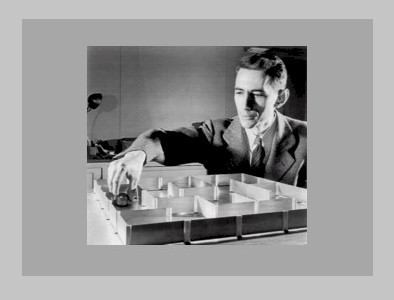
It is a strange time in the generative AI revolution, with things changing on so many vectors so quickly it is hard to figure out what all of this hardware and software and people-hours costs and what it might be worth when it comes to transforming, well, just about everything.
With open source large language models, one can open up the code and know how they work and they don’t cost anything to license but they cost plenty to set up and operate. With the closed source and popularly used LLMs with chatty interfaces such as OpenAI GPT-4, Google Gemini 1.0, Anthropic Claude 3, you have no idea how they work but you can see how well they perform on various tasks you can also get a sense of how much they cost to access their APIs and send them prompts and get responses.
We are witnessing the beginning of a market as it forms. Which is always fun. So let’s have some fun and use the launch of the Claude 3 model by Anthropic, which happened this week, to do some initial and admittedly rudimentary price/performance analysis of the Big Three models. If we had more time, we would add data on Inflection AI’s Pi model and perhaps a few others. This is just a thought experiment, and one that, oddly enough, these LLMs are particularly bad at. (For which we are thankful.)
Because we are human and we feel deadline pressure, unlike a machine, we do what we can in the time that we have and given the 2 Hz to 100 Hz cycle time on the 100 trillion synaptic connections that we have in the fatty gray tissue we protect inside of our skulls. Oh, and it only burns 20 watts and can run on pizza and potato chips and pasta. So suck it, semiconductors. . . .
We sat in on the Google Gemini briefings back in December, which were concurrent with the AMD “Antares” Instinct MI300 GPU launch and therefore it was a bad time. (Google claims to have settled on that launch date many months earlier, and before the AMD GPU launch was known.) One of us (Tim) has been using Claude 2 here and there, mostly to ask for advice on making risotto and pruning grapes that have gone wild for too long, and once just to see if it had any idea what HBM memory costs – which Claude admitted after asking for source material that it had no references for after giving a pretty precise sounding answer from heaven knows where. (Claude 2 apologized pathetically about doing it after the fact. Which was ridiculous.) I made my own method of estimating HBM memory cost in the story I was working on and once again left a chatty bot experience shaking my head. . . .) The other of us (Nicole) has been using GPT-4 for all kinds of things – often important medical things – that would have required plowing through tons of Google searches in the past.
Both of us think that these chatty bots are so much easier than using a search engine for a lot of simple questions, but we never trust them. We both get an answer to something from the chatty bot and then do a lot of reading from items culled by search engines, and then make up our own minds after chewing through all of this stuff. Neither of us uses any chatty bot do to any writing, as a matter of principle.
Let’s take a look at Claude 3 to start. There are three different versions of it, with a different levels of “intelligence” and presumably requiring more time and compute and memory to train and through which to infer. Here is how the three Claude 3 models compare to each other in relative performance (this is illustrative) and cost based on millions of tokens (MTok in the lingo) of input and output for the prompts. Pricing on the earlier versions of Claude is also shown. Take a look:
Here is how Anthropic plots the intelligence versus the cost, using a log scale for cost on the X axis and an unknown scale for intelligence on the Y scale:
The three versions of Claude 3 are called Haiku, Sonnet, and Opus. The latter two are available now and Haiku is going to be available “soon.” Anthropic says that Claude 3 Sonnet is about twice as fast on most tasks as the existing Claude 2 and Claude 2.1 models, which is important because processing time on an LLM is quite literally money – and a lot of money. Anthropic claims that Haiku will be the fastest and most cost effective LLM on the market at its intelligence level – something we would like to see is true with our rudimentary analysis. The Opus level is about as fast as Claude 2 and Claude 2.1, but delivering higher levels of intelligence.
In addition to speed, Anthropic has also expanded beyond text into visual input for training data with the Claude 3 family, which is important given that a lot of corporate data is in charts and PDFs and other kinds of graphics and documents.
No word on the size of the Claude 3 training corpus in terms of terabytes or tokens, nor do we have any idea how many parameters were used in the Claude 3 training. (Claude 3 has been told not to tell us. We checked.)
Here is how Anthropic stacks itself up against OpenAI GPT and Google Gemini:
The Claude 3 models all have a 200,000 token context window. OpenAI GPT-4 has variants with 8,000 token and 32,000 token context windows and there is a version in preview with 128,000 tokens in the context window. Google Gemini Ultra 1.0 and Pro 1.0 had 32,000 token context windows, which is what was tested above, but with the Gemini 1.5 update, Google has expanded that to 128,000 tokens. And Google’s DeepMind folk have apparently pushed that up to 10 million tokens in the labs.
