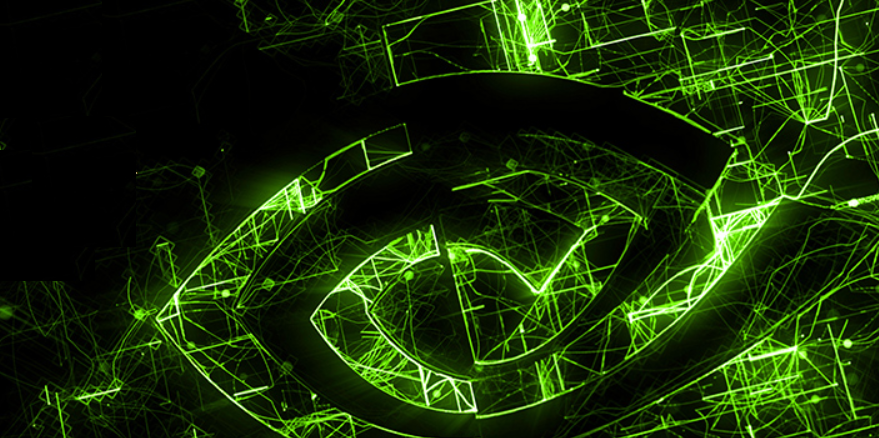
The datacenter business at Nvidia hit a rough patch a little less than a year ago, but it is starting to pick up again after a few quarters of declines and may soon be in positive growth territory. The addition of InfiniBand and Ethernet networking company to the Nvidia fold will eventually help lift that datacenter business a bit more, when that $6.9 billion deal announced back in March finally gets approved by government regulators.
In documents filed with the US Securities and Exchange Commission going over the numbers for Nvidia’s third quarter of fiscal 2020 ended October 27, the company said that it was still in discussions with regulators in the European Union and China and that it now seemed likely that the Mellanox closing will happen in early 2020 rather than by the end of the 2019. (Those are references to calendar, not fiscal, years.) The good news for Nvidia is that while its revenues have been down across most of its product lines in recent quarters, it has still been throwing off profits and now, after eight months, has more than enough cash now to do the Mellanox deal. This is one good side effect of having the regulatory approvals drag on. Nvidia finished fiscal Q3 with $9.77 billion in cash and investments and $7.78 billion net of total debt, so this deal is not even a stretch.
It is, however, still the largest investment that Nvidia has ever made in its history. Mellanox made similar big bets in its past, and it is possible that in the coming years Nvidia will have a taste for other technologies. An FPGA acquisition might be in the cards, for instance. We suspect that Nvidia will not buy a CPU provider, but it is possible that it could create a server-class RISC-V chip to round out its compute portfolio. Why not be the first? No one has yet emerged with a server-class RISC-V design, and if Nvidia wants to win exascale-class deals and have all of the trickle down effects into the enterprise and across to the hyperscalers and cloud builders, why not?
Back in reality, we expect that Nvidia will start talking about its plans for Mellanox when co-founder and chief executive officer Jensen Huang hosts an event at the SC19 supercomputing conference next week, but with the Mellanox deal not done and the companies still legally required to operate independently, there is only so much that Huang can do, much less talk about.
In the fiscal third quarter, Nvidia posted sales of $3.01 billion, down 5.2 percent. Gross profits were essentially flat year on year, but increasing research, development, sales, and marketing costs pushed net income down by 26.9 percent to $899 million. By the way, that net income is still 30 percent of revenues, which is about where it was averaging in fiscal 2018 and although not as high as the average in fiscal 2019, Nvidia is far more profitable, in terms of net income as percent of revenue, than it has been at any other time in its history excepting the bumper year in fiscal 2019. Since 2011, which is where we started tracking it as Nvidia took off in HPC and was poised to take off in machine learning, the average net income per quarter ranged from 10 percent to 20 percent of revenue – and Nvidia was three times as small at that.
This has been nothing short of an amazing transformation from a company that supplies GPUs for games and workstations to a company that provides compute platforms that span from the edge to the datacenter. The datacenter business has been a big part of that transformation, as has the never-ending appetites for better graphics among gamers, which creates the virtuous cycle that Nvidia has been riding. The interesting bit is that starting with the “Volta” GPU generation, the HPC and AI workloads got all of the features first – notably Tensor Core math units – and then only after a while was Nvidia able to tweak these to create the AI-assisted ray tracing units that are part of its GeForce and Quadro GPU cards and Tesla T4 compute engines. Nvidia moved up from the bottom, and now its technology is cascading down from the top – as it should be.
Forget the year on year compares in the datacenter business for a minute, which don’t look great, and just look at the absolute revenues in the chart above. Remember, now, that Nvidia is dependent largely on HPC centers, hyperscalers, and cloud builders for the revenues in the Datacenter group, and remember also that these three markets have huge and capricious spending swings. That the revenue line for the Datacenter group doesn’t look more like the EKG of a cornered animal is pretty good. Supercomputer makers Cray and SGI, for instance, rarely had such a smooth curve because they were so dependent on government and academic budget cycles and processor cycles from Intel and sometimes AMD.
