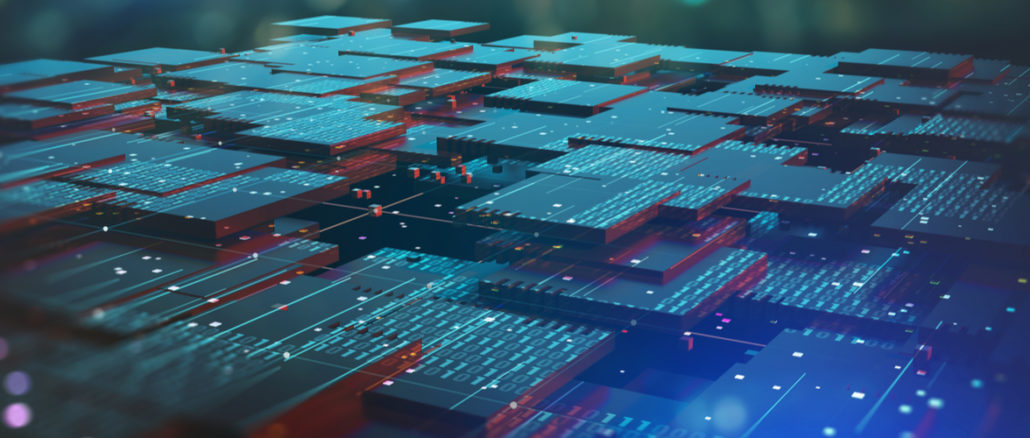
We knew the day would come when an AI chip startup hit the $1 billion funding mark and so it has.
SambaNova Systems has announced its Series D round of a fresh $676 million with SoftBank leading the charge, bringing the company to over one billion with a valuation SambaNova says is at the $5 billion level.
This is quite a feat for a company that does not appear to have many publicly cited customers beyond national labs, including Lawrence Livermore National Laboratory and Argonne National Laboratory but it’s 2021 and if we know anything by now it’s that money, at least in this industry, is ethereal. When it comes to the AI chip startup landscape, it’s not just SambaNova that serves as a reminder of this, either. More on that later this week—and on the idea that there must be multiple customers that are not public.
We have to assume that Intel Capital, Google Ventures, BlackRock, and Walden International (investors in various SambaNova rounds, including this Series D) have lenses the rest of us lack. They’ve been placing big bets on the future of SambaNova’s customer roll and technical approach, which includes both appliance-based DataScale systems and their subscription-based Dataflow-as-a-Service (DaaS) platform. Systems aside, perhaps the greatest asset the company has as it continues to propel itself forward following its 2017 start is its founder, the “father of the multicore processor” Dr. Kunle Olukotun, along with his co-founder, Chris Re, Stanford professor and MacArthur Genius Award recipient.
The legitimacy of these founding forces, along with the staggering venture capital means we should be seeing bigger things from SambaNova—and soon.
So where will they place their focus and fresh funds? And how will it stack up against standard hardware including GPUs and CPUs—not in terms of benchmarked performance but in how open potential large-scale customers will be to the idea of implementing something that doesn’t have the time-tested software tooling and robust roadmap? At the end of the day, some customers are willing to leave some performance or capability on the table for the above elements. But then again, some aren’t.
So who are those needy customers seeking a fast escape from general purpose processors and accelerators? And, even in this “funny money” world of AI chip startups, are there enough of those customers with high-enough value workloads to warrant this kind of valuation and investment? And not to overburden with questions, but for the highest-value CSPs if they like what you do, they’re going to just built it themselves, thank you.
SambaNova’s VP of Product, Marshall Choy says that for high-value workloads in recommendation, speech, and definitely computer vision, there is a lot of demand to ditch the GPU and find better memory balance and avoid chopping up data and images to force a fit. He says they are making big strides in a wide range of key large enterprise areas. SambaNova is not focusing on every AI use case, but those that matter at scale—the biggest problems in NLP and computer vision in particular. For those customers, SambaNova is betting on their need for fast acceleration at scale and without any of the heavy delays of training data science and other teams to have access to speedy, operational AI/ML. The whole concept of their DaaS is that everything from model optimization and deployment is handled on the backend without end user involvement. The heralded “magic box” for AI.
