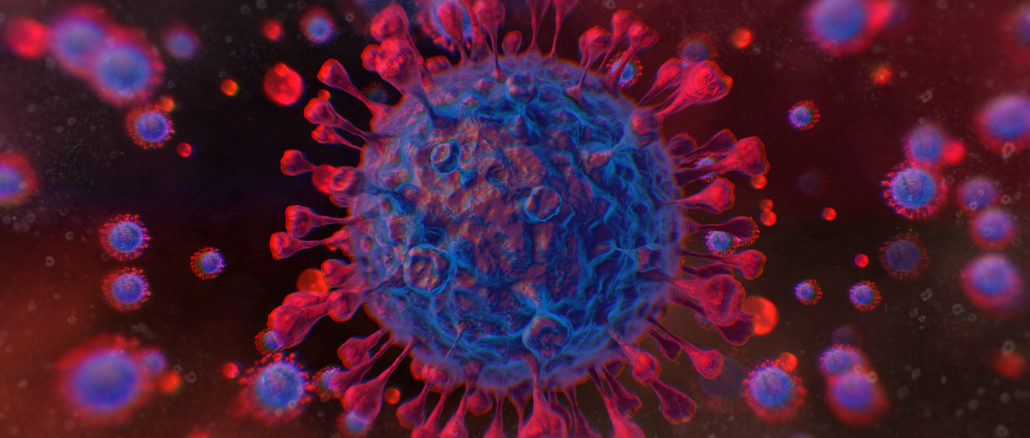
Dan Stanzione has a lot of compute power at his fingertips. As executive director of the Texas Advanced Computing Center (TACC) in Austin, Stanzione is in charge of a number of supercomputer, including “Frontera,” a Dell EMC machine powered by Xeon SP Platinum processors from Intel that was deployed last year, has more than 8,000 available compute nodes and currently sits at number five on the Top500 list with a peak performance capability of 38.7 petaflops. And right now, about 30 percent of its compute cycles are being used by engineers and medical researchers working on issues related to the COVID-19 global pandemic.
Other high performance computing systems at TACC are being used in coronavirus-related projects that address everything from the search for treatments and vaccines to understanding the nature of the virus to modeling the spread of the outbreak. That includes “Stampede2,” another Dell EMC system that is number 18 on the Top500 list, with a peak performance of 18.3 petaflops; “Longhorn,” a subsystem of Frontera based on IBM’s Power9 processors and leveraging 448 V100 GPU accelerators from Nvidia; the “Jetstream” Dell EMC-powered cloud environment, and even “Wrangler,” a Dell EMC supercomputer that the computing center is in the process of shutting down but that can still run some COVID-related workloads.
In all, 500 to 600 researchers are working on about 30 pandemic-focused projects that are running on TACC systems, Stanzione tells The Next Platform. At the same time, staffers and engineers at the center also are helping researchers with human support, from data management and visualization to building out web portals and rewriting software code.
“We put 1.5 million node hours in April on Frontera for virus stuff, and several hundred thousand node hours in the a seven-day stretch went into this,” Stanzione says. “You figure the machine can put out 8,000 nodes 24 hours in a day. That’s like two days of the seven days’ time on the entire machine went just into the coronavirus modeling. We’re obviously giving them higher priority, so it’s stretching out the queues for everybody else, but we’re doing a ton of work on this.”
Thierry Pellegrino, vice presidents of business strategy and HPC solutions for Dell EMC’s Server and Infrastructure Systems unit, tells The Next Platform that “as scientists engage in countless research efforts in response to COVID-19, HPC systems play an integral role in understanding the virus and accelerating the development of treatments. Through methods such as high-powered modeling and analysis, HPC is crucial for organizations to make new discoveries and breakthroughs.”
What’s happening with Frontera, Stampede2 and the rest of the TACC systems is important but it’s not rare. As we’ve written about in The Next Platform, countries and researchers worldwide are leveraging the massive amounts of compute power and speed stored in HCP systems and modern technologies like artificial intelligence (AI) and machine learning algorithms to enhance modeling and simulation efforts and data analytics, all to attack the coronavirus from multiple angles with the goal of gaining a deeper knowledge about it and ultimately defeating it. That includes the launch in March of the Covid-19 HPC Consortium, launched by the likes of IBM, Microsoft, Amazon and Google in a private-public partnership with the federal government to give engineers, scientists and medical researchers access to an aggregate of more than 330 petaflops of performance in 30 supercomputers around the country, including “Summit,” a hybrid IBM system with Power9 processors and Nvidia Volta V100 GPU accelerators at the Oak Ridge National Laboratory that is at the top of the Top500 list, and “Sierra”(below), another Power9 supercomputer at the Lawrence Livermore National Lab, that is second on the list.
