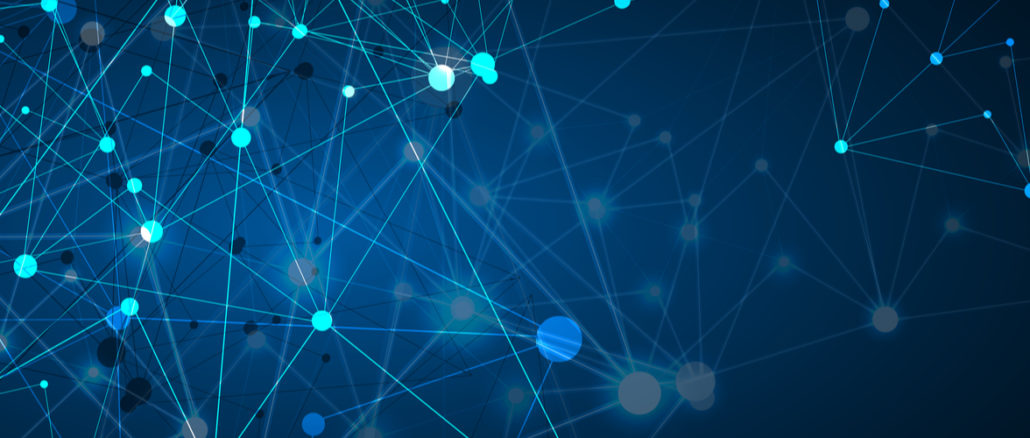
Covering developