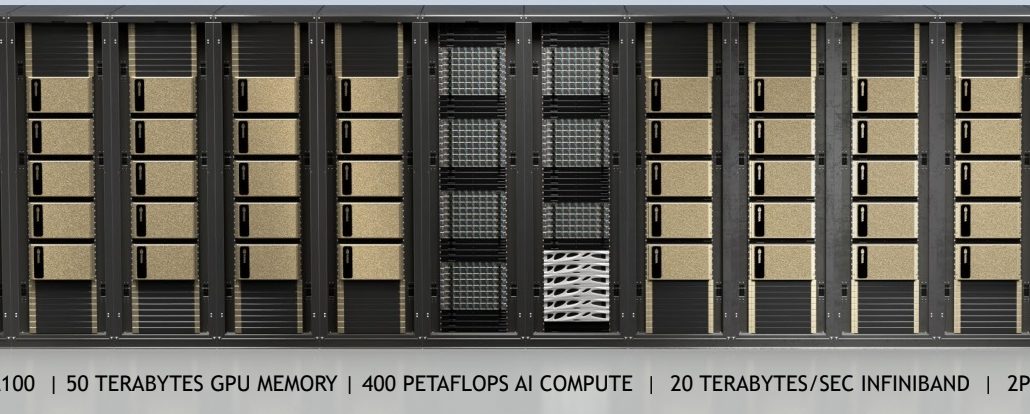
Targeting HPC, AI, Analytics trifecta: A100 GPUs get double the memory with 80GB HBM2e for server, DGX, and appliances while Nvidia/Mellanox announce NDR 400G Infiniband.
Nvidia has outpaced itself with so many new GPUs for large-scale computing over the last several years that the strategy now seems to be to leave some near-term capability aside to allow big releases at the expected time of year. In today’s case that timing is around the annual Supercomputing Conference (SC20) and while there is not something entirely new to marvel at GPU-wise, there is definite doubling of capacity and capability.
The GPU maker announced that its A100 GPUs are capable of literally double the memory and performance with the addition of 80GB HBM2e devices, already shipped to some of its biggest HPC “Superpod” and in their DGX systems with wider availability via their partner network beginning in January 2021. For those partners, the overhead is simple, the capability and capacity jump adds another option without any overhead for the 400W of the 40GB GPUs and for the early Superpod customers, it’s a simple tray shift, according to Paresh Kharya, Senior Director of Product Management at Nvidia.
Having something new to announce is one thing, but it is probably more likely that without some delays, the original A100 might have had the 80GB of memory already. There’s also upward mobility to 96GB in theory with all six (8GB per stack, only five active in the original A100) controllers activated if they went four memory chips high for the next iterations on the A100 for even larger models, but we’ll stick to what’s been announced today.
Jumping to 2TB/s is a big deal to keep the GPU fed and the ability to finally partition GPUs into seven isolated instances (via multi-instance GPU, or “MIG”) adds utilization and memory bandwidth together for quite an improvement in both traditional HPC simulation and large model-driven AI training. On that note, all precisions relevant to Nvidia’s total view of AI supercomputing, which encompasses modeling and simulation, data analytics, and deep learning are part of the package along with various optimizations Nvidia says it’s made to both hardware and software with this new doubling of all elements.
Kharya says that this combination, especially with 600Gb/s interconnect bandwidth between GPUs enables efficient scaling and across all the improvements, they’re seeing 1.8X faster runs for traditional simulations than the 40GB A100, 3X faster training for recommender systems on a 450GB dataset, all the while showing impressive results on the Green 500, although the results aren’t out quite yet. “The HGX AI platform is a single, unified platform for converged simulation, analytics, and AI, scalable from single server to exascale,” Kharya adds. “It all starts with the guts behind the DGX with the software level including support for multi-node GPU.”
Speaking of the DGX, as one might imagine, there is a new one soon available with the new A100 80GB variant with a total of 640GB. This, as one might imagine, will be an AI training beast in particular, allowing far larger models, thus greater efficiency and performance. While there is no pricing released since Nvidia leaves this detail to its partners to arrange, we can guess the addition of Samsung’s HBMe2 probably does jack the price up a bit beyond the other improvements.
Charlie Boyle, VP and GM of the DGX line says that the new DGX featuring the doubled-down A100s have been in testing and production inside Nvidia’s own datacenters for some time alongside select Superpod customer sites where they have been installed.
“We doubled everything to make it more effective, from GPU memory, DRAM, and storage along with impressive 200GB networking. We cut the price of analytics in half because there it’s all about capacity. And for those who want a lot of users on the same system, the MIG tech just got twice as useful.” He highlights the modularity, adding that for those who already had A100 40-based systems, upgrading to the new spec is a tray change and field updates and they’re up and running. Nvidia hasn’t been just selling a couple of these systems at a time, it’s usually large clusters, moving to Superpods, Boyle explains (which can be a cluster of 20-140 systems in multiples of 140 after that). “This is the fastest way to get AI into the datacenter with our recipe and support,” he says.
This leads to another announcement along those same lines. For those who are still in the toe-dipping phase of on-prem AI infrastructure there’s now a workstation featuring the A100-80GB. Normally we don’t cover much on this side of things but this, like its lesser-memory counterpart, provides a way to develop and test the exact same server chip that might eventually be in the datacenter in a format that plugs into a standard wall socket (which apparently isn’t as bad an idea as it sounds). With the MIG partitioning, an entire HPC or AI team can use the machine in isolated pockets and truly test the viability of an A100 with now third-generation TensorCore, NvLink, and tons of memory for big models. Take a look below:
On another note, despite the transition not so long ago, the Mellanox folks, now fully integrated into Nvidia, have released much-anticipated NDR 400GB Infiniband, which we’ll discuss in a different, focused deep dive today.
“The most important work of our customers is based on AI and increasingly complex applications that demand faster, smarter, more scalable networks. The Nvidia Mellanox 400G InfiniBand’s massive throughput and smart acceleration engines let HPC, AI and hyperscale cloud infrastructure achieve unmatched performance with less cost and complexity,” Gilad Shainer, senior VP of network at Nvidia said of the seventh generation of InfiniBand released today. NDR 400G Infiniband provides 3X the switch port density, he added, noting that it boosts switch system aggregated bi-directional throughput by 5X up to 1.64 petabits/second, which makes larger workloads possible.
While all of the announcements from Nvidia for SC20 represent incremental improvements on existing technologies, there is little doubt that GPUs are on the rise, fed by the convergence of HPC, large-scale analytics, and increasingly, AI a burgeoning part of some scientific workflows.
“AI supercomputing requires much more than just raw FLOPs,” Kharya says. “It requires an end to end platform that accelerates end to end workflows for simulation, data analytics, and AI and a unified platform with the full hardware and software stack.”
The A100 80GB GPU is a key element in that HGX AI supercomputing platform which brings together the full power of Nvidia GPUs, Nvidia NVLink, Nvidia InfiniBand networking and a fully optimized AI and HPC software stack to provide the highest application performance. It enables researchers and scientists to combine HPC, data analytics and deep learning computing methods to advance scientific progress,” Kharya concludes. And break out your leather jackets and spatulas, there will be more detail about all of these announcements today during the company’s special address at SC20 at 3:00 Pacific today.
Be the first to comment