That said, Wall Street looks at year-on-year compares and had been expecting somewhere around $760 million in datacenter product sales at Nvidia in the current quarter, and that did not happen. Rather, Datacenter group posted an 8.3 percent decline, to $726 million. Revenues were up 11 percent sequentially, and Huang said on the call with Wall Street analysts going over the numbers that for the first time in its history, the Tesla T4 GPU accelerators outshipped their Tesla V100 older and bigger sibling. Most of those Tesla T4s, Huang added, were being adopted for inference workloads, and as we have shown in the past, these are among the best price/performers when it comes to image recognition workloads. Inference is now a “double digit” share of Datacenter group revenues – call it somewhere between $75 million and $100 million is our guess – and the business, explained Huang, had “doubled year over year.” (Presumably Huang meant both shipments as well as revenues in this reference, since the Tesla T4 product line has not changed in the past year).
We are not surprised that there has been a bit of softness, relatively speaking, for the Tesla V100 GPU accelerators. This time last year, IBM was working with Nvidia to build out the “Summit” hybrid supercomputer at Oak Ridge National Laboratory and the “Sierra” supercomputer at Lawrence Livermore National Laboratory, and these had a combined 44,928 Tesla V100s. Summit and Sierra were pre-exascale systems and no one is building an exascale box right now and won’t start until 2021 and 2022, depending on the deal and we don’t even know if Nvidia is going to get any piece of that action. Moreover, everyone expects for Nvidia to unveil a 7 nanometer kicker to the current Volta architecture or a full-blown “Ampere” sometime in 2020, so that is a probably contributing factor to the flattening of datacenter sales in recent quarters, too. This time last year, the hyperscalers and cloud builders were on a server binge, and they were buying GPUs in droves to build up their machine learning training and HPC capabilities. Additionally, Nvidia had a large DGX system deal. And, we think Nvidia has been dropping prices on Tesla V100s because if sales of V100s are at record highs, as Huang had said, then the revenue should have gone up more than it did.
This is pretty much the definition of a tough compare. But that is just the nature of the upper echelon of the computing business. And everyone here trying to build the next platform at scale is used to that, even if Wall Street has unreasonable expectations. That’s their problem, not Nvidia’s.
What Nvidia is doing is capturing the demand by the hyperscalers to build AI-assisted recommendation engines and to bring conversational AI capabilities into their applications, something that we have discussed as the next big opportunity for GPU training and inference. As Nvidia has explained, conversational AI, brining human-like natural language processing to applications, is pushing Tesla V100 sales for training because these models have 10X, 20X, or sometimes 100X the number of parameters that to build the neural network than were needed to do image recognition. (Accents and vocabulary differences are harder to process than lighting and angle differences, we suppose.)
Moreover, the inference part of conversational AI has more neural networks as well as denser ones, which requires lots of compute, as well as a need to get it all processed – taking out the noise, recognizing the speech, figuring out the response in text, and converting that text to computerized speech – has to be done in under 30 milliseconds. This is what is driving Tesla T4 sales, according to Nvidia, and the company claims further that this low latency requirement absolutely necessitates acceleration compared to running it on CPUs. This was not the case with image recognition, where the inference was largely done on CPUs, with some GPU and FPGA exceptions here and there where organizations really wanted to push the performance and price/performance envelope.
“Just as AlexNet seven years ago was the watershed event for a lot of computer vision-oriented AI work, now the Transformer-based natural language understanding model and the work that Google did with BERT is a watershed event also for natural language processing,” explained Huang on the call. “This is, of course, a much, much harder problem. And so the scale of the training has grown tremendously. I think what we’re going to see this year is a fair number of very sizable installations of GPU systems to do this training.”
Looking ahead to the fourth quarter of fiscal 2020, which will end in January, Nvidia said that it expects for sales to be around $2.95 billion, plus or minus 2 percent, which is 34 percent growth at the midpoint of that range. Nvidia is expecting strong sequential growth in the Datacenter group, but a decline in notebook and gaming GPUs. None of this includes any revenue contribution from Mellanox.
Mellanox does not say much about its financials these days, and certainly not with the granularity that it used to provide, giving us a sense of InfiniBand transitions as well as its ramp of Ethernet products at the hyperscalers and cloud builders.
What we do know is that revenues in the calendar third quarter ended in September rose by 20.1 percent to $335.4 million and that net income was up by 19.2 percent to $44.2 million. Mellanox exited the quarter with $742 million in the bank, which is a third again as big as the cash pile was at Mellanox when the deal was announced, which helps cover some of the cost of the acquisition. By the time the Mellanox deal closes, there is a pretty good chance that it might have $1 billion in the bank if the 200 Gb/sec HDR InfiniBand cycle starts taking off in earnest and the hyperscalers and cloud builders resume their spending and lift the Ethernet adapter card sales at Mellanox accordingly.
I have no issue with NVDA MLNX acquisition, except, MLNX did not need to financially on operating enterprise, someone must want to retire on this acquisition exit strategy?
To the extent MLNX on which the entire industry relies as equal arbitrator there were other, merger potentials, those retiring can at least exit with a clear conscious.
Kept on, likely, David forbid, sent to the pits as design slaves for the foreseeable future.
R&D innovation on NVDA investment acceleration? MLNX is self funding.
I would have recommended a bond issue. In a very short period, like before you knew, the entire issue would have sold out.
Most recent NVDA GPU channel data here;
https://seekingalpha.com/instablog/5030701-mike-bruzzone/5360948-gpu-channel-inventory-today
This week RTX v GTX channel holdings are 28.7% and 71.2% respectively GTX builds. In last four week Quadro GP/P channel inventory increase 1580% attached to back in time Xeon workstations similar RTX at 6K intro exclusively attached to v4/v3 back in time workstation that’s more than enough control plane processing for this application.
Mike Bruzzone, Camp Marketing