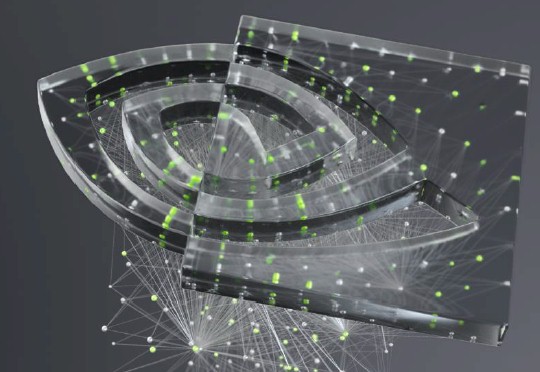
If the emergence of Nvidia in datacenter compute shows anything, it is the value of controlling the software stack as you come to dominate the compute – and the revenue and profits – in the hardware stack.
When it comes to AI, the combination of open source frameworks from the wider AI community, which Nvidia contributes to, and closed source libraries and tools that make up the Nvidia GPU Compute software stack that is underpinned by the CUDA environment, gives Nvidia the kind of control over a complete software/hardware stack that we have not seen in the datacenter since the RISC/Unix server days of the dot-com boom and earlier with proprietary systems from IBM, DEC, and HP, as well as IBM mainframes since the dawn of the data processing age.
There are some differences this time around, and they are significant. The operating system is consequential, of course, but with all AI workloads being deployed on Linux, it really doesn’t matter which one you pick. Linux is about as interchangeable as DRAM memory modules in the server and it really comes down to preferences and a few technical differentiations. And to a certain extent, the X86 server that houses the Nvidia GPUs is fairly interchangeable, too. But fi you want to make GPU compute fluid and easy, then you have to realize that not every can – or wants to – buy an Nvidia DGX-A100 or DGX-2 system. Hyperscalers and cloud builders have their own ODM suppliers, enterprises have their own OEM suppliers, and they want to be able to run the Nvidia AI stack on platforms from their suppliers rather than having to add a new vendor into the mix.
That, in a nutshell, is why the company is rolling out its Nvidia Certified Systems program. There is more to it, of course, with Nvidia now being a DGX server and HGX component supplier in its own right, including the NVSwitch GPU interconnect, as well as a supplier of Mellanox switching, network interface cards, and BlueField DPUs. A lot of the componentry in GPU accelerated systems, in fact, is going to come from Nvidia, but there are variations on themes as well as differentiations that give the ODMs and OEMs their own foot in the datacenter door. And someone has to make sure that all of this hardware in its many combinations will just run that NGC stack and get people running AI codes (and traditional HPC codes, too, which are also in the NGC stack) as quickly and as smoothly as possible.
The NGC stack is pretty widely used at this point, and Adel El-Hallak, director of product management for NGC at Nvidia, says that in the past year over 250,000 distinct users did over 1 million downloads of NGC from the Nvidia cloud and public cloud catalogs that distribute it. NCG is a kind of containerized substrate for AI and HPC workloads that is uniform across on premises and public cloud infrastructure. But up until now, it was not clear who was going to do the certification on various on premises gear. As of today, we know the answer: Nvidia is committing engineering resources and so are ODMs and OEMs working with them to ensure this is done, and once this is done, something very important can happen. Nvidia can offer technical support services for the NGC stack that have hardware issues contained at the ODMs and OEMs.
This, as much as fit and finish, is what makes NGC a true platform for enterprises. Now, companies will be able to get systems that are certified to run the NGC stack right out of the box, they can get hardware support from their ODM or OEM, and they can get software support from Nvidia. And if they buy systems from Nvidia, they can get that proverbial one throat to choke – but as we say, most companies are not going to want to do that. Just enough to make Nvidia a top system provider is our guess. (Probably north of $500 million a year but probably south of $1 billion a year, including its own supercomputer acquisitions for internal use.)
