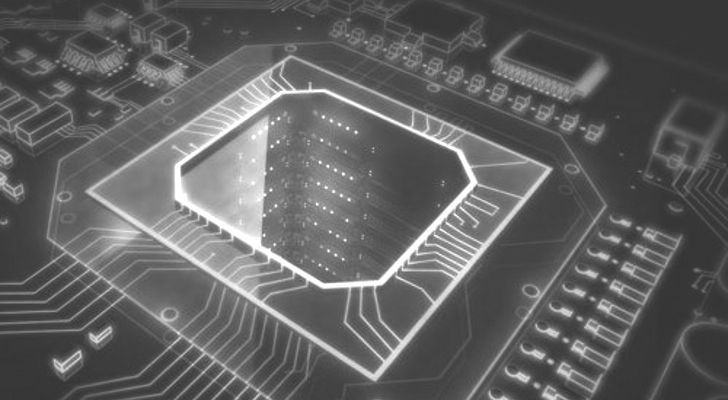
GPU computing has deep roots in supercomputing, but Nvidia is using that springboard to dive head first into the future of deep learning.
This changes the outward-facing focus of the company’s Tesla business from high-end supers to machine learning systems with the expectation that those two formerly distinct areas will find new ways to merge together given the similarity in machine, scalability, and performance requirements. This is not to say that Nvidia is failing the HPC set, but there is a shift in attention from what GPUs can do for Top 500 class machines to what graphics processors can do for AI supercomputers.
The last several annual GPU Technology Conference (GTC) events have focused on Nvidia’s expanding supercomputing presence, beginning in earnest with the launch of the Titan machine at Oak Ridge National Laboratory in 2012, which was the top supercomputer in the world—and the first of its kind in terms of its GPU count. At this year’s GTC, however, we heard far more about slinging together DGX-1 appliances to build AI supercomputers than we did about future Volta-based GPU supercomputers like the forthcoming Summit and Sierra machines.
To be fair, that could be because these machines won’t even be produced until the end of this year, but the point is, HPC has less topical appeal lately than AI. This should come as no surprise as every company with a product that was analytics-focused (hardware or software) is recasting and retuning that product set to have a machine learning angle.
Ian Buck, GM of Nvidia’s Accelerated Computing group tells The Next Platform that while AI indeed captured the most attention this year, there is no pulling back from HPC. In fact, he says, the Volta GPU story is as strong for the supercomputing market as it is for users in the deep learning space. “It has 7.5 teraflops of double precision performance and supports a catalogue of 450 accelerated applications. The top ten HPC applications are all ported to GPU, the last of which was Gaussian, and Volta we be going into the CORAL supercomputers, Summit and Sierra that will be operational as early as next year.”
We have already written about the future challenges and opportunities of a Volta-based machine like the forthcoming Oak Ridge National Lab Summit machine, both from an HPC and deep learning perspective, but for the wide range of national labs and companies where Volta might find a home as an upgrade from K80s or Pascal, what will the addition bring to the table?
According to Satoshi Matsuoka of Tokyo Tech and lead on Japan’s massive Pascal GPU-based AI supercomputer, Volta represents a 41% performance jump, but the real value, at least from an HPC perspective, lies in many smaller, less visible improvements that in sum, make Volta a game-changer for standard HPC applications.
“There are various improvements in Volta, all positive,” Matsuoka tells us, pointing to major advances like unified memory. “Some of these smaller improvements that are actually quite important are the addition of page access counters, which we have been banging on companies to add for a long time. This is hard to do in software without big overhead.” These and other additions are making threading and programming easier, he adds, and while they don’t get front page attention, the many tweaks Nvidia has offered for both HPC and AI tally up to big changes in Volta.
Of course, some have wondered about the less-than-expected performance and memory bumps for Volta. “In terms of raw performance, some codes might get a huge efficiency increase versus just performance,” Matsuoka says. “Pascal became widely available a year ago, Volta is not available until the end of this year, so not even a two year gap and just over a 40% performance increase—from 15 billion transistors to 21 billion and a die size increase, a lot of which will feed into the Tensor Core.” We will talk more in-depth about the Tensor Core this week, but overall, Matsuoka is pleased with progress on the device. And while the memory capacity is less than many expected with Volta (16 GB), he says the current configuration is the only one that would make sense from an architecture, expense, and energy efficiency standpoint.
“If you look at an HBM2 die stack, there are four on each stacked to get to 16 GB. To increase capacity it would be possible to use higher density DRAM or have eight layers, but eight is not cost effective—DRAM is pushing the lithography limits. Stacking more means a power increase and further, decreased yields on those devices…They’ve opted instead to go with 16 GB stacked and to beef up NVlink so it can increase that way and these are lower cost decisions but it means Nvidia can sell ore chips by using slower but cheaper DDR4. There is also a delay here because TSMC couldn’t get its 10 nanometer process efficient so a lot of projects were hit with this as well.”
Jeff Nichols, acting director for the National Center for Computational Sciences at Oak Ridge National Lab tells us it’s important to remember what national labs like his are used to when it comes to new hardware. His teams got used to having 6 GB memory across Titan’s K20 GPUs and now with Volta on Summit, the combination of 20 petaflops on a single machine and the vast improvement in memory over the K20 mark a significant leap in performance and capability.
Looking ahead, while Volta is compelling, another future supercomputer, a contender for top in the world, is built on Intel’s Knights Hill, which is based on true 10 nanometer will be very competitive, not just in terms of floating point capability and flops per watt—at least assuming Intel meets its projected numbers for this. Matsuoka says that single precision—something many labs are shifting to for as many applications as they can—will offer an attractive option on this front as well.
With all of this said from a device level, the HPC and deep learning convergence is an important mesh for traditional supercomputing sites, Buck says. In terms of feedback on the year ahead from a national lab perspective, he explains that centers are seeing that deep learning is one workflow that is being seen as a cornerstone of exascale—a new tool that the nation’s top supercomputers should be taking advantage of. He notes, however, that the generalized frameworks for deep learning are too broad to be applied to many domains in HPC, pointing to work Nvidia and a national lab collective did on the Candle framework—an AI platform to recognize patterns in DNA sequence information to lead to clearer cancer insights.
“What’s exciting for us from an HPC standpoint is that we are building the next AI supercomputer, and from here on, every supercomputer will be an AI supercomputer.” – Ian Buck, GM Accelerated Computing, Nvidia
“Neural network frameworks are not really designed for open science types of problems. For instance, in the Candle example, none of the frameworks had DNA importers. The kinds of neural networks you need are different than image or speech recognition or translation.,” Buck says.
“What the labs do have, however, is the talent to understand how to make this work. Even here, we did not know how to deep learning when we started. We had smart people and we simply trained them; it’s not an impossible thing to learn. It’s data science with a little bit of programming and labs have these people—it’s not hard to learn how to use deep learning frameworks if you’re already a research scientist.”
Be the first to comment