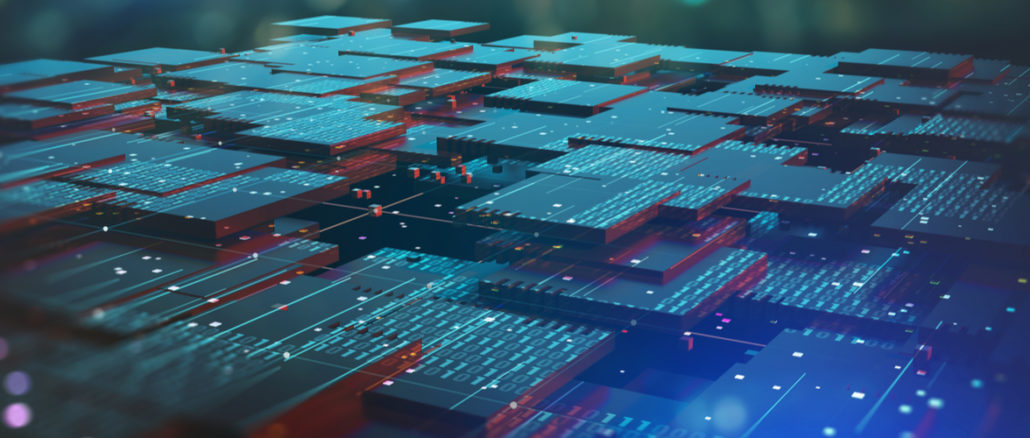
The term “mainstream” in the context of the largest systems on the planet still only means a select few. But it is quite possible that proofs of concept for the sites building those huge future machines could set the stage for what’s next in some of the most demanding compute environments.
After all, what else is on the not-so-distant horizon that looks practical from a general-purpose point of view?
Right now, the options seem to be riding the decline of Moore’s Law or getting on the quantum computing train. The first is inevitable. The second is far from general purpose, especially for the largest government labs with decades-long code bases for nuclear and other mission-critical simulations that are tailored to traditional computing. But there is actually a middle route. While it has its challenges and its own scaling limitations, it could stretch supercomputing performance gains out a while longer while the world (ostensibly) gets ready for practical quantum or whatever is truly next.
Here’s why this middle option, an ultra-heterogeneous, customizable, waferscale platform, makes sense, and also why it didn’t until relatively recently, both from a technical and a market perspective:
Market-wise, the world owes much to AI systems startup, Cerebras, for not only reintroducing the concept of waferscale but also proving they can work. The concept isn’t new, it was a hot topic as far back as the 1980s with re-emergence in the 90s. Failures were too common among so many transistors (for the time) and finding them at runtime was useless frustration. It just didn’t work.
That market rationale only goes so far. Cerebras is focused on AI training and inference with the option to do some general-purpose work as we talked about here. But the compute is power in numbers, SRAM is small and shared between cores. It’s perfect for AI but not so much the HPC shops that can afford to invest in, experiment with, and build around new waferscale. And that’s where the technical readiness comes in.
Cerebras has proven it can be done and that there are a few customers willing to take a look (mostly national labs) but they haven’t shown that the biggest bottleneck with all big compute (the interconnect) can be the key to unlock mainstream potential for waferscale (with “mainstream” defined as general purpose at the high end for now). On the other end, AMD is proving the chiplet approach is the most promising path to continued scaling. And at other ends, Intel, IBM, and AMD as well are showing what’s possible at the interconnect level.
So why not put those things together and build a waferscale interconnect that can leverage chiplets for ultra-heterogeneity, creating big systems that cut out the most pressing bottlenecks, add scalability and customizability? Even better? Make that underlying waferscale interconnect open standard so all the many possible chiplets or (dielets) can join the party, processors large and small, DRAM, HBM, all in configurations that can be workload-suited.
Of course, that won’t be cheap. But neither is placing a billion dollar bet on quantum computing just to have something to look forward to post-Moore.
With major vendors working on various pieces, from EMIB to AMD’s chiplet strategy none of this unattainable. And now, there’s some pioneering work that shows, on the ground, how this can all be practical, from design, reliability, manufacturability standpoints.
In 2015, Saptadeep Pal of UCLA was collaborating with a team on the concept of waferscale. This was before Cerebras emerged to prove to the market it worked. Pal tells The Next Platform a few from his group flew up to San Jose to meet with Andrew Feldman and the Cerebras team but they didn’t exchange technologies, just talked.
“The main idea was to build a system with many cores. Not just compute cores; memory and different types of memory all tightly interconnected and not like systems today but by building the interconnect on the wafer.”
Pal says in the past, waferscale was dismissed because of transistor failures at that scale but the answer was not to build transistors at all, but rather create the interconnect, then take normal dies that can be built and tested separately, grab the known good ones from different sources (processors, DRAM, flash, etc) and pack them onto a waferscale interconnect to capture the best of all worlds. “On a wafer, we already know how to build meta-layers. We could see how to connect these chiplets to the wafer for a true heterogenous wafer and that was our starting point in 2015.”
