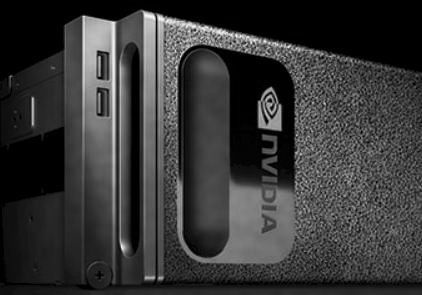
A shared appetite for high performance computing hardware and frameworks is pushing both supercomputing and deep learning into the same territory. This has been happening in earnest over the last year, and while most efforts have been confined to software and applications research, some supercomputer centers are spinning out new machines dedicated exclusively to deep learning.
When it comes to such supercomputing sites on the bleeding edge, Japan’s RIKEN Advanced Institute for Computational Science is at the top of the list. The center’s Fujitsu-built K Computer is the seventh fastest machine on the planet according to the Top 500 rankings of the world’s fastest systems, and the forthcoming post-K super, which will swap SPARC for ARM, is set to go online in 2020, marking what some believe could be the first truly exascale-class machine.
While teams at RIKEN have already been working on getting their supercomputers to think in lower precision for both simulations and machine learning workloads (as we will detail in an article featuring Tokyo Tech/RIKEN’s Satoshi Matsuoka later this week), teams at the Japanese research hub are pushing deep learning and AI faster with the addition of a new, more purpose-built machine slated to turn on at RIKEN’s Yokohama facility in April.
Just one year after its founding in 2016, the RIKEN Center for Advanced Intelligence Projects will be among the first to have a purpose-built AI supercomputer. This machine will have 24 DGX-1 server nodes, each with eight Pascal P100 GPUs to complement a larger complex of 32 Fujitsu PRIMERGY RX2530 M2 servers. While this is by no means the largest deployment of the DGX-1 boxes (Nvidia itself made the Top 500 rankings with its 124-node system outfitted with the latest Pascal generation P100 GPUs) it will be interesting to see how RIKEN blends current HPC and new deep learning frameworks for current and future applications. The system will be capable of four petaflops of half-precision performance—an unfamiliar metric for those used to gauging supercomputing systems, which are measured on their double-precision capabilities. Fujitsu and RIKEN have worked together to bolster the software stack in addition to the deep learning frameworks that are already optimized and ship with the DGX-1 appliances.
Some back of napkin math on this machine reveals an interesting split of compute with, you guessed it, the beefiest portion on the DGX-1 side. With a projected list price of $129,000 per DGX-1 node and, let’s conjecture $10,000 for the 32 PRIMERGY nodes, the machine all ready and configured is around $4 million. This is a small system by supercomputing standards, but if it is able to eventually chew on some HPC workloads at lower precision eventually, it could bolster the case for 8-GPU boxes handling a mixed machine learning/simulation workload—something that we hear is gaining steam at some centers, at least in research circles.
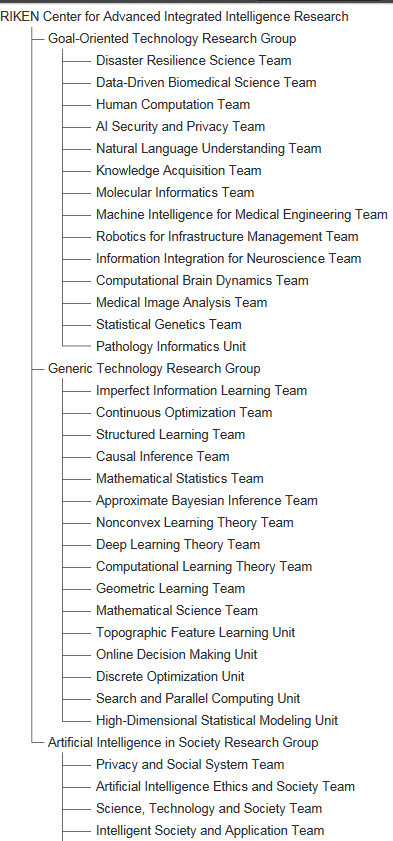
One of the challenges that Nvidia solved with its own 124-node DGX-1 supercomputer was the multi-GPU scaling issue, which has plagued early users of deep learning at scale. MPI-based efforts are still developing and being released into the open source ecosystem, which will allow easier building and use of densely packed GPU-based machines. The software work to get the hardware to hum has been handled by RIKEN, Fujitsu, and Nvidia teams, but real application scalability will provide some interesting insight.
The Center for Advanced Intelligence Projects is stretching an AI effort across many domains, some of which include traditional supercomputing simulation arenas. Current sub-organizations at the center include those devoted to bioinformatics, natural language processing, medical engineering, neuroscience, mathematics, and beyond, with special groups focusing on security, privacy, and human-AI communications.
RIKEN is already on our watch list due to the upcoming ARM-based systems slated for the future, but until then, he reason RIKEN stands out among its HPC peers is because of the uniqueness of the SPARC architecture, which has been employed on many of its top systems. While this may not always secure the center the top slot on the Top 500 list of supercomputers, the architecture performs well on both the Graph 500 and Green 500 in addition to the newer HPCG benchmark, which offers a more accurate measurement of a supercomputer’s performance on real-world applications. This is all important because teams at the center are willing to look beyond the traditional X86 track for simulation workloads—meaning they might be at the cutting edge for blending HPC and deep learning between these architectures.
RIKEN, and other centers in Japan, including Tokyo Tech, are also on our radar because of research efforts centered on finding ways to use single precision versus double for applications that don’t require it. We expect the new AI-focused center to continue pushing existing applications into lower precision ranges while building new ones based on open source deep learning frameworks.
Be the first to comment