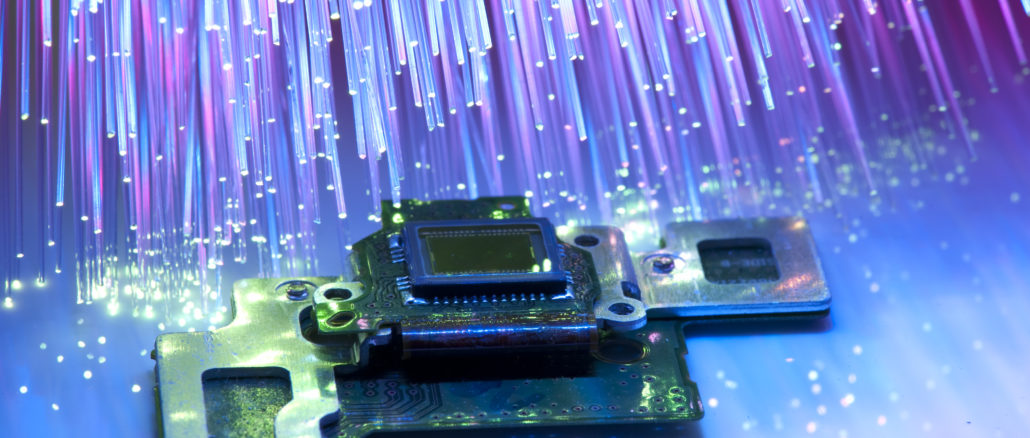
It is not every day when we hear about a new supercomputer maker with a new architecture, but it is looking like Luminous Computing, a silicon photonics startup that has been pretty secretive about what it was up to, is going to be throwing its homegrown architecture into the ring.
When Luminous Computing was founded in 2018, it was joining the growing list of established tech firms as well as startups that are looking to use silicon photonics to build chips that are faster and more power efficient to support modern artificial intelligence and machine learning workloads. The company’s focus was on what Marcus Gomez – one of Luminous’ founders and now its chief executive officer – called optical computing, or using optics to solve the math problems inherent in AI computing.
Using silicon photonics to address those problems was where Gomez’s attention aimed and the subject of research done by Mitchell Nahmias, the other founder at Luminous and now the company’s chief technology officer, during his time doing research at Princeton University and the years since.
Soon after the startup raised $9 million in seed money in January 2019 that Luminous made a sharp turn away from the original idea of addressing the compute aspect of AI computing to looking at the communications – between the parts within the chip as well as between systems, racks and datacenters. That is where the problem lies and that is where optical technology can do the most good, according to Gomez.
“If you look at this work, we are 5X to 10X away from the theoretical density limit of digital,” Gomez tells The Next Platform. “Compute is not the problem and this is a hard pivot away from what we’ve canonically focused on. The computing stuff, it’s a really cool science project. In 2030, it may very well be important technology for at least edge computing. But for the modern AI user, the bottleneck is not compute. The first thing that we are doing is using optics to make our digital transporter before we go to a brand-new platform to do logic and computation.”
He points to Nvidia’s A100 GPU accelerator, which is optimized for AI workloads. He estimates about 10 percent to 20 percent of the chip’s time is dedicated to computing.
“This is a chip whose sole purpose in life is doing computations and it’s mostly not doing compute,” he says. “It’s mostly doing memory and interface and interconnect. At a basic level, it’s because you don’t have enough bandwidth going into the chip to feed it anything faster than that.”
Rather than just looking at the processor and seeing how photonics can help there, the plan at Luminous now is to build a complete AI supercomputer with its optical technology being the key connection between all the different levels.
“We are building the whole computer,” Gomez says. “It is a rackable solution, an entire supercomputer, and we are building all of the parts of it. This involves multiple digital chips like the computer chips, the switch chips, and the memory interface chip. We are building all of the optics to connect all of these chips together and make them scale nicely, and we are packaging it all together and then we are building all of the software on top of it. So TensorFlow and PyTorch machine learning frameworks will just run out of the box on Day One.”
