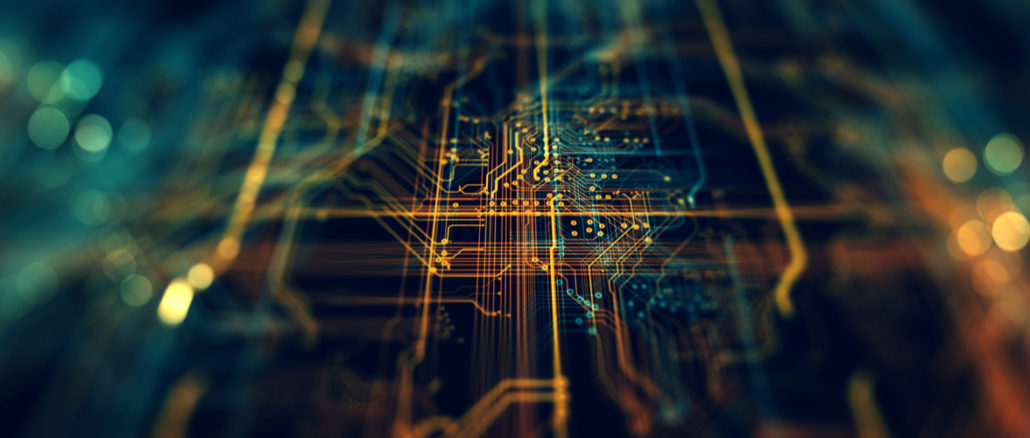
When it comes to a silicon startup bringing a product to market in a tough competitive landscape, nothing is easy. The list of challenges is long but to be taken seriously against the incumbents, a strong MLperf showing is now paramount.
As usual, Nvidia and Google swept the MLPerf results this time around with the DGX A100 system and TPU4 respectively. While those results can be found here we wanted to leave the clear leaders performance-wise aside thist ime and look at startup Graphcore’s results which, while not chart-topping, do show some notable price/performance advantages.
Since this is a benchmark that is ostensibly being used to help potential sites make decisions about what to deploy, this seems like an important piece, especially with training costs soaring along model size curves. We can always infer the cost of a system used for MLperf but for this training iteration of MLPerf, Graphcore lays it out: their POD16 system costs half what a DGX A100 does and provides on-point performance. That is, for two models, only one of which (NLP-focused) has direct correlation in the real world these days.
Performance might be paramount for the largest users of AI/ML training systems but we’re quickly hitting a point when that price/performance figure will be the defining metric, especially as successive, more expensive hardware hits the market in the coming years. AI training is already elite, but how will the middle of the market look at dedicated training hardware purchases in five years?
It is worth the heavy software lifting and hardware resource dedication for Graphcore to do the MLPerf dance. It’s legitimizing and lets them tell that price story that is more pressing than mere scalable performance these days: sacrifice only a bit on performance to get something you can afford now, something built to scale with the addition of more boxes, and keep costs as low as possible. And at the same time, by going through the hell of serving a benchmark with limited internal resources, learn more about just how far you can push your system and software stack.
Graphcore’s Matt Fyles says that it’s taken several months of optimization while undergoing work for existing users on their internal hardware, but they’ve finally managed to bring results in both the open and closed sets for two models, ResNet-50 for computer vision and BERT for natural language processing. “When MLPerf was introduced we were reluctant to contribute because we weren’t sure about the benefits to our customers. But as it gained momentum, we knew we had to do MLPerf. We have to show our tech can play in all the same boxes with all the same constraints as competitive technologies do.”
And by competitive technologies, Fyles certainly means Nvidia, which he mentioned often during a call to go over the results. The GPU maker’s DGX A100 results are standard by which Graphcore is placing their performance results. And while there aren’t massive gains for the POD6 over the DGX A100 necessarily, it’s the price performance difference Graphcore wants to push.