The consortium includes 38 members and to date, 51 active projects – 11 of which from countries outside of the United States – have been given access to supercomputers.
We’ve also written about the coronavirus-related workloads running on the “Corona” supercomputer (below) at Lawrence Livermore that was built by Penguin Computing and leverages AMD CPUs and GPUs, and more recently the “Fugaku” system under development in Japan, a follow-on to the K supercomputer that has been pressed into action to help in the fight against COVID-19. And all that is essentially just scratching the surface. Microsoft teamed with universities, the National Center for Supercomputing Applications at the University of Illinois at Urbana-Champaign and enterprise AI software provider C3.ai to create the Digital Transformation Institute to provide researchers and scientists with access to supercomputers, cloud tools and AI software for their Covid-19 projects.
Hewlett Packard Enterprise also is a member of the Covid-19 HPC Consortium and projects funneled through the group as well as coming from outside of the consortium are finding their way to HPE systems, such as the “Theta” supercomputer at the Argonne Leadership Computing Facility, “Catalyst” at Lawrence Livermore and “Jean Zay” (below) at the GENCI HPC facility in France. Scientists are running AI and machine learning workloads on these systems to better understand the virus and find treatments and vaccines. Computer is about accelerating time to insight, so using supercomputing to more quickly reach therapies and vaccines makes sense, Peter Ungaro, the former Cray CEO who now is became HPE’s head of HPC and mission critical solutions after the company bought Cray last year for $1.4 billion.
“It’s something that is just a natural fit for what we’ve been doing in supercomputing,” Ungaro tells The Next Platform. “We’ve seen examples from all over, from more traditional modeling and simulation – just understanding the structure and function of the virus – to AI to looking at how we understand different chemicals and compounds that are being screened and just being able to screen millions of them vs. going through a wet lab process and really predicting the binding potential of the chemical and figuring out whether it is a viable chemical. We can focus the time and energy on those that are really viable candidates vs. the ones that aren’t. What we’re finding in using deep learning in this avenue and machine learning is that only 5 percent to 10 percent of the chemicals and compounds that look like they are potentials are really viable going forward.”
Being able to leverage AI and machine learning to quickly sort through potential candidates to find the ones most promising can accelerate the timeframe for a treatment or vaccine. And the technologies can be used for a broad array of workloads, including modeling the impacts of policy decisions, such as when to open up businesses – a key one now as states begin to rev up their economies – to patient trajectory models and ventilator and other resource allocations.
“You have the ability to have more processing than you’ve ever had before,” he says. “You have the ability to leverage a very high-performance network and high-performance storage. So how do you take an application that maybe was running on a departmental system in a university and run it on one of these huge supercomputers and take advantage of all of that? Different technology plays out in different applications, so I wouldn’t say that it’s one technology or another, like CPUs vs. GPUs. We’re matching the right technology to the right problems. It’s really more about helping people to take advantage of the resources that are available to them, because many of our customers – not just in the U.S, but around the world – have opened up their supercomputers to researchers doing work in this area. How we best leverage that capability is really important.”
TACC’s Stanzione says the workloads running on the center’s systems fall into three broad categories, with one being basic biology and molecular dynamics. Much of the drug screening work comes from understanding the molecular level of the virus’s actions, such as the cells it attacks, and this comes from research around chemistry, molecular dynamics and simulations. These are the most compute-intensive workloads, he says. There’s also genomics work to understand the novel coronavirus’ evolution, where “you look at the host genome that it’s infecting, see what the factors are in the genome that lead to better outcomes [and] worse outcomes and different infection rates. You look at the evolution of the virus through different organisms to see what may work as an effective treatment there,” Stanzione says.
The third area is the epidemiology work, including modeling at the whole-person and society levels. The work looks at such areas as the spread of the coronavirus and how people are interacting, where they’re traveling, how much they’re moving about and how social distancing can mitigate the spread. There’s also the forecasting of trends like hospitalization and death rates.