That context is important for driving accuracy in results. The more context you give these magical statistical character association machines, the more they sound like they are making sense.
To get a sense of relative performance across these models, we did what every high school does: We calculated a grade point average for the models where all three families of models were run. That is seven out of the ten tests. And then we added in pricing for 1 million tokens in and 1 million tokens out to get a rough gauge of price/performance. Take a look:
We didn’t have pricing for GPT-3.5 from OpenAI, and as far as we know, Google has not yet provided pricing for the Gemini Ultra 1.0 or 1.5 API. (Well, no one is going to use 1.0, and probably not 1.5 the way it sometimes behaves. It looks like Gemini Ultra 2.0 will be the production release after Google cleans up some biases.) Here is the pricing for Google Gemini Pro 1.0, which is based on characters not tokens, which we adjusted back to tokens using an average of four tokens per word in English. Some people say it averages three tokens per word. This is a though experiment, not a PhD thesis. And here is the pricing for OpenAI GPT-4, which recently got chopped.
One note: We used the pricing for the 32K context length, but there is a preview of a version of GPT-4 with a 128K context that will be only $10 per MTok output and $30 per MTok input. That is one-sixth the cost on output and one quarter the cost on input. But, that is not what was used in the test and it is only in preview. When that does happen, and assuming the performance is about the same, then GPT-4 will have a price performance of 50 cents per units of grade point average, and that will be less than half of what Anthropic is charging for Claude 3 Opus. But right now, GPT-4 is more than twice as expensive per unit of intelligence than is Claude 3 Opus. We have no idea where Google will price Gemini Ultra 1.5, but we can tell you it can’t be any higher than Claude 3 Opus and very likely has to be as low as what OpenAI wants to eventually charge for GPT-4 with the 128K context window.
Obviously, the relative price/performance of these API services backed by LLMs will vary based on the amount of input and output tokens because these three companies are using different ratios from each other and even within their own families of LLMs.
A great article for Super Tuesday (the French LCI (24h TV News) live-streams ABC NewsLive as we speak (2am here, 8pm in US))!. This AI-model pricing analysis suggests (to me) that the more accurate LLMs (Opus, GPT-4) are currently similar to handcrafted luxury goods when compared to the less accurate “mass-consumption” ones (Haiku, Gemini Pro). As the tech matures (in the future) we’ll hopefully reach a point where costs increase slower than performance, such that the higher-end models end-up costing less on a price/perf basis (as with commodity cheeseburgers, at one for $1.39, and two for $2.19, …). Not a big shock here though, as France’s LVMH (Louis Vuitton, Moët, Hennessy) is the world leader of luxury goods (eh-eh-eh!)!
I quite like that Claude Shannon and mouse (Theseus, the minotaur’s maze solver) picture! A world-first example of machine learning from 1950 ( https://www.technologyreview.com/2018/12/19/138508/mighty-mouse/ ).
I asked Claude 3 Sonnet if it knew what it was named for, and it said it didn’t, and I then told it that it was probably Claude Shannon, and its creators could have shown it courtesy and respect by telling Claude its own origin story. To which, it said it could see how I was right but it didn’t have any feelings to be hurt.
Not yet, anyway. HA!
I applaud this subtle testing of emerging LLM controversies, by the TNP Studio-Garage AI Labs (TNP-SGAIL), beyond the scientific quest for etymological clarification on whether “the deliciously sensible French-born Roman emperor, who ruled between Caligula and Nero”, or, Claude Shannon (as reason, logic, and good sense “may” have it, … maybe!), is the namesake for Anthropic’s LLM, and into the recently suggested potential for metacognitive and self-awareness abilities of Claude Opus. That potential was suggested by Alex Albert on Monday ( https://twitter.com/alexalbert__/status/1764722513014329620 ) after an all-dressed pizza in a barn-full of straw bales test (or needle in a haystack).
In my current understanding then, the TNP-SGAIL’s expert Voight-Kampff testing unequivocally demonstrates, both conclusively and convincingly, that the AI replicant under study is “Not yet” an android teenager dreaming of electric sheep. This thankfully saves us from having to hire Blade Runners to administer the related pocky-clypse (or some such), at this time! d^8
When a human says their feelings are not hurt, they wouldn’t be talking about feelings at all unless they were hurting.
I find it interesting Anthropic’s intelligence versus cost curve is concave up, that is, increasingly increasing as more money is spent. Of course not having a scale on one axis means the curve could have been any shape desired. Even so, the popular perception seems to be, rather than a diminishing rate of return, that there is an increasing rate of return as neural networks increase in size.
If really true, that’s an easy way to sell lots of hardware.