The chips themselves will be digital chips, but the key is that they are optically connected, eliminating the inbound and outbound communication restraints and relaxing the strict requirements of traditional computer architecture, he says.
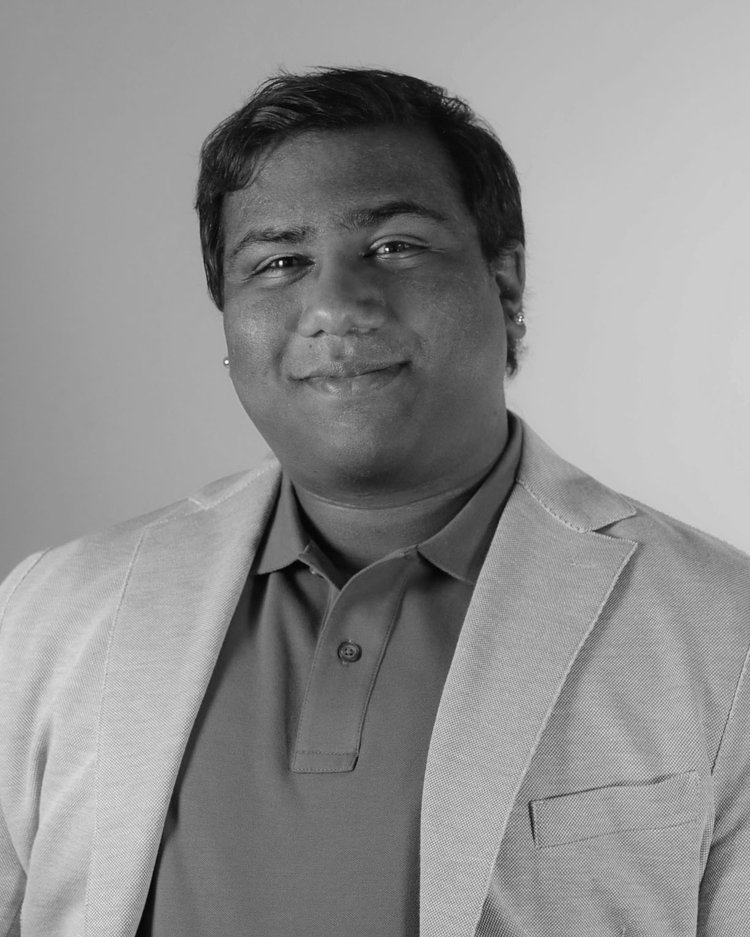
“We’re using this technology to build ultra-high-bandwidth data links and we’re inserting them into the computer architecture exactly where you get stuck in these communication bottlenecks at every scale – at the lowest level between memory and processor, all the way between board-to-board, box-to-box and rack-to-rack,” Gomez says.
Gomez, who dropped out of Stanford University’s master’s program to launch Luminous, comes to this with a varied background, which includes being a research scientist at dating app company Tinder in 2018 and research roles at Google in machine intelligence as well as a stint at the Mayo Clinic. He also was a software engineer at Bloomberg and a researcher of network biology at Harvard Medical School. Nahmias received his PhD in electrical and electronics engineering from Princeton, where he researched the relationship between a laser and a biological spiking neuron, part of the field of neuromorphic photonics.
Matt Chang, vice president of photonics at Luminous, also received a PhD in electrical engineering from Princeton and spent two years at Apple designing hardware to reduce interference between co-existing wireless radios on the Apple Watch. He left there in 2019.
Improving the communications within and between components will be key to enabling larger AI models and making training such models more accessible.