The Nvidia certification will be available for edge and datacenter use cases, and initially will be focused on guaranteeing a level of performance for AI training and inference, but we expect this to be extended to HPC workloads as well. Security testing is part of the certification, and so is scale to ensure that the NGC stack doesn’t just run on one node, but can scale up across many nodes to drive the kind of performance that enterprises will need for their AI workloads (and their HPC workloads, too).
Specifically, OEMs certify interoperability of their systems using Mellanox ConnectX-6 NICs and BlueField-2 DPUs; for networking, the systems are certified for Mellanox 8700 200 Gb/sec HDR InfiniBand director switches and Mellanox SN3700 200 Gb/sec Ethernet switches.
“Doing AI at scale is difficult,” explains El-Hallak. “In the past, we have seen that doing AI at scale has been a do-it-yourself type of program. And so the drivers for this go back to this deluge of data and the complexity of the models, which drives the need for multi-node, clustered training. We have replaced an approach that was do it yourself, figure it out for yourself and do what it takes to scale efficiently, with a program that gives you a predictable means to identify systems that deploy easily and scale easily and make the most of the AI use cases. To make this as turnkey as possible, with some predictability.”
This focus on scale is an important differentiator from other certified systems programs that we have seen in the past from the HPC crowd. These were stacks of compute, storage, and interconnect that were put together and ready to load up an operating system and MPI stack and job scheduler, but there was no certification specifically to set a performance level, and it will be interesting to see how Nvidia gauges this across certified systems. We suggest actually benchmarking systems as part of the certification, but Nvidia is not promising any such thing. But why not? When SAP was taking over the enterprise world in the late 1990s and early 2000s with its R/3 application package, it certified configurations and provided benchmarks – and smart-alecks like us went through the details and configured up the cost of the machines and did price/performance analysis across vendors and architectures. It was such great fun. . . .
At the moment, Dell, Gigabyte, Hewlett Packard Enterprise, Inspur, and Supermicro have a total of 14 systems that they have put through certification, and El-Hallak says that Nvidia has up to 70 systems coming from nearly a dozen vendors based on the pipeline it has at launch. It is not clear how the ODMs will certify, but clearly they can, and anyone who wants to grab Supermicro motherboards and chasses and create their own variations on the AI hardware theme can do so and get their machines certified. It is unclear how Nvidia may charge for such certifications, but we do know that it is expending its own engineering time on the effort and may only expect for OEMs and ODMs to do the same. What Nvidia wants is to have a lot of different hardware ready to run its NGC stack to grease the AI and HPC skids.
Given that so much of enterprise infrastructure is virtualized, we would not be surprised to see certifications for the NGC stack running in containers atop Red Hat OpenShift and VMware Tanzu which may or may not have a KVM hypervisor or ESXi hypervisor associated with it.
The launch of the certified systems program from Nvidia has also given us something we have always wanted: A list price for the support of that NGC stack. And El-Hallak, to his credit told us the price. Support costs for the NGC stack are tiered in blocks of four GPUs, and the underlying CPU doesn’t matter. (This is a GPU centric worldview, after all.) Support for the Nvidia software stack on those four GPU blocks costs $10,000 for a three-year contract. So on a DGX-2 fully loaded with 16 V100 GPU accelerators, that would work out to $40,000 or $833 per year per GPU. This machine cost $399,000 with the NGC support in it, so the underlying hardware cost $359,000 and the support represented 10 percent of the cost. On a DGX-A100, which has only eight A100 GPU accelerators (each with anywhere from 1.5X to 2.1X more oomph on HPC workloads and 6X more oomph on AI training and 7X more oomph on AI inference), the NGC stack support would cost $20,000, or again 10 percent of the $199,000 list price for this machine and $833 per GPU per year. We don’t know what, if anything, Nvidia charges for the hardware maintenance, but perhaps this is bundled in for its own customers. OEMs will charge hardware maintenance on top of this – or at least try to. And many enterprises will pay both. Happily, given that AI is a new and critical workload.
Update: Hardware maintenance is separate and also bundled by Nvidia into the DGX pricing and is separate from the NGC stack support costs.
Be the first to comment