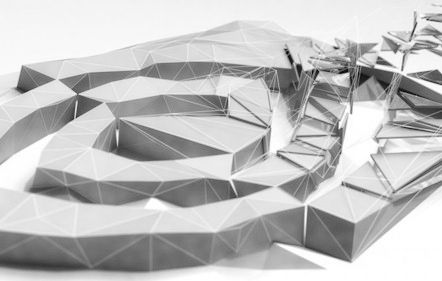
Nvidia co-founder and chief executive officer, Jensen Huang, would be the first one to tell you that the graphics chip maker was an unintended innovator in supercomputing, that what the engineers who created the first Nvidia GPUs were really trying to do was enable 3D video games.
But by making GPU cards more programmable for graphics and by adding 32-bit floating point math to help do that, Nvidia stumbled upon a new market: HPC. And from there, the Tesla GPU accelerator business ballooned, the GPUs got more complex and powerful, CUDA and OpenACC made them more programmable still, and now it is the engine of choice for machine learning, too. The Tesla GPU accelerator has become the compute engine of choice for high-end HPC and machine learning.
These two markets formed in front of Nvidia separately, but now, many think that HPC and AI are converging, or at the very least mashing up, and that was a big topic of conversation in the interview that The Next Platform did with Ian Buck, general manager of accelerated computing at Nvidia, during the SC18 supercomputing conference in Dallas. What Buck sees happening now is simulations being used as inputs to machine learning models, and to perform what is called AI preconditioning to actually reach into algorithms and figure out where the heaviest math will be needed to do the most important parts of a simulation. (This particular work that Buck refers to was done by researchers from the University of Tokyo to speedup earthquake simulations by a factor of 25X when running on the “Summit” supercomputer at Oak Ridge National Laboratory, and the success of this AI preconditioning approach made it one of the six Gordon Bell Prize finalists for 2018.)
One of the big questions is what will HPC centers do when they want to train machine learning models and then add inference capabilities to their simulations. It could turn out that they will take the approach of hyperscalers and cloud builders, breaking machine learning training (which can be run on the same machinery as GPU accelerated HPC systems) from the machine learning inference that is used in applications. Interestingly, both the high-end “Volta” GPUs and the low end “Turing” GPUs can do both training and inference, but the former is really geared for HPC and machine learning training and the latter for inference and graphics rendering with real-time ray tracing enhanced by inference. But researchers – including Buck himself when he was at Stanford University back in 2000 – have a habit of making computing devices do what they want, not what they were initially designed for.
The real question now is what will HPC centers do as they experiment with machine learning and then try to put it into production. Will they play around in the cloud, and then bring AI back into their own datacenters, or will they just buy machinery that can do both HPC and AI and be done with it. Buck tells a what he thinks about this, and how Nvidia will architect for the future with both workloads in mind.
Be the first to comment