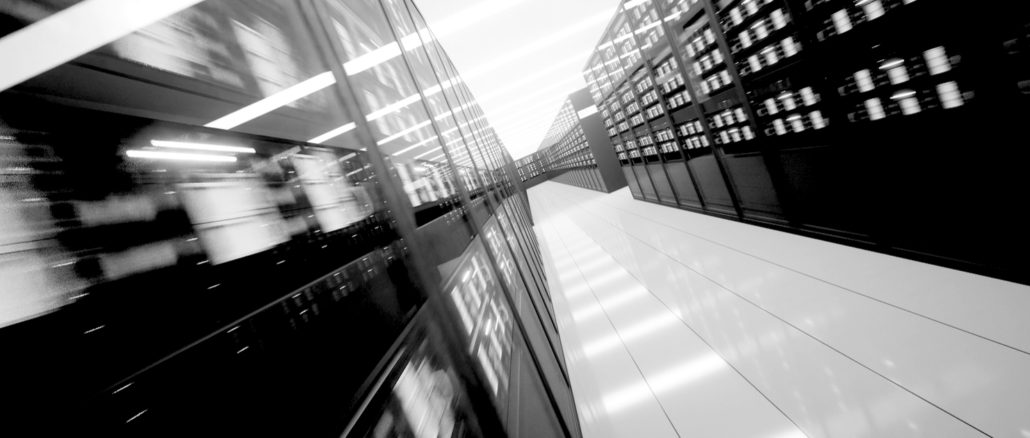
Storage is no longer an afterthought when it comes to systems of any reasonable scale.
This is not to say significant thought has not gone into various storage architectures for HPC and large-scale enterprise but the options are expanding.
Now, with AI and its latency and bandwidth requirements added to the mix, storage is more diverse than its been for the last decade. All-flash arrays, advances in NVMe over fabrics, new protocols and data movement innovations to keep accelerators fed, and a rethink of traditional parallel file systems are all pushing storage in new directions.
Despite all the freshness in terms of approaches and technologies, there are still questions about where to start that hinge on the workloads at hand. While there are plenty of sites that might just have a dedicated AI-driven cluster, many are doing AI in conjunction with simulation, analytics, and other workloads on the same machine with resources that have been carved off a much bigger system.
This brave new world for storage and I/O was given a dedicated segment at The Next AI Platform event on May 9 in San Jose. We brought together experts across this diversifying arena to share perspectives on what is new and, in some cases, what has long been established but has had to pivot to match changes in what people need from storage systems.
Among those interviewed live on stage was Curtis Anderson, a lead architect at Panasas, which was one of the first companies to capture the shift from monolithic supercomputers to the scale-out era that very quickly followed. In 1999 Panasas built a robust file system for HPC and has since used that expertise to capture attention in AI given so much shared momentum and convergence between HPC and AI.
Anderson talks about this transition over the last twenty years and what that shift from monolithic supercomputers to parallelized architectures meant then, and how that story arc reflects what is happening today.
We have driven home over the last few years the convergences of HPC and AI technologies and there are good reasons to think about this further in the storage context, as Anderson explains well.
“We are using HPC history as an example of the story arc and using that logic to predict what the future of the AI market will be from a storage perspective.”
“The HPC definition of good performance is total wall clock time to result, which includes I/O wait time. So the engineers at the time customized their software to minimize those I/O wait times. They built their software knowing what the parallel file systems of the day could achieve. But AI workloads are different; it’s not possible to put all the data into one file and stream it,” Anderson explains.
He adds, “There is an element of enforced randomness; you can’t reprocess things in the same order or you overtrain a mode. And that randomness is where the low latency requirement comes from. Further, GPUs are expensive and you don’t want those to go idle. That’s the biggest difference between HPC storage subsystems and something tuned for AI.”
“It is our belief that as enterprises adopt AI they will try it out; they will have many different neural network frameworks they want to try, all operating on different time scales, which looks like a randomized workload. It’s not just the training set that’s randomized, but the different datasets coming and going over time. A team might tweak a model and then do runs on a training dataset, for instance, and that is not something that you can tune for.”
If Anderson is correct and AI evolves along a similar trajectory as HPC did over the years we will see a shift away from the big singular systems for AI training and more distributed machines doing AI training, inference, general analytics, and a range of other workloads. This diversity and lack of tuning of storage means having a flexible system from the beginning that can handle large and small files equally well and have the intelligence built in to understand what compute elements need to be served over others as conditions change.
This is no easy task and as we heard during the event, there are multiple ways of solving these problems from a storage and I/O perspective. Stay tuned for more interviews that cover various perspectives on this topic filmed during the Q&A based Next AI Platform event.
Be the first to comment