“We are focusing on enhancing DaaS as a core go-to-market offering and strategy,” Choy tells us. When asked if they have any reason to plans to just exit the hardware business entirely (and all of its overhead and margin loss) and just do software, he says that the DataScale hardware is still a critical piece of that their strategy, that the DaaS is just another way to reach key markets, including ecommerce for recommendation, healthcare with NLP and high-res image processing, energy and banking, and the one area where it has some traction, HPC/AI convergences.
“We are still doing the DataScale hardware product, it enables a lot but with DaaS we’re raining the level of value we can deliver with an extensible ML platform service. Whether it’s computer vision, language processing or other workloads, we want to give users the ability to abstract out the infrastructure and just make API calls into the system,” Choy explains. “For instance, if we have a large GPT or BERT model we can handle all the optimization and bringup, short-circuiting deployment by many months if not a year in terms of planning and optimization. It’s just an additional route to market in many form factors and consumption models depending on what is needed, we’re just broadening the market for the Datascale hardware.”
Out of all workloads and verticals, where SambaNova is shining brightest is in computer vision, especially for areas like medical imaging. He says that unlike GPUs where downsampling and tiling of images to fit means lessened resolution and quality. Their devices can handle 50k x 50k resolution, making SambaNova especially viable in processing the entire image slide without loss of quality or data. He says there is room in the market for better performance and scalability and while indeed, GPUs have market share, it doesn’t necessarily mean it’s the best tool for plenty of emerging workloads in speech and image.
Further growth areas include commercial HPC areas in pharma and oil and gas where the company’s ability to bridge the HPC/AI divide as proving out at LLNL and Argonne is gaining traction. SambaNova is up to around 400 employees and Choy hinted about expansion beyond its current North America and Europe roots.
But here’s the thing about an area like oil and gas. The money is big for systems but the software is old as dirt. GPUs are only just really hitting mainstream at many of the OG majors and even then, it’s only for a slice of the workloads. In other words, going after the high-value markets with a new device is tough–and not just because of the newness factor. Software has been created over decades and porting it to something different is a many-months undertaking. Pharma’s molecular dynamics and other HPC workloads are similar. This is the struggle for AI chip startups right now–how to get the big names doing high-value work that can a) talk about it b) have it displace enough of the existing workload to make a big difference and c) show those users that despite performance gains from specialized ASICs that software stack, roadmap, support system, and so on is worth the risk.
So, on the business end, what else could be next? And have they just VC’d themselves out of the running for a future acquisition? After all, when we’ve seen Intel Capital invest heavily in AI hardware it generally tends to land back at the mothership (Nervana and Habana, for instance)? In Choy’s view, there is plenty of market share to be grabbed, it’s a matter of finding the workloads that are well-suited to their architecture and giving those customers choices in how they deploy and with what level of guidance. Without OEM partners to push their chips into existing systems, it means a CAPEX intensive business building and deploying all that hardware, no matter how it’s managed. But with $1 billion, all things are possible.
“SambaNova has created a leading systems architecture that is flexible, efficient and scalable. This provides a holistic software and hardware solution for customers and alleviates the additional complexity driven by single technology component solutions,” said Deep Nishar, Senior Managing Partner at SoftBank Investment Advisers. “We are excited to partner with Rodrigo and the SambaNova team to support their mission of bringing advanced AI solutions to organizations globally.”
“We’re here to revolutionize the AI market, and this round greatly accelerates that mission,” said Rodrigo Liang, SambaNova co-founder and CEO. “Traditional CPU and GPU architectures have reached their computational limits. To truly unleash AI’s potential to solve humanity’s greatest technology challenges, a new approach is needed. We’ve figured out that approach, and it’s exciting to see a wealth of prudent investors validate that.”
“Traditional CPU and GPU architectures have reached their computational limits.”
someone better tell Jensen Huang
when IPO, go for it mow.