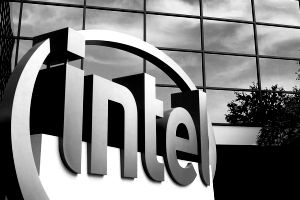
Over the last few years, every major technology company has made a grand movement to embrace data in much the same way they did in the mid-2000s with cloud. New divisions were launched, investments pushed, and of course, new “evangelists” were assigned to espouse the role of the anointed technology platform.
Intel certainly fits into that camp, with new divisions attached to the Data Center Group mothership rolling out to match evolving trends. And while the backstory can often be crammed to fit into the “customer demand” corner, what’s interesting in some cases, including the chipmaker’s, is that the real impetus (in this case on the large-scale analytics or, (okay, just this once) big data side, came from within.
The backstory of Intel’s own data infrastructure team, which is led by former Intel IT engineer, Ron Kasabian, is one worth telling. Because much of what Intel encountered in its long journey to make sense of its own disparate data has been echoed by a large number of big enterprise shops we have talked to here at The Next Platform. The data is scattered and siloed; the maturity of the toolsets to mesh the data is still lacking in some areas; and the expertise required from the many required algorithms and models that make a large-scale predictive or in-depth analytical platform functional at scale is also somewhat siloed and hard to merge.
Under then-CIO, Diane Bryant, who later placed him at the helm of the Data Center Group’s data and analytics division, Kasabian struggled with the still-evolving and immature ecosystem tied to Hadoop, as well as with a range of legacy databases and tooling. Over a course of a couple of years, he led the team to develop a wide-ranging, ultra-complex predictive analytics program that spanned the entire Intel organization and culminated in far more efficient testing and production at the company’s fab facilities.
This program resulted in a reduction of the validation cycle for new chips by three to four months and as of 2014, saved Intel $500 million. For Kasabian, however, there was another big value point—he was able to see firsthand just what Intel’s customers at large scale are talking about when they grouse about data challenges. And while he agrees there’s some undue hype around the term “big data” there was no other way to describe their process of getting data collected across multiple parts of the organizations to even see if there was any potential value in it. The analytics are the second hard part. And this is something that can take many months, if not years.
“There is a lot of siloed data inside Intel. It was never natural behavior for those different groups to figure out how to share it,” Kasabian recalls, agreeing that this is echoed among the large-scale end users with widespread infrastructure and multiple teams who simply don’t share. Intel had some data in Teradata data warehouses, some in other business intelligence systems, and some that was less structured and needed to find its way into the mix. This process took close to a year, he says.
Aside from the collection and meshing of disparate datasets from across Intel related to how parts performed, the predictive analytics approach the company finally cobbled together out of several open source pieces tells the real story. We’ll get to those components in a moment, but in essence, what Intel built was a predictive system to help its chip testing teams predict which lots might not even be worth testing based on where they were on the die and what production lines they came from. Since Intel runs tens of thousands of tests to see what bin split parts should go into, short circuiting those tests by determining which chips can skip 1 GHz and 2 GHz testing and move up through the line significantly reduced the time to market for new chips and avoided wasted expense in massive testing efforts.
“Articulating the business value of data that’s spread across multiple parts of the organizations is really hard,” Kasabian states, noting that these types of challenges were the impetus for Intel to spin out the big data division within the Data Center Group and put him at the helm two years ago.
“In 2012 or so, there weren’t people talking about Spark and all the things you could do at scale. It was Hadoop and the amount of analytics possible there were still emerging. We had a bunch of tech; from MPP databases, an enterprise data warehouse, a bunch of tools for pushing data to and from certain points. But there were no sophisticated analytics packages. There was no H2O, there was little. And on top of that, we were taking 12 to 15 different software components to stitch together that were never designed to go together. Not to mention the fact that a lot of domain expertise was assigned to these tools and the custom creation of algorithms and specific models. It took a very long time.”
The question is, how much has changed for large organizations that have, over the course of years (if not decades) now that some of the frameworks for managing and integrating data at scale evolved? Kasabian says that there are a lot more in the way of capabilities to choose from that integrate better but the fundamental challenges in meshing together data and seeing the big picture for analytical strategies is still a big roadblock.
While the hype curve might weigh in favor of the mighty BD buzzword and the Hadoop ecosystem appears to be thriving still, the big cultural and technical transitions for big companies present a tough road—and there are plenty of organizations that are still at that beginning stage, despite all the interesting users at the Fortune 1000 level we talk to here. Compliance, silos, lack of wide enough technical domain specialization, inability to integrate open source tools that aren’t commercially supported, and even lack of like file and data types are still the reality for many companies, even if the stories that get all the attention are about companies who have stepped into a new realm of platforms to discover what we keep being told are massive goldmines of raw data buried in those distantly placed silos.
And here was another interesting takeaway from Intel’s general manager for big data. It is that there is no one single approach or platform—there is not a single magic bullet on either that data collection/organization/de-silo-ing side, nor on the technology platform side–that is poised to solve the woes described. But there are movements—and no, Hadoop, despite Intel’s massive investments in the distribution vendor, Cloudera, is not the cure all, even if it is vastly important. On the Hadoop front, Kasabian notes that this came about due to his team’s direct use of Hadoop and its subsequent tooling (they have a team that develops and contributes back to the open source Hadoop stack as well), but Hadoop is still evolving—as are the tools that are hooked into it.
Kasabian says that the task for his team at Intel, especially as they are out talking to end users in the field, is to identify the algorithms, models, approaches, and problems that exist and looking to the ecosystem, both open source and through internally developed software tools to target those things. And for a chipmaker that keeps beefing up its software portfolio, and for a data lead who knows firsthand what some of the real firsthand problems are when it comes to large-scale analytics challenges, this is worth something. Even if Hadoop use hits a plateau, there next phase of analytics, which will include machine learning and more advanced approaches to seeing data in a new light, are also on the drawing board. While Kasabian pointed us to folks for a follow up on that front, he agrees that there is yet another evolution of analytics on the way.
Be the first to comment