“Computationally, the molecular dynamics is the biggest job because of the volume of what we can do there,” he says. “It’s a big simulation. If you’re doing the basic molecular dynamics, you’re looking at hundreds of millions of atoms. That’s something we can spin up doing a lot of 3,000-node runs. That’s 150,000 orders. We can work on that problem at one time. Forty-eight hours to do one structural run. The drug screening, there’s a few million molecules we know we can synthesize. There’s actually trillions of possible ones. When you’re doing what they call the docking computations, where you’re trying to fit a molecule over the virus structure, they’re computationally large and we’re trying literally millions of combinations against tens of different sites of the molecule. … You have billions of runs to do there pretty easily. In terms of cycles that we’re putting out on the supercomputers, that’s the number-one area.”
It’s the epidemiology work that has the fastest impact, Stanzione says, adding that “you see those in the papers every day. People make policy decisions on them, sharing with the general public and they’re computationally smaller. We’re doing sort of Monte Carlo runs of these tens of thousands of runs. It uses thousands of node hours. It’s not something you’d want to do on a laptop kind of scale. It’s just nowhere near as big as the molecular dynamics, but those are where we’ve been doing small molecule docketing and molecular dynamics. Those are pretty well-established fields of computational science with decades into the codes, whereas half these epidemiology and virus spread models are pretty new at large scale. We’re putting a lot more of our staff time into the epidemiology stuff just because they’re not really traditional computational sciences. The portal scheme that this team, the various code support teams are probably spending more time on epidemiology than anything else. Computers are spending more time on the molecular dynamics than anything else.”
AI and machine learning are playing important roles, but right now more than 80 percent of the work is done via more conventional methods. There are challenges with AI and deep learning in explaining how neural networks draw their conclusions and in verifying the outcomes, he says. However, that’s offset by what these technologies can do right now. Supercomputing centers are throwing massive amounts of resources – from technology and human resources to government dollars – at the problem and AI techniques help in using those resources more efficiently.
“If the AI drug screening works, we can’t necessarily explain what we’re doing with it,” he says. “But even if we don’t really know why it’s saying that this molecule looks like a good candidate or this one looks like a good candidate, if we’re trying to screen across billions of possible molecules or combinations of molecules and insights, then we can still run our very well understood first-principle simulations on the ones the AI says to look at and get a very rigorous and explainable answer from that. But the AI may take two orders of magnitude off the number of cases we have to run, even if we don’t know exactly in terms of physical principles how it’s doing that. It’s a great thing to reduce the amount of work that we have to do and really optimize what we’re doing.”
The coronavirus’ entrance onto the scene comes at an interesting time in HPC. With the rise of such technologies as GPU accelerators and technologies like AI, the capabilities are significantly better now then even a few years ago. Stanzione notes that Frontera is almost a 40-petaflop system; the first Stampede a few years ago had only a quarter of that. Computationally, the field is probably 20 to 50 times more capable. In addition, researchers and scientists already have been through similar tests over the past couple of decades, from SARS to Ebola to H1N1, so the combination of more compute power and experienced researchers puts the world in a better position with COVID-19, Stanzione says.
That said, the industry is a year away from the first US exascale-level systems – “Aurora” and “Frontier,” both HPE/Cray systems – coming online. How would things look if the pandemic hit in three years rather than now?
“That would have been amazing to think about, these exascale supercomputers with all of this advanced capability that they’re going to have being available to the researchers today to solve these kinds of problems,” HPE’s Ungaro says. “Those machines – the three systems that have already been announced – would be probably two orders of magnitude more capacity and capabilities than the fastest systems today. It’s pretty exciting overall what these machines will bring to researchers, especially from an AI perspective. You’re one order of magnitude from a traditional modeling and simulation and probably two orders of magnitude from an AI perspective.”
Stanzione expects the TACC systems will keep running at their current pace on COVID-19-related projects for several weeks before the work on treatments and vaccines moves to the next level.
“The good news is for a lot of the clinical trials and for both treatments and vaccines, it moves to the medical chemists and then it moves to the human and clinical work, which is long in calendar time compared to the supercomputing,” he says. “We will have given them a bunch of candidates in the next month or two and the epidemiological models will move into more of a retrospective thing once we’re sort of past the near-term crisis. That will ramp down, but I think there’s going to be a lot of basic science to do to understand this better, to understand what went on and to get us ready for the next thing that crops up. We probably will stop treating it as emergency computing that squeezes out all other fields in the next two months or so, but I imagine we’re going to be working on this problem for years to come. It’s not going anywhere.”
Be the first to comment