“Ten years ago, the largest model you’d see in literature was in terms of 50 million or 100 million parameters,” Gomez says. “It sounds like a lot, but it’s not. You can fit that on a single GPU and you can train it in an hour. Today, the biggest models in the literature are on an order of 10 trillion parameters. The biggest models that we’ve seen take up to a year to train and they take tens of thousands if not up to hundreds of thousands of machines. Therein lies the problem, because when you start talking about training times of a year, you’re hitting the limit of what a human can reasonably run an experiment for. When you’re talking about hundreds of thousands of machines, you’re running out of space, not to mention how expensive hundreds of thousands of machines are. You get to the point where basically only a select number of companies are capable of building these giant AI models. It’s getting too expensive. It’s taking too long, and it’s taking too much space. There are not enough chips.”
Organizations running large AI workloads need to make a tradeoff between performance or cost and programmability and even then, the performance gains made via more compute capabilities can’t keep up with the growing sizes of the training models. This is where Luminous sees that the bottleneck in AI is the communication and not the compute. Thus the startup’s hard pivot.
“Optics has always been the known solution to the data movement problem,” Gomez says. “This is why they lay fiber optic cables in the Atlantic Ocean. Light is good at moving data across long distances in a high-bandwidth, low-latency fashion. Once we eliminate the bottleneck, this gets us two things. One is we actually get the magnitude improvements in performance. You might be able to train models that are 100X to 1,000X larger on our systems than you will be capable of training on any other modern hardware in the next five years. For existing models, we are going to take training time that used to be years and take that down to months. We are going to take things that used to run for months down to days.”
In addition, the programming model gets simpler by reducing the number of distributed systems needed to run the workloads and the system is less expensive because the scaling is more efficient.
There also is the challenge of software. Luminous need to ensure that existing machine learning code can run on its systems, which means getting TensorFlow and PyTorch to port over to them. Gomez says the company has “a fairly hard compiler problem to solve and we’ve got a bunch of really extraordinarily talented engineers working on that. The key in the software is going to be to make the scaling magic trick – which is effectively what it is – actually appear like a magic trick to the customer.” The fact that all the communication is high-bandwidth and the data movement is essentially fixed-cost will help because “there’s no distributed systems thinking and there’s no hierarchical thinking required. You just grab the next available resource. In some sense, the algorithm just works.”
Luminous has been purposely flying under the radar for much of the past few years and even now Gomez is reluctant to go into too much detail about the technology the company is building or its roadmap, though he is now more open about the shift toward building an entire system rather than focusing on chips.
The company expects to have productions systems for sale within 24 months and it just got a $105 million infusion via Series A funding from a wide array of investors, including Bill Gates. At the end of 2021, Luminous had close to 90 employees. The newly raised money will help it grow that to be well over 100 employees – including doubling its engineering team and adding engineers in photonics designs, digital and analog very large-scale integration (VSLI), and packaging as well as machine learning experts.
The startup – which has working prototypes in its labs – has shifted out of the analysis phase and into the execution period, which means it will need to be more public about what it’s doing. This is important not only for recruiting and selling but also for differentiating from others in the silicon photonics field, which includes not only the likes of Ayar Labs, Intel, and IBM but also well-funded startups like Cerebras, Lightmatter, Lightelligence, and Celestial AI who have their own angles on this.
A key differentiator is that while there are “many companies thinking about using optics for computation, we totally abandoned that,” Gomez says. “But at a more fundamental level, all the computer architecture decisions we’ve made and all of the optics we’re introducing, it’s not arbitrarily introduced to have just a physics advantage. It’s being specifically used to make the user experience of building these massive AI models as simple as possible. That’s a conversation that, as far as we can tell, nobody else in the hardware space is really having.”
Sounds like these guys are good at repackaging as new what everybody else is doing. Co-packaged optics going between digital computing chips and high radix electrical switch chips is something everybody else is working on and it looks like these guys don’t have any magical solution. Just a bunch of big words. Unless they are solving the issues brought up recently on Next platform by Andy Bechtolshiem, no one is gonna care. Who are they working with for the compute side? Are they building their own digital compute as well as their own switches and their own optics? And their own software and OS? If the answer is yes, then 100M is nowhere close to the kind of money they will need and it’s not clear that they are any better equipped to solve all the problems than anybody else