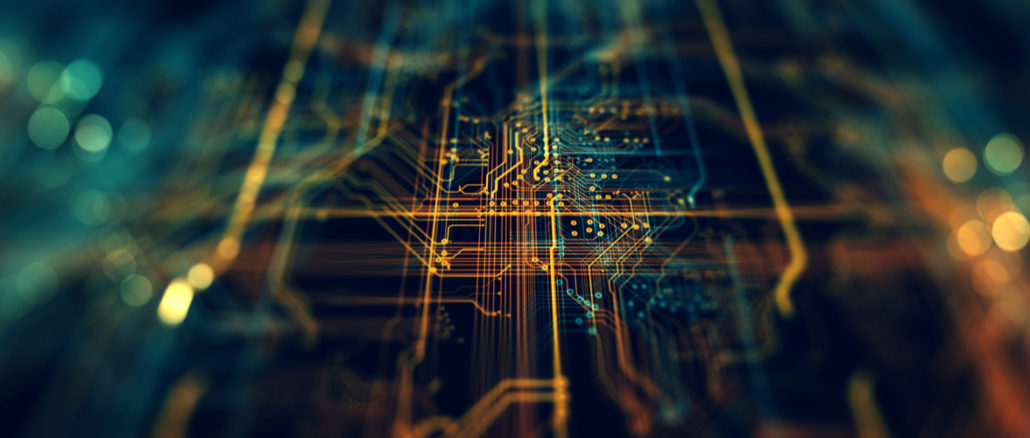
A lot of the people who are working at the many AI chip startups have a long history in processor development in the datacenter, and that is certainly true of the folks who founded SambaNova Systems. And this is a fortunate thing because these people can leverage some of the good ideas they know worked when commercializing a new technology and avoid some of the big mistakes their former employers sometimes made.
At our recent The Next AI Platform event, we sat down with Rodrigo Liang, co-founder and chief executive officer of SambaNova Systems, which is one of the upstart custom AI chip producers vying for attention and budget dollars. SambaNova was founded in 2017 by a bunch of ex-Sun Microsystems techies as well as a few from Stanford University, which is of course where Sun itself was born in 1982. The co-founders include Kunle Olukotun and Chris Ré, professors at Stanford, with Olukotun being the leader of the Hydra chip multiprocessor research project and sometimes known as the father of the multicore processor.
Liang is in charge as CEO because he has both technical and business experience. Back during the dot-com boom, Liang was a hardware manager at Hewlett Packard and did a short stint at a semiconductor startup called inSilicon before becoming the director of engineering at a startup called Afara Websystems that in 2001, just before the dot-com bubble burst, decided to make a clone Sparc chip that was massively cored and threaded. That Afara chip laid the groundwork for the “Niagara” Sparc T1 family of processors, which Liang was in charge of for the next eight year, and after Oracle bought Sun in 2010, Liang stayed on and worked on a dozen Sparc chips that Oracle put in the field. When Oracle quietly pulled the plug on its Sparc efforts in late 2017, the three founders got together to design a custom ASIC and software stack for machine learning and analytics, resulting in the SambaNova DataScale platform that is based on the company’s Reconfigurable Dataflow Unit, or RDU.
“A lot of us think that this is one of the biggest transitions that the computer industry is going to see, certainly since the Internet,” Liang explained in his talk during our event, referring to artificial intelligence and the hardware and software that is evolving to support it. “And if you look at what is available today, most of the architectures that are really playing in that space have been around for quite a while. And the question really isn’t about celebrating AI today. It’s really about what is the future of computing will look like, and we are starting to see a lot of new architectures coming in to try to figure out how to solve that problem.”
The new architectures are being driven by three main issues, says Liang. First, datacenters are in a crisis when it comes to power, sprawl, and cost, which is compelling companies to create new architectures for data processing – not just AI. The second issue is not the massive amounts of data that is being created and stored – that is a given at this point – but more importantly the turning of that data into value for a business. This is easy to say, but exceedingly difficult to do, as readers of The Next Platform know full well. And importantly, every industry is being turned upside down by this transition and those who can convert data into information and then into money will be the winners in each industry and those who can’t, well, they will be eaten like Sun Microsystems was by Oracle. The third thing is that companies want is to be able to create applications that take advantage of a new architecture easily.
“It’s about ease of development, ease of deployment, and ultimately coding value faster,” Liang says. “And for us, it is thinking about this is as a much more general case – thinking beyond AI – and turning this into a computer industry transition. It is about how do we actually accelerate development of technology to enable people to do things that they can’t do today.”
Unlike a lot of startups in the chip business, SambaNova started out with the software stack envisioned in the research of Olukotun and Ré at Stanford University.
“If you think about the problems that we’re seeing struggling with today, it’s about large datasets and how do you actually make all of that data useful,” says Liang. “So my co-founders spent a lot of time thinking about what does the compiler need to look like – and even beyond the compiler, what does the entire dataflow software stack needs to look like. I think they spent quite a bit of time researching that. And from the company’s perspective, we started in the same way, taking some of those ideas and convictions and taking the path to commercialize them, to try and figure out what does a real, live commercial customer need. And so we began in the same way, going down the stack, understanding what the computation needs were from the software perspective before we even started down the path on architecture and hardware.”
Foer those who are looking to bet on a new architecture, Liang offers some good advice, which you will be able to hear if you listen to the presentation. And frankly, it applies equally well to any of the major transitions we have seen so far in the datacenter over more than five decades of history, and that advice will apply equally well to the many transitions we will see in the next five decades. So have a listen.
Be the first to comment