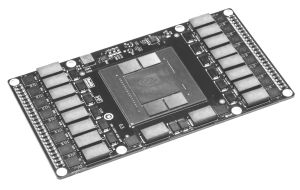
Deep learning could not have developed at the rapid pace it has over the last few years without companion work that has happened on the hardware side in high performance computing. While the applications and requirements for supercomputers versus neural network training are quite different (scalability, programming, etc.) without the rich base of GPU computing, high performance interconnect development, memory, storage, and other benefits from the HPC set, the boom around deep learning would be far quieter.
In the midst of this convergence, Marc Hamilton has watched advancements on the HPC side over the years, beginning in the mid-1990s at Sun, where he spent 16 years, before becoming VP of high performance computing at HP. Now the VP of Solutions Architecture and Engineering, he says that there is indeed a perfect storm of technologies intermixing in both deep learning and HPC—and this bodes well for Nvidia’s future business at both supercomputing sites and deep learning shops alike.
“The reality is that every one of those supercomputing centers is producing data every year, more than they know what to do with, and they have problems they just can’t sole with classic scientific computing and HPC approaches,” Hamilton tells The Next Platform. “The number of people looking at how to apply deep learning to curing cancer or tackling weather prediction with deep learning is growing. What someone does to optimize a deep learning software package is different than what is needed in HPC, but at the end of the day, it’s all matrix math. And that is what we do well; so HPC benefits and machine learning benefits.”
Hamilton pointed to the company’s product line, which now includes an 8 Pascal-generation GPU box with NVLink, quad-rail Infiniband and 7.2 TB of SSDs on each node. Although he says the $129,000 box is targeted at deep learning shops (where they are still limited by multi-node scalability) it is, in fact, an HPC box as well. One of the features of the DGX-1 appliance is there is a cloud gateway to Docker containers with TensorFlow, Caffe, and other deep learning frameworks for quick transport to the system, but there is nothing stopping Nvidia from doing the same containerized HPC application approach on the DGX-1 appliance for HPC. Still, that won’t happen anytime soon. “There are over 400 scientific computing applications in our catalogue but getting those shipped over to Docker and ready for DGX-1 won’t happen right now but it’s in the plan.”
None of this is to suggest that Nvidia will pull back on HPC ambitions in the wake of their very vocal ambitions to own the deep learning training and inference markets—at least on the accelerator front. While deep learning is certainly a very public focus, the company just secured its first large Pascal GPU installation on a forthcoming Cray cluster to be housed at CSCS and we are expecting the announcement of several more Pascal-boosted systems between now and the upcoming International Supercomputing Conference and beyond, although those could very well be OpenPower systems with NVlink and full integration versus the one-to-one hop from CPU to GPU of non-OpenPower boxes.
As we noted during last week’s GPU Technology Conference (GTC), Nvidia is “all-in” when it comes to supporting the needs of machine learning shops, with some dedicated chips and firmed up libraries to support such efforts. There are already the M40 and M4 GPUs aimed specifically at those markets, and now the P100 Pascal cards are also being touted as good solutions at the high end, including inside the company’s new 8-GPU DGX-1 appliances for ready-to-roll deep learning. While indeed, plenty of shops appear to be using Titan X cards in a way Nvidia never intended inside of training servers for deep neural networks (including Baidu among others), the company is serious about pushing its next generation of GPUs toward targeted workloads in HPC, hyperscale, and of course, for the training and execution of deep learning jobs.
The company’s M40 GPUs are a key component of Facebook’s Open Compute-based Big Sur platform, and while they represent what happens to pricing at volume, Hamilton says that just looking at the pure purchase price of systems for deep learning is missing the bigger picture. “For example, if you’re just looking at buying 250 2-socket X86 servers with no GPUs, it’s quite easy to say the cost of adding in either Infiniband or 10 GbE will create something that costs more than DGX-1. And as for those who are cobbling together their own deep learning training boxes the far cheaper (and lower thermal) Titan X cards, this indeed can be done, but “if you look at what the mainstream OEMs are doing, none support Titan X in servers because they are not designed for that kind of 24/7 use in servers.” In short, there is nothing preventing the use of the $1000 Titan X parts, but the performance is not as high or tuned for such workloads as the M40 for training.
While Pascal’s thermals are bit too intense for some deep learning shops, and the M40 and M4 need to develop a use case life of their own over the coming year (hopefully on more applications beyond image and speech recognition), Nvidia’s strategy for deep learning, as it turns out, is complementary to its high performance computing push. The goal is to create differentiated GPUs for accelerating certain types of workloads. In the Tesla division, with K80, now Pascal, and older generation GPUs, including the K40 and K20, will continue to back the fastest supercomputers while other specific, targeted workloads, including deep learning, can get a break from the heat, use mere single precision without the heavy overhead of a Tesla GPU, and accelerate more efficiently.
Stay tuned as we walk through these differences in HPC hardware for deep learning based on another chat with Baidu in the wake of Pascal—and what a card like that might mean for big deep learning users at scale.
Be the first to comment