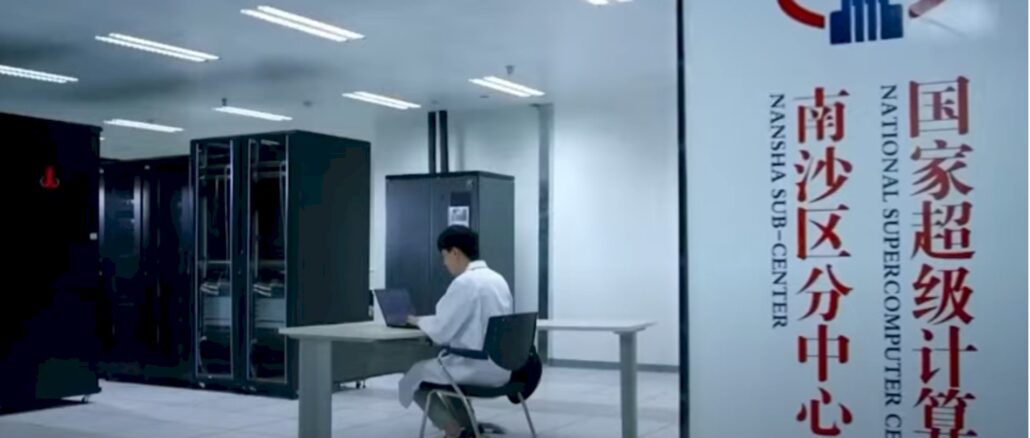
As far as we have been concerned since founding The Next Platform literally a decade ago this week, AI training and inference in the datacenter are a kind of HPC.
They are very different in some ways. HPC takes a tiny dataset and blows it up into a massive simulation like a weather or climate forecast that you can see, and AI takes a mountain of data about the world and grinds it down to a model you can pour new data through to make it answer questions. HPC and AI have different compute, memory, and bandwidth needs at different stages of their applications. But ultimately, HPC and AI training are trying to make a lot of computers behave like one to do a big job that is impossible to any other way.
If this is true, then we need to add the money being spent on traditional ModSim platforms to the money being spent for GenAI and traditional AI training and inference in the datacenter to get the “real” HPC numbers. This is what Hyperion Research has been trying to do for a few years, and it has just done a substantial revision on its HPC server spending market to take into account the large number of AI servers that are being sold to customers by Nvidia, Supermicro, and others who it has not counted in the past.
Seating was a little tight at the traditional Tuesday morning briefing by Hyperion that is given at the SC supercomputing conference, so we were a little close to the projection screens and hence at a very tight angle that makes the charts a little hard to read. Just click to enlarge them and they will be easier to read.
The big change in server spending comes from the addition of these “non-traditional suppliers,” as Hyperion calls them in its revised revenue and forecast. In 2021, there was $1.34 billion of these machines sold into the collective HPC/AI, which grew to $3.44 billion in 2022 and in the wake of the GenAI boom, rose to $5.78 billion in 2023. And the expectation is for these suppliers to rake in $7.46 billion in 2024, and that amount will almost double to $14.97 billion by 2028. The historic part of the HPC/AI server market, shown in blue in the chart above, is expected to account for $17.93 billion in revenues this year and will climb to $26.81 billion by 2028.
Add it all up, now, and then the combined HPC/AI server spending across all suppliers will account for $25.39 billion in sales this year and rise at a compound annual growth rate of 15 percent to hit $41.78 billion by 2028.
Now, not all of the spending on HPC and AI compute is on premises, of course. There is a big chunk of the IT budget for HPC and AI workloads that ends up on the cloud. And here is how Hyperion cases that, including a forecast out to 2028:
Hyperion reckons that HPC and AI applications running on the cloud together consumed $7.18 billion in virtual server capacity in 2023 and that this will grow by 21.2 percent to $8.71 billion in 2024. By 2028, HPC and AI compute capacity in the cloud will drive $15.11 billion in spending, and the compound annual growth rate for sales will be 16.1 percent from 2023 through 2028.
Compute is not the only thing in an HPC and AI system, even though it feels that way sometime with the way we all like to talk about compute engines. There is spending on storage, software, and services as well, and here is a table and chart that brings it all together:
If you add all of that up, then in 2024, Hyperion expects for total HPC and AI spending to rise by 22.4 percent from $42.4 billion to $51.9 billion. With a compound annual growth rate of 15 percent between 2023 and 2028, the whole HPC and AI enchilada will comprise $85.5 billion in spending by 2028, double the level of spending in 2028. (Note to self: A CAGR of 15 percent over five years and six periods is a factor of 2X growth over that time.)
