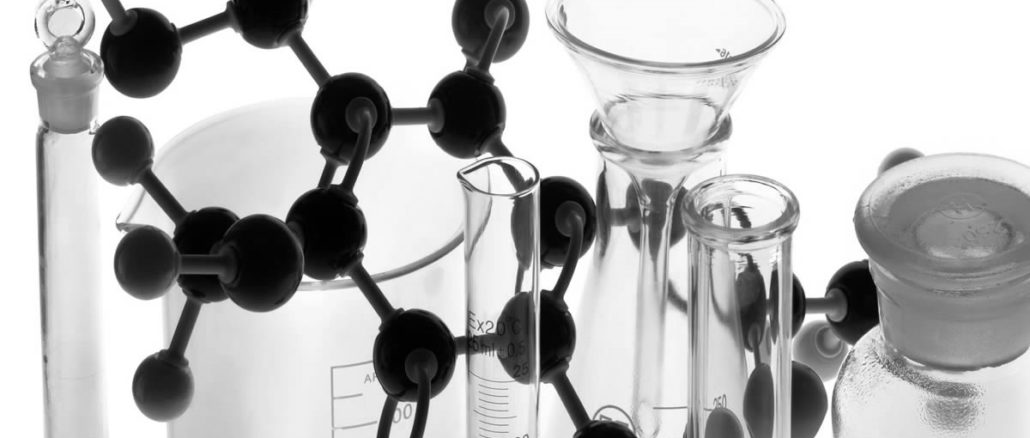
Researcher Olexandr Isayev wasn’t just impressed to see an AI framework best the top player of a game so complex it was considered impossible for an algorithm to track. He was inspired.
“The analogy of the complexity of chemistry, the number of possible molecule we don’t know about, is roughly the same order of complexity of Go, the University of North Carolina computational biology and chemistry expert explained.
“Instead of playing with checkers on a board, we envisioned a neural network that could play the game of generating molecules—one that did not rely on human intuition for this initial but very challenging step in any drug discovery project.”
Since that initial inspiration, Isyev and his research team have put the concept into practice at UNC and other institutions. Working with families of proteins, the group’s goal to create a molecule that binds to a specific protein has been realized and while they were able to speed the computationally intensive first step in de novo (from scratch molecule-based) drug design, the applications of the same generative and recurrent deep learning approaches they used for their work cannot be extended to the much slower drug discovery pipeline that comes after.
While neural networks might help drug companies find new molecules to test far faster than with human-centric or other slower computational approaches, the next step—synthesizing the molecule and testing it in physical environments to meet regulatory approval—is bound to its historically slow timetables. So while we cannot say that deep learning is revolutionizing the entire drug discovery pipeline, it can play a role in speeding new molecules to the test stage and opening the door for broader applications of deep learning in other parts of the testing phases eventually.
In this game of generating molecules, there are two players. The agents generate organic molecules while the environment scores these. In this case, the scoring function is a user-defined chemical or biological property or something that binds to a specific protein. So if the game is played it is possible to teach the machine to present new molecules that are predicted for specific proteins, for example. Ultimately this presents a new way to generate new types of molecules intelligently (i.e. without mere human intuition).
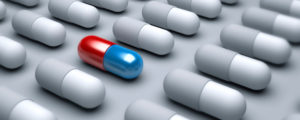
The technical side of the analogy is a strategy that integrates two neural network types, generative and predictive, that are trained separately but employed jointly to generate novel chemical structures with the desired properties. Generative models are trained to produce chemically feasible results, and predictive models are derived to forecast the desired compound properties. In the first phase of the method, generative and predictive models are separately trained with supervised learning algorithms. In the second phase, both models are trained jointly with reinforcement learning approach to bias newly generated chemical structures towards those with desired physical and biological properties.”
Unlike the initial Go winning algorithm, which was a very new and tailored approach to AI at the time, deep learning frameworks like PyTorch have allowed researchers like Isayev to go from concept to proof-of-concept in far less time than if they were using the rudimentary versions of the AlphaGo victor. The team initially started the effort using Theano but switched to PyTorch for even greater ease of use. While he says TensorFlow is appealing, for researchers that want to focus on chemistry and the problem at hand more than the ins and outs of the framework, this decision made sense.
This brings up another interesting point. Just as computational chemists do not want to worry about the underlying software frameworks (they just need to get their science done), the same goes for hardware. Isayev tells us that while many pharma shops have smaller GPU clusters and some GPU workstations, they often do not have the same infrastructure for HPC or deep learning training that some other areas in science and web companies would have. They are simply slower to adopt new hardware in part because it is not their core competency. At the end of the day, whether a molecule makes the cut depends on physical testing versus HPC simulation. Still, he says having a deep learning framework that is easy to interface with like PyTorch and can be used on the smaller GPU clusters in house at many shops could be appealing.
Even though the hardware and software pieces might be in place for drug companies to speed the first step to drug discovery, Isyev says the excitement over deep learning further down the pipeline could take some time to build. “In pharma they are often cautious about new methods that claim to solve many problems at once. There are many iterations and ultimately, a lot of those methods that were developed in the 90s and early 2000s overpromised and undelivered.” He says that while there is a lot of progress for deep learning in other areas (and even with their own proof of concept) the sense of those achievements is still conditional.
A deeper look inside this “game of molecules” can be found here.
Be the first to comment