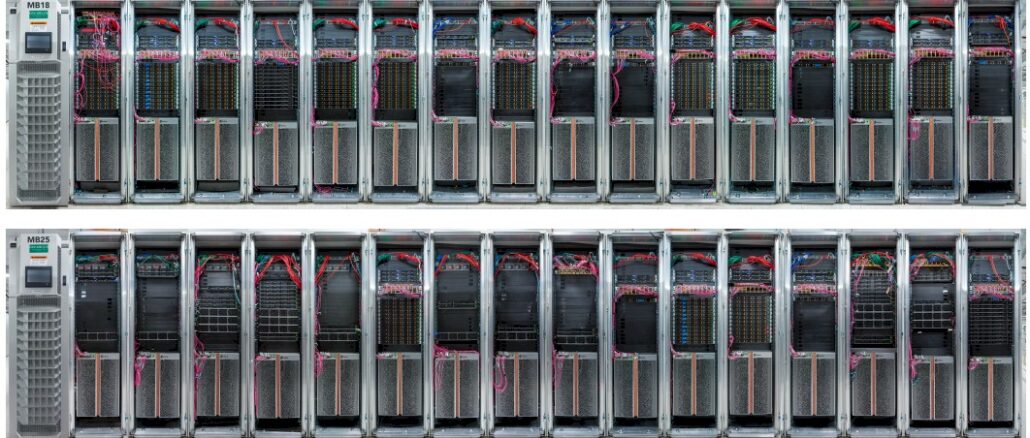
Scientists from KAUST and engineers from Cerebras Systems have fine-tuned an existing algorithm, Tile Low-Rank Matrix-Vector Multiplications (TLR-MVM), to improve the speed and accuracy of seismic data processing.
The collaborative work is up for a Gordon Bell prize to be presented at SC23.
At the core of this is the Cerebras CS-2 system, which is housed in the Condor Galaxy AI supercomputer, that we looked at as just one piece of a broader Cerebras/UAE push in this deep dive from July.
With that in mind, recall Cerebras is making massive investments in its future in oil and gas workloads, in addition to others relevant to the Middle East region. Group 42 (G42), a technology company based in the United Arab Emirates, partnered with Cerebras to develop the Condor Galaxy system, aimed at bolstering regional AI infrastructure.
As noted in July, the first phase involves a $100 million investment to construct the Condor Galaxy-1, a machine equipped with 27 million cores and a processing capacity of 2 exaflops (FP16), alongside the existing Andromeda cluster. Cerebras CEO, Andrew Feldman, outlined a multi-phase plan, culminating in an extensive network of clusters achieving up to 36 exaflops of performance by the second half of 2024.
These systems are worth further discussion but when it comes to the Gordon Bell prize, it’s never just about hardware capability.
The work hinges on a redesigned TLR-MVM algorithm which was mapped onto the CS-2 system’s disaggregated memory system. The algorithm reduces the memory footprint and arithmetic complexity, which gives it an edge in processing seismic applications which traditionally require large memory resources to store intermediate results and matrices.
More specifically, the algo uses ultra low-rank matrix approximation to fit the data-intensive seismic applications onto the memory-limited hardware of the CS-2 system. By recognizing and exploiting the sparsity present in seismic data in the frequency domain, the team managed to implement parallel processing for TLR-MVMs, which are critical components in Multi-Dimensional Deconvolution (MDD) inverse problem-solving.
This “little” adjustment led to memory bandwidth of 92.58 PB/sec, a noteworthy reach across 35,784,000 processing elements in the system.
By optimizing the memory layout and executing batch processes on the ultra parallel, waferscale CS-2 systems, the team managed to maintain application-worthy accuracy using FP32, while also cutting consumption, dropping to 16kW as opposed to the higher power profiles witnessed in comparable workloads on standard X86 hardware.
Cerebras and the KAUST research team are touting this as a solution to the “memory wall” problem, at least for this domain. They also note in the full paper this is also a potential target in areas like geothermal exploration, carbon capture, and storage projects, with greater efficiency and lesser environmental impact.
The KAUST/Cerebras team hope to refine their approach by transitioning from the TLR-MVM kernel to TLR matrix-matrix multiplication (TLR-MMM), a move that is expected to present new opportunities for scaling and further enhancing the efficiency of seismic processing technologies, especially in the analysis of complex geological structures.
Even if the exascale race has slowed a bit, the Gordon Bell Prize awards at SC23 will be anything but a snoozer.
Here in France, with gas at 2 euros per liter (approx. $7/gal) and massive shrinkflation at grocery stores, some folks (this week) suggested popular revolt as a next logical step. The TNP SC23 expert contingent will definitely have to attend this important GBP presentation, press-passes in hands, to ascertain whether supply-side relief could be finally at hand on this (even as we struggle to wean ourselves off of hydrocarbons and develop greener lifestyles and datacenters … which takes a bit of time, and pain it seems)!
In comparison to SCREAM’s parabolic system of PDEs (that involve momentum diffusion, through viscosity), the propagation of seismic waves is more of a hyperbolic process (like ray-tracing, but with way more diffraction and refraction) that is closer to being embarassingly parallel. Its application in an inverse-modeling context, to reconstruct the (heretofore unknown) geological structure of the subsurface, by deconvoluting seismic measurements obtained at the surface, remains, however, a huge computational challenge!
I find the KAUST-Cerebras approach to this to be most impressive! Mapping low-rank MVM to the dataflow architecture sure looks like a match made in heaven!
Figures 15 and 16 of their GBP paper ( downloadable at https://repository.kaust.edu.sa/handle/10754/694388 ) clearly demonstrates the dataflow advantage in this application, with just 16 kW of power consumption!
My immediate (system 1) gut reaction was along similar lines: now that exploiting oil fields already found and known is no longer considered ok in UK, getting awards for better seismic processing seems somewhat tougher than Cerebras getting Emirati money for Arabic AI.
It could be useful to help survey aquifers in drought-stricken Portugal, Spain, and France. Possibly also help perform subsurface characterization of the Moon or Mars. And inverse stereoscopic ray-tracing underlies our visual cortex’s operation (from retina to spatial comprehension) and so its “simulation” using algorithms developed for seismic exploration could help us better understand how our own optical system actually works. Oil is old hat but the tech should apply more broadly I think.