Highlighting the differences between what Cerebras does versus what Pal and team propose is important to the specific versus mainstream future for such devices.
Physically, Cerebras is building a single wafer with transistors connecting the many thousands of cores. What Pal’s team is doing is taking chips from different sources, putting those on an interconnect wafer, and interconnecting the dies. This leads to the same density, but far more heterogeneity.
“What Cerebras is doing is great for ML with so many cores on a wafer with small SRAM all connected ina mesh network. Every few cores share some SRAM. But we are taking these many different chips, now Arm cores on each chip and DRAM as one experiment of many possible and now we can have a wafer with 100k or 200k cores and terabytes of memory. These memories can be stacked for density and we can see 1TB/s per mm edge on the wafer, for instance.”
The cool thing to think about is what’s possible with mixing and matching. Beefy X86 cores for HPC, accelerators for specific workloads, stacked memory, high density. The options are endless. “And Cerebras really did impressive work by showing how these devices could be powered and cooled.”
Despite Pal and team’s successes and the manufacturing/market/reliability issues addressed by Cerebras, there are still some broad challenges to pushing this into the wider HPC sphere (because it has to start there). The software challenge is its own article, which we’ll save for another date. The sheer number of possible die combinations on such a device creates endless issues, so we’ll spare you that for now. Other than software, just getting these to market on the hardware side is going to take better ecosystems for testing the reliability of chiplets, among other things.
“Testing the chiplets, putting them on the wafer so you can get 99.9% of the to work after bonding? That’s definitely a challenge. But at the same time, because we are chiplet based, we can test separate devices and have learned tricks to get higher confidence beyond the redundancy Cerebras had to build into their architecture.”
He says the real difficult is, once you have that, it’s all very costly and even with a software stack, how do you address this market? “Right now, we are just building off Arm, we haven’t reach into the compiler level stuff. Now is about letting people know whether this one big thing that can offer 100X more performance is going to be reliable.” He adds that people are comfortable with how systems are built now. They can replace elements in their system. What is there to allow taking care of faults on a system like this, especially faults that can affect the overall functionality?
“Systems are getting bigger; HPC is running on hundreds of nodes stretched far apart and interconnect is that bottleneck. We need a consolidated system. Putting it all together on a single piece of silicon that takes care of the interconnect is the answer. Moore’s Law is going to 2nm or 1nm and it’s going to be costly as well. But going to chiplets, as AMD has shown, works. Scaling it out with waferscale has been shown as well. And finding a common software stack, one everyone can be comfortable with today is what we’ll be doing next with X86 and Arm first,” Pal adds.
Rakesh Kumar, a University of Illinois collaborator on the waferscale effort with Pal, adds, “”A chiplet-based approach allows heterogeneous integration of technologies on the wafer. This means that a chiplet-based waferscale processor can have high density memories such as DRAMs, flash, etc., reside on the same processor. This can allow much better memory capacity characteristics than the Cerebras approach that cannot support heterogeneous technologies on the processor, limiting the processor’s memory capacity. This is going to be critical for many applications (including many ML models) whose application requirements far exceed what is provided by the Cerebras processor.
As Kumar explains, “A chiplet-based waferscale processor may also have yield benefits since, unlike the Cerebras approach, a large monolithic die does not need to be manufactured.”
AMD is in a good position to build these. Intel and IBM could do it as well. Cerebras showed a startup can manage to swing it as well but not reach any large market or capture the big DoE deals that will be there for the taking if post-Moore’s capabilities can be shown. There is need, there is capability, there is a manufacturing and market angle.
The time is right and, unlike quantum for areas like supercomputing, the pieces are all there with known mechanisms for getting codes to run. And at the end of the day, that’s what the post-exascale/post-Moore’s HPC realm needs.
HPC is no stranger to advanced packaging. IBM, Cray, and others have been packing components closer together using multi-chip-modules, advanced cooling, and extremely large circuit boards for several decades. This fell apart in the HPC space 10-15 years ago not because the technology wasn’t compelling, but because it wasn’t cost competitive with commodity server processors.
Wafer scale interposers working as an extended wire mat would significantly improve the signal density and total package size compared to current MCM designs. My question is: does it have application outside of the HPC/AI/ML space? If not, is there enough money to support so much divergence in the way we build HPC systems from the way we build other datacenter systems? I notice HPC/AI systems are built with the same processors as AWS server farms. HPC GPUs have diverged some from the GPUs used for video games, but not completely. The system interconnects are sometimes unique, but share the same serdes cores, transceivers, and cables as are used in more common systems. If AMD, or someone similar, were to make use of this packaging technology, would they be able to reuse the same core chiplet designs that they use for regular datacenter processors, and if they do, would they still be able to realize a substantive advantage through this technology?