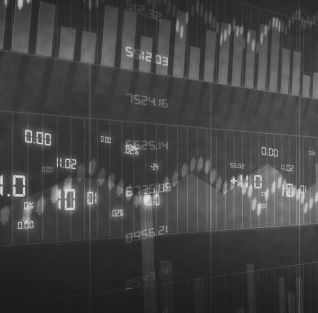
Data analytics is a rapidly evolving field, and IBM and other vendors over the past several years have built numerous tools to address segments of it. But now Big Blue is shifting its focus to give data scientists and developers the technologies they need more easily and quickly analyze the data and derive insights that they can apply to their businesses strategies.
“We have [created] a ton of different products that solve parts of the problem,” Rob Thomas, general manager of IBM Analytics, tells The Next Platform. “We’re moving toward a strategy of developing platforms for analytics. This trend of products to platforms is a dominant one in how we’re building out what we’re doing here for analytics.”
IBM’s latest case in point is the Integrated Analytics System, which as the name suggests is a unified platform for chewing on data that is designed to enable data scientists to apply advanced analytics wherever the data resides – whether on public, private, or hybrid clouds – and to view all that data as a single pool. They can also move workloads from one data store to another and collaborate regardless of the programming language they are working in. The Integrated Analytics System also leverages machine learning techniques and integrated data science tools to help automate many of the manual processes involved in data analytics, from ETL to data cleansing.
The more manual processes that can be automated, the more time the data scientists will have for more value-add jobs like model deployment and training, which drive the deeper insights and intelligence that organizations can use to drive their business efforts, Thomas says. Some early users of the technology have been able to reduce the amount of manual processes from 80 percent to 15 percent or less, he adds.
At the core of the Integrated Analytics System, introduced this week at the Strata Data Conference, is the IBM common SQL engine, which enables businesses to easily move workloads to the public cloud, a key step in helping to automate processes through machine learning and to enable them to scale their analytics efforts. Many businesses currently are not leveraging the public cloud because of the challenges to migrating their workloads. By using common code for public, private and hybrid clouds, moving the workloads is no longer a hurdle, he said. A variety of data types are supported, including the Watson Data Platform, Db2 Warehouse on Cloud, Hadoop and IBM’s BigSQL.
In addition, using the IBM common SQL engine enables data scientists to move and query data across multiple data stores, including Db2 Warehouse on Cloud and Hortonworks Data Platform.
Another key part of the analytics offering is the integration of the IBM Data Science Experiment, Apache Spark and Db2 Warehouse, all of which have been optimized to work together. The Data Science Experience includes a set of development tools – including Jupyter Notebooks for working with Python, R and Scala – and RStudio for development in R and machine learning capabilities – for building intelligent applications, and having Spark included brings in-memory data processing that enables data scientists to bring the analytics capabilities to where the data is. The embedded machine learning also lends to the ability to moving the analytics processing to the data, which IBM officials said reduces the processes involved in analytic chain and accelerates the training and evaluating of predictive models by putting the testing, deployment and training in one place.
That is a key differentiator for IBM, Thomas explains. Most data analytics tools on the market require the data to be moved to the application. It is more efficient to bring the analytics to the data – and that is the genius of the MapReduce technique invented by Google so long ago and copied by Yahoo to create Hadoop. Thomas used the example of a financial services firm that is using data from multiple sources, such as customer profiles and stock portfolios. The data can be encrypted and secure inside the Integrated Analytics Systems and then, using the common SQL code, data scientists can federate the data in the public cloud and unstructured data that’s sitting in a Hadoop environment. The customer and stock data remains secured, while other data sets are run through the platform.
“It’s better to run the data inside the analytics by bringing the analytics to where the data is,” Thomas said.
The new system leverages technologies such as asymmetric massively parallel processing (AMPP) with IBM’s Power-based servers. It also uses flash memory hardware and takes advantage of capabilities from IBM’s PureData System for Analytics and Netezza data warehouse technologies.
Be the first to comment