Now, within this forecast is the vast spending on traditional exascale-class supercomputers. Here is the latest chart that shows pre-exascale and exascale system spending by region, from 2020 through 2028, inclusive. Take a look:
The number of machines by region and the value of the machines over the years is not nailed down precisely in future years because plans are not installations. Sometimes plans fall through, and other times, a plan comes together quickly to result in a new machine being installed that was not originally expected.
According to this table, China had two exascale systems installed in 2021 for $350 million each. Another one went in for $350 million in 2022 and yet another one for $350 million in 2023 and – hold on – still another one went in this year for $350 million. We have talked about Tianhe-3 and OceanLight, but the other three systems are mostly a mystery to us. China is expected to put in another one or two exascale machines in 2025, according to Hyperion, for an estimated cost of $300 million a pop, and another two after that for $300 million apiece in 2026. Let’s call it nine exascale machines for $2.95 billion, or about what Elon Musk’s xAI paid for its 100,000-GPU “Colossus” cluster.
By contrast, Japan still does not have an exascale machine at FP64 precision, and will get its first one in 2026 for the cost of $200 million. Hyperion expects Japan to install two or three machines, at a cost of $150 million a piece and for a total of $300 million to $450 million in additional costs, in 2027 and 2028. Be optimistic and call it four machines for $650 million.
Europe has had a bunch of pre-exascale systems, of course, but will get two exascale machines in 2025 at an estimated cost of $350 million each, and will add two or three machines in 2026 for around $325 million each, two or three in 2027 for $300 million each, and two or three in 2028 for $275 million each. Be optimistic and it is eleven machines at a total cost of $3.4 billion.
The United States installed one exascale machine in 2022 (“Frontier” at Oak Ridge National Laboratory) and two in 2024 (“Aurora” at Argonne National Laboratory and “El Capitan” at Lawrence Livermore National Laboratory). For some reason, this table ignores Aurora, which had a list price of $500 million but we think which cost only $200 million after so many delays by Intel, which invented its compute engines. We think that the United States spent $1.4 billion to get three exascale machines in recent years.
This Hyperion exascale system table shows a forecast of two exascale systems going into facilities in the United States in 2025 for a around $600 million each and one or two of them going in each year for a descending amount of money each year per machine: $325 million each in 2026 and $275 million each in 2027 and 2028. Add it all up and be optimistic about the number of machines being higher instead of lower, then you are talking about $4.35 billion for eleven systems.
Add all of the exascale systems in China, Japan, Europe, and the United States together and assume the best case for the highest number of machines, and you are talking about 35 exascale machines for a combined investment of around $11.35 billion. As large as that sounds, that is only a relatively small share of expected cumulative spending on HPC and AI systems in the forecast period.
Non-traditional suppliers (NTS) should be great sources for the innovative knives required at each TNP-classic trifurcation buffet of diverging performance requirements ( https://www.nextplatform.com/2022/10/06/the-art-of-system-design-as-hpc-and-ai-applications-diverge/ ) … lest one wants to go digital by computing on his/her actual fingers (best reserved for eating, in finger lickin’ good ways)! And at 21% CAGR for NTS, it’s a doubling every 4 years (a tad better than the “Note to self” 15% overall!).
I’m guessing Hyperion’s NTS include the outfits developing systolic dataflow near-memory machines as well as reconfigurable optical networking, that should help with AI and flexibly addressing workloads on the continuum from HPL to AI (along with MxP and HBM). But there’ll also be challenges with HPCG and graphs to tackle, for which innovations beyond MRDIMM might be needed (possibly retro-futuristic ones: https://www.nextplatform.com/2023/09/01/what-would-you-do-with-a-16-8-million-core-graph-processing-beast/ ).
It’s remarkable for example that under current trifurcated conundra, the CPU-only Fugaku is 6.8x faster than Frontier, and 8.5x faster than Aurora at Graph500, even as those two are, conversely, 3x faster than Fugaku on HPL ( https://graph500.org/?page_id=1332 ). Hopefully some NTS “knives” can make these diverse workloads operate with more consistent perf on future supermachines!