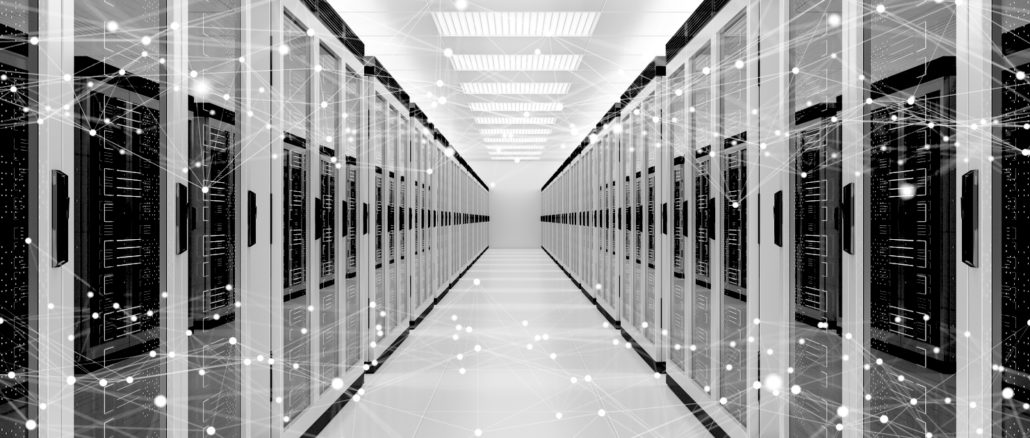
Disaggregated storage has become the norm in large-scale infrastructure and we expect that in 2019 those who are pushing the limits with NVMe will have quite a successful year—vendors as well as their hyperscale and high performance computing users.
Before we look at how this is going to play out, however, let’s take a step back and look at what has pushed this trend, beginning with the first NVMe products in the 2014 timeframe when there was no slim protocol, just a driver running on a server that had to have control of the storage to access it.
Standalone NVMe was a game-changer for some workloads with its direct connections to non-volatile storage but the real story is just beginning to play out with the rapid adoption of NVMe over fabrics. Before that happened, however, hyperscale companies, along with some high performance computing shops in traditional HPC or financial services, for example, rushed out to invest in NVMe on selective nodes. In other words, NVMe was done on a per-node basis, which offered little in the way of flexibility and left a lot of performance on table.
At that time, most servers had loads of disk and a couple of flash drives with some NVMe ports were loaded to varying degrees depending on budget and workload. And there is a reason we say “budget” first since NVMe added a significant premium in 2014—up to 50% in some cases.
The point was, most users could not realistically put NVMe in every server, instead reserving it for special workloads that were both bandwidth and latency sensitive. For example, there might be two to four ports out of 24 or 36 what would have NVMe. And while the early results of this boost were promising, nothing could change the fact that all of those NVMe ports with the flash sat behind a disk controller, which cut down on the available bandwidth from flash. And flash was not the problem, the network connecting the processor to it was.
But flash forward to now with NVMe over fabrics and dramatic reductions in the cost of NVMe and we can begin to answer the question of why companies that maximize NVMe are in for a hot year. Especially since the users they are catering to who invested big in NVMe now have a better way to get far more utilization three or four years after that expensive move.
In an era where it is possible to get 100GB/s or (later this year) 400GB/s, the bandwidth explodes and putting storage in one place and making it look local in terms of latency is possible. And therein lies the hook to what Mellanox, Pure Storage, and startups like Excelero are doing. They are pushing thin ways to pool resources and make remote storage look local with the ability to configure it on the fly according to what each server needs.
Of course, the difference is in how all of this is done—and there are subtle differences in how those example companies tackle the job. But the end result is essentially the same, disaggregating storage is possible without penalty—and those who made investments in NVMe can finally start to see some serious benefit now that it storage is streamlined. And this opens the door to much larger clusters that can do more with less—and even allows for specialized clusters like the DGX boxes from Nvidia, for instance, to truly live up to their AI training promise.
But enough background, let’s put this in real-world context.
And there are numbers on this coming growth but the real story is that we know the costs of NVMe have dropped but the I/O challenges will keep mounting.
We’ve established that the per-node approach to NVMe at the beginning of the boom happened at hyperscale and in certain areas of HPC, including financial services. Big storage hardware investments were made for key workloads that were bandwidth and latency sensitive and while there was some early payoff, as noted above, the utilization and performance was still stymied by how this was all engineered.
