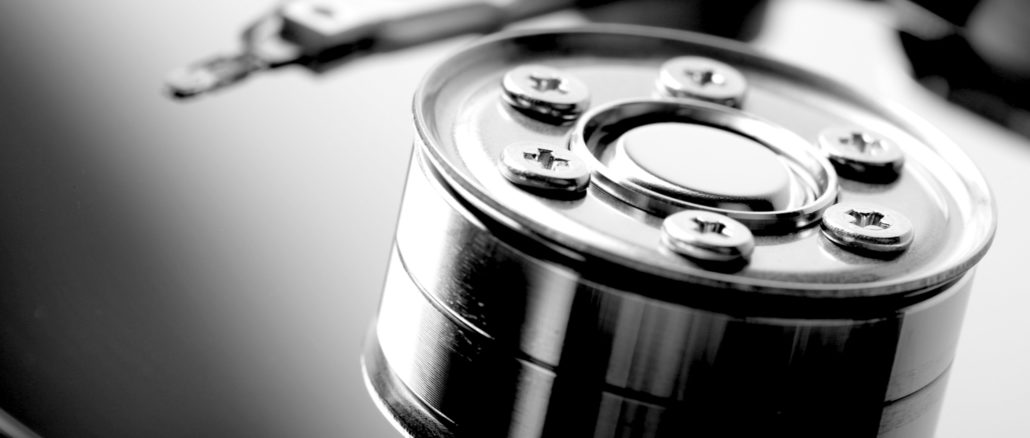
Although artificial intelligence is relatively new to the HPC scene, it can arguably be cast as the quintessential high performance computing workload. That’s not only because this application set is so dependent on computational performance, but also because, like traditional supercomputing, it’s driving system design into uncharted territory.
One area where this has become very apparent is in storage architectures, which have struggled to keep pace with the computational intensity demanded by AI applications. For training neural networks, in particular, the computational demand is insatiable, pushing vendors to build substantially bigger and more powerful systems with every product iteration.
This will be the topic of in-depth live interviews and panel sessions at The Next AI Platform in San Jose next week as we talk to some of the key companies and end users building around bottlenecks and tweaking across the stack for optimal performance and efficiency in training and inference alike.
Just consider that Nvidia’s original DGX-1 appliance introduced in 2016 provided 170 teraflops of deep learning performance. Two years later, the company unveiled the DGX-2, which delivered an unprecedented two petaflops. This second-generation appliance doubled the number of GPUs from 8 to 16, compared to the original, but thanks to the latest deep learning-optimized V100 processor, system performance grew by a factor of 11. Meanwhile DGX storage, based on NVMe gear, increased only by a factor of four, from 7.6 TB to 30 TB.
For systems like DGX-2, including their third-party counterparts derived from Nvidia’s HGX-2 reference design, feeding those GPUs with storage is a lot more challenging than it was for the 2016 system. And if those appliances are scaled out across a datacenter, those challenges become even more acute. That’s why we’re seeing third-party storage solutions for such computationally dense AI platforms, most of which are based on sort of scalable NVMe technology.
One of those that we here at The Next Platform have reported on in some detail is Excelero’s NVMesh offering, which pools NVMe storage so that it can be used as a shared resource across a cluster, regardless what the local storage situation is like on individual nodes. Excelero CEO and co-founder, Lior Gal spoke with us recently about why he believes such designs are critical to the success of AI.
He notes that even when you’ve bought the most expensive servers and accelerators for AI work, you’re only part of the way there. “You still have to feed them,” explains Gal. Essentially, it comes down to two elements: capacity and speed — capacity because smarter, more complex neural networks need bigger datasets to train on, and speed because the utilization of high-throughput GPUs requires big data pipes from storage to server.
For Gal, the capacity element can be addressed by aggregating local storage installed in servers so that it can be shared as a unified resource. The speed element demands fast networks, of course, but also can be helped along by minimizing the amount of data movement so as to eliminate unnecessary copying. Basically, says Gal, you have to think about the storage system at the level of the datacenter, rather than from the perspective of the individual server.
The network element is crucial, and thanks to RDMA-capable technologies like InfiniBand and RDMA over Converged Ethernet (RoCE), you can have both raw speed and low latency. That’s why Gal thinks that you need to rethink the network and storage infrastructure for AI and make sure they’re part of an integrated systems level solution. “When you’re talking about AI it’s really about network storage,” he says.
We will be discussing this and other topics related to the storage and data movement challenges inherent to next-generation AI systems at The Next AI Platform event on May 9 in San Jose. Register now before it’s too late for an-depth view into the entire hardware stack for deep learning; chips/accelerators, storage, networks, systems software, and more.
Be the first to comment