As a startup, it wasn’t quite possible for Graphcore to run its MLPerf results on anything higher than its POD64 system (shown below) for BERT with the POD16 machine for less hearty ResNet-50 math. Google and Nvidia bring full-on supercomputers to the MLperf match. “We can scale up to 64,000. It’s not something we’ll do next week but we’re definitely looking to get to the others early” Fyles says. “The sweet spot for our actual customers is around POD16 and POD64, those are the people bringing IPIs in to experiment. The ease of scaling is built into our architecture.”
“We could have done more with a more of a scaled-out system but we are showing in these benchmarks how we configured the machines to take advantage of disaggregation,” Fyles says. He adds that by choosing different configurations to meet the needs of different workloads (ResNet vs pre-processing/data intensive BERT) they can show off how the different systems can provide tuned performance and efficiency, depending on training requirements.
Graphcore is aiming directly for Nvidia’s DGX A100. “We want to bring a different hardware architecture and platform that’s not just another HBM-based accelerator system. Nvidia hasn’t published the A100 system price but based on market intelligence and what resellers tell us, it’s around $300,000. A POD16 from us is $149,000.”
Graphcore plans to do future training runs for MLPerf that go beyond the POD64 configuration in the next iterations of the benchmark with systems that are more on par with what Google and Nvidia are submitting, although that’s a stretch for now. “We want to do this in 128, 256, and while it won’t be next week, 512,” Fyles says. “This is an investment to show we’re here, we’re in this space, and we’re competing on benchmarks and applications in the same way as others.”
The scalable systems, while matching pure performance against the DGX A100 or TPU4 when normalizing results, are nothing to sneeze at. Seeing the software scalability alone of the 512-class system in the wild will be interesting, although we wonder if that will be ready by the next MLPerf training run.
Remember what was said above about finding an angle when you’re at a slight performance disadvantage? Finding a unique angle. Another tried and true trick is cherry picking which segments of the benchmark you want to report, something that MLPerf runners are notorious for doing. Graphcore went entirely beyond the MLPerf suite today and picked out one particular benchmark, EfficientNet, which they think signifies elements that are important to some of their early customers. Here, they were able to show even more dramatic price per dollar advantages against Nvidia’s DGX A100:
“We’re delighted to be sharing our outstanding results – BERT time-to-train of just over 9 minutes and ResNet-50 time-to-train of 14.5 minutes on our IPU-POD64,
Fyles says. “This is supercomputer levels of AI performance, putting us firmly ahead of NVIDIA on performance-per-dollar metrics.”
Very perplexed on the article as well as the company claims; my main critiques:
a. 16 of their TSMC fabricated 7nm 823mm^2 IPU’s vs 8 of Nvidia’s TSMC fabricated 7nm 826mm^2 A100’s (twice the silicon).
b. Much smaller memory capacity and slower (comparison done on 80GB A100’s rather than 40GB ones, which include a 1.5x price premium, while the models used are TINY).
c. DGX systems are NOT base sys. In another comparison Supermicro system showed 1.6x Price/$ advantage in ResNet model and 1.3x advantage in BERT model in favor of nVidia (https://semianalysis.com/graphcore-looks-like-a-complete-failure-in-machine-learning-training-performance/).
d. One (ONE !!!) NLPerf benchmark exposed per sys. Nvidia, 4 GPUs and all the 4096 tests (the ONLY one to do so). Google with few benchmarks, Graphcore, 4 different system configurations, 2 different software stacks, 4 total results. Huawei and Intel/Habana submitted multiple results for a system.
OK marketing claim, but please be attentive on the reality: their AI ASIC are TODAY not comparable with OLDER nVidia GPU, power consumption way better, a far more versatile chip. Additional elements ANYONE should remember:
1. no manager who is even worthy of this title would forget the importance of the ecosystem (of course, if knowledgeable about it and many are NOT);
2. I do not need to do only datacenter stuff; with nVidia, I can go from Top500, to 4U servers in Datacenters to the Edge WITH THE SAME SOFTWARE;
3. RAPIDS lowers in an important way the step / barrier at the entrance for developers;
4. far easier to find people on their tech that on anything else.