For instance, as Josh Goldenhar from Excelero (deep dives on their approach to NVMe/fabrics and how it differs from things like Mellanox Bluefield or Pure, NetApp and others), which specializes in the similar kind of NVMe over fabrics work that has pushed Pure Storage into the limelight (along with Mellanox’s Bluefield as a variation), tells us, one of their largest hyperscale users (they can’t go on record but we’ve confirmed) and one of their smaller hedge fund users both had the same basic problem. They climbed on the NVMe bandwagon early on with per-node implementations and, given the rise in demand from AI and beefier analytics workloads, have had to find a way to pool resources to get tolerable utilization and better performance out of that investment.
“HPC and the hyperscalers are closely intertwined but have different methods but generally, they both need low latency and high bandwidth and they both tended to deploy NVMe locally on a per-node basis.” Goldenhar explains.
“Our largest hyperscaler and a smaller hedge fund with a large AMD Epyc clyster both deployed NVMe this way and they have the same problem. Their utilization was very poor because when they put it per host, it meant someone had to decide what size drive each host should have. Neither wanted to err on the side of going too small, so they both went larger than what they needed to be prepared for the next three years, and they were getting between 15-20% utilization because each host needed something different; some would use 90% of the drive, some close to 0%.
The cost of NVMe has come down significantly, of course, which means the opportunities to bring up that utilization at scale will keep cropping up. And it bears repeating that the cost considerations are one crucial difference between these two users. Hyperscale companies can buy NVMe for about the same price as SATA but even for others the price differential has narrowed and the benefits of bandwidth that comes from NVMe over SATA (around 500MB/s versus 3GB/s) make NVMe hotter than ever.
Goldenhar says that NVMe over fabrics was somewhat esoteric even at Excelero’s launch but last year at the Flash Memory Summit the tides shifted and suddenly the interest exploded. While Facebook and others have been doing the same thing with their disaggregation of storage before this peak of interest, for the lower-tier hyperscale companies, this is was a revolutionary concept. It took hold in these markets as well as at big banks with large problems that were continually I/O starved.
“What really started to push interest was there were more options on the market. Traditional players like Pure Storage and NetApp started to support NVME over fabrics and once you have players that size pushing things that support this protocol, then the next challenge is in overcoming the problems with the traditional storage architectures they have,” Goldenhar argues. “The protocol by itself for limited transactions is great—the latency is dramatically reduced but the traditional controller design becomes the bottleneck.” To this end Excelero has started supporting TCP in beta with some customers but he says that generally, “2019 is the year we will see more adoption in more places and using NVMe over the network is gaining acceptance and will flourish this year.”
It is hard to disagree with this. Consider the challenges big banks are facing.
One of their first large financial production customers, a major European bank, is running vanilla SAS analytics but they found that in different passes on a certain key dataset the traditional hardware was far too slow for them to comply with EU regulations for daily reports that were taking 40 hours to process. They could not just deploy local NVMe because it was based on a SAS Grid cluster built on top of GPFS. In other words, there might be eight nodes working on the same dataset at one time, which means it wasn’t possible to stuff as much NVMe in because the dataset couldn’t be split. This was both a latency and bandwidth sensitive workload—high bandwidth when the database did its full index scan of all the day’s data then with very high random I/O and smaller reads as it checked individual records. “The combination of NVMe that can handle both demands and our software allowed them to use shared pools under GPFS and all the GPFS servers and the hosts could quickly get to the block devices that underly everything Excelero does. This cut latency for small reads and bandwidth was only limited by the network, which brought processing time for these reports down to around six hours.
This is not to cheer a vendor so much as to show that the one big problem in many HPC shops in particular, which is chewing on mixed workloads, is being solve this way and maximizing previous investments in NVMe. The other takeaway here is that by using something like the underlying block storage, a bulky parallel file system like GPFS can ride on top and offload the things it is not good at (namely small/random I/O). Lustre, as a side note, is not as shiny of an example of a parallel file system getting a new life, if you’re curious why we can provide a comment answer.
The other point to the disaggregated storage story is that previously, taking a massive dataset and copying it to every single host is crazy overhead with multi-terabyte drives. Everything goes over the network and even with high-speed networks, that is sending tons of data across the network to do a compute job only to delete it and move on. Centralized storage just makes sense. And this year we expect that lesson to catch on, no matter how that happens—and there are an increasing array of options from major storage vendors to small startups alike.
A more detailed look at how this works can be found in a similar use case where a company called CMA created its own baby version of an Oracle Exadata cluster for using legacy databases while maximizing NVMe investments. Again, this is not to push what this one company is doing in this space, but to show why this trend will kick in this year.
