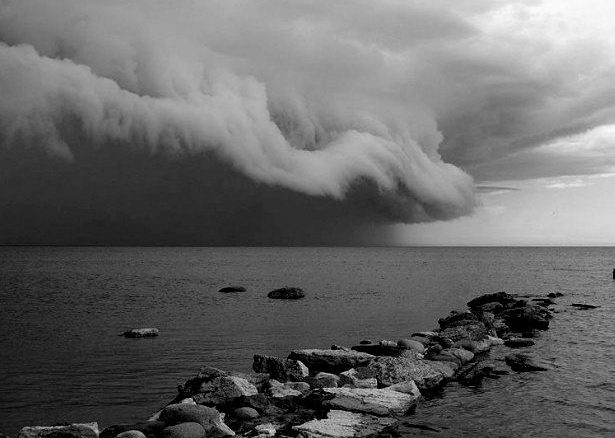
The fields where machine learning and neural networks can have positive impacts seem almost limitless. From healthcare and genomics to pharmaceutical development, oil and gas exploration, retail, smart cities and autonomous vehicles, the ability to rapidly and automatically find patterns in massive amounts of data promises to help solve increasingly complex problems and speed up discoveries that will improve lives, create a heathier world and make businesses more efficient.
Climate science is one of those fields that will see significant benefits from machine learning, and scientists in the field are pushing hard to see how the technology can help them address the challenges presented by climate change. Those efforts were on display at the recent Conference on Artificial and Computational Intelligence and Its Applications to the Environmental Sciences run by the American Meteorological Society, where one of the tracks focused on machine learning and others looked at complementary technologies like big data and cloud computing.
The presentations on machine learning ran the gamut, from using neural networks and machine learning to better predict the hazards presented by straight-line wind events – those that aren’t tornado-related – and better understand low marine clouds to improve predictions around power outages and manage traffic flow through popular outdoor resort areas. It is a broad array of research areas, but it all comes down to similar goals: to use machine learning tools to find patterns in massive amounts of climate data in hopes of saving lives, money and time, and to better address the coming impact of a rapidly changing environment. At the center of it is information. “Data is king,” Christopher Lee Kuhn, an official with Raytheon, said during his presentation on using historical analogs as a training set for a machine learning effort to develop a forecasting tool for geopotential heights.
Thunderstorms in the United States are deadly and destructive, causing more than 100 deaths and $10 billion in damage every year, according to Ryan Lagerquist, a Ph.D student at the University of Oklahoma. Researchers around the country have used machine learning to improve the prediction of such dangers as tornados, hail, and lightning, as well as turbulence in aircraft. However, there haven’t been many that specifically have looked at the dangers presented by straight-line wind, a phenomenon that is more common than a tornado. At the University of Oklahoma, researchers developed a machine-learning system that uses multiple data inputs – radar images, model soundings, and surface wind observations within a storm cell to up to 10 kilometers outside of it – to better predict severe wind (up to 50 knots, or 58 mph) at lead times that range from zero to 15 minutes up to 90 minutes.
Researchers at the Goddard Space Flight Center are using a combination of a machine learning algorithm and crowdsourcing techniques to better classify marine low clouds using satellite images from NASA. According to Tianle Yuan at Goddard, developing a better understanding of these clouds – including how the organize and behave – will improve climate change projections. The algorithm developed at Goddard is being used to leverage what it has learned to better classify the clouds through the use of full NASA images.
Atmospheric Data Solutions (ADS), a company in Orange County, California, is working with San Diego Gas and Electric in the development of a 110-mode system designed to better predict weather-related power outages. The system includes short-range forecast from six to 90 hours and a long-range forecast from one to ten days, leveraging such data as 16 years of historical information on outages from the San Diego area, weather from the Weather Research and Forecasting (WRF) model, and ten-day forecasts of mean sea-level pressure, 850mb wind speed, and 500mb height. In addition, the model includes such tools as 24 distinct Random Forest models to forecast outage predictors and predictands and self-organizing maps (SOMs) to give the power company a more complete picture of where power outages are most likely and what the most likely causes are, according to ADS founder Scott Capps. For example, in one region in the San Diego area, the system was able to pin down where outages are most likely to happen and why – in this case, blame the annual Santa Ana winds.
Another project is using machine-learning techniques in a project that is looking at what’s happening in the English Channel to forecast the two-dimensional parameters of the ocean as the Earth warms. It leverages data from satellite images, and comprises a convolutional neural network to useful information from the data, a recurrent neural network for feature predicting and another convolutional neural network to reconstruct images from the predicted feature of the previous block. According to Julien Brajard, a researcher at LOCEAN/IPSL in France, early results have shown the model to be better at predicting the mean situation and extreme events, such as storms, than more traditional approaches.
Such projects are examples of where researchers are using machine learning to better understand a climate that continues to change rapidly and will have near-term and long-term impacts on life on the planet. Climate scientists are in a position to give decision-makers the information they need to make better decisions on how to react to the impacts of climate change – such as more rainfall, higher temperatures and rising sea levels – and are getting the tools to more quickly and accurately develop that information, according to Cynthia Rosenzweig, a climatologist at the NASA Goddard Institute for Space Studies.
In her keynote, Rosenzweig focused primarily on urban areas, where the impacts of climate change are playing out in dramatic fashion and where there is a lot of activity in addressing the dangers of what she called the “stresses” associated with climate change. For what she called “urban heat,” cities like New York are painting the roofs of buildings white and green. Others are using porous pavement and network basins to help deal with the runoff from more powerful rainfalls, tidal basins and dikes to address rising sea levels and other tools for addressing poor air quality.
“What we as scientists need to do is not only focus on understanding the process of the stress itself, but we also need to utilize our science to understand how effective the interventions are – what’s working and what’s not,” she said, adding that they can give city leaders a greater perspective on what they’re facing. “City decision-makers are really just on a day-by-basis. They are having to respond to the crisis of the day. But as scientists, what we need to do is help them put these day-to-day crises into the context of the past, the present and the future.”
NASA, Microsoft, and the University of Illinois are in the exploratory phase of developing a technology – the Urban Environmental Data Platform – that will deliver the information and tools that cities will need as they deal with the challenges that will come with climate change, Rosenzweig said.
Be the first to comment