And here’s what is really interesting. Just like it is possible to pool together to created a massive shared memory pool similar to enterprise appliances like an Exadata machine, for instance, the DGX systems from Nvidia are also getting the disaggregated storage treatment, which allows the kind of networking that can make multiple DGX-1 systems truly hum.
Goldenhar says that in finance in particular there are folks with several DGX-1 appliances chewing on a single workload. “There are people with farms of DGX-1s and the problem here is that Nvidia only put a single bi-8 interface to the SAS chip to drive the SATA flash inside. But at the same time they put about a 4GB/s interface to all the SATA flash you can put in there and they loaded it with 100GB interfaces. So you can get much more bandwidth and lower latency over SATA by accessing NVMe flash outside the DGX-1 than you can by accessing the SATA flash inside.” He says the DGX-2 machines, which have NVMe inside are still limited by that amount and can only use what is inside the box. Using an approach like they have it is possible to have all of those DGX-2s with NVMe and pool all of that to allow any of those machines to access any of the memory without using the CPU inside, or as he calls it, “logical disaggregation.”
On the subject of AI and its storage angle, Goldenhar says that this workload is beginning to drive serious business on the NVMe side, particularly with an over-fabrics hook. Outside of the DGX appliances, users in two key areas—those doing algorithmic backtesting and the new use cases in medical imaging and GIS have big benefits to gain from AI but are hitting bandwidth and latency limitations because of the size of images for training in particular.
He says that benchmarks like ResNet are using tons of small images to help users get a sense of relative performance but in the real production world, training images are massive.
“The resolution of images from MRI or satellites can be in the several terabyte range. If you’re looking at high resolution images of potential skin cancer or you’re a government agency looking at satellite images to detect builds or launches you need low latency and high bandwidth for training. There is a great deal of pressure to take in datasets that are not only larger in count but in the individual elements being analyzed,” he says. “As a lot of these methods get applied and people move from testing to putting things into production we will see not just more NVMe in general, but the need to share it.”
“Imagine an entire database of transaction and account records. It’s not possible to copy that and keep it up to date for every single compute host. They need to scale the GPU cluster to handle real-time queries but do that against a centralized resource so they can accumulate enough capacity to do a random access against it. So when you are in the tens of terabytes it’s not possible to do that on every host without a centralized resource that can be updated and instantly available to every host. Even when these are in different regions or countries.”
The short answer to why NVMe will flash forward, in other words, is that for demanding workloads, companies at scale are rethinking their infrastructure from the ground up. This is definitely true in storage since I/O is the bottleneck in many cases. But it’s happening at the system level as well.
As we are going to argue in live interviews in May, AI is driving a lot of the changes that are going to hit traditional systems makers starting in earnest this year. The rise of NVMe over fabrics is just one aspect of the bigger picture. From experimentation with general purpose and custom accelerators for certain workloads (and all the complexity that will introduce), to actual options beyond traditional X86 and Intel, to new control frameworks to allow infrastructure to continue its move beyond the brick and mortar, down to how applications are developed and packaged to move seamlessly, the whole stack is changing.
It’s a good time to be called The Next Platform.
Ok, I’ll bite: what about Lustre?
Also, how do you turn a block interface like NVMe into a cluster filesystem?
A couple of comments/clarifications:
1) AFIK, Pure Storage does not have front end NVMe, they only have NVMe drives. As you know, the performance difference is on the front end and only a small improvement can be made on the drives alone.
2) Persistent Memory on servers (which can be pooled with NVMe external storage) with the new Intel Cascade Lake servers will provide single digit latency, the absolute fastest, lowest latency NVMe data access possible. Low latency applications (like high-transaction banking applications) will all be gravitating to this if performance is the primary factor.
1. My favorite solution was that one delivered to NASA Advanced Supercomputing Center (https://www.excelero.com/wp-content/uploads/2018/04/NASA-Ames-Customer-Profile.pdf)
2. What they did with NVME, that “fabric” could be applied to RAM disks, ie allow a cluster of servers to amass local ram disk in a huge fast drive
This is a good article but anyone considering NVMe disaggregation should take a close look at NVMe over RoCE and NVMe over TCP and figure out which is best for their needs. In our experience (http://www.lightbitslabs.com) NVMe/TCP is best for nearly all deployments, and now that it is standardized and upstream drivers exist, it’s a breeze to deploy with Lightbits’ high-performance, consistently low latency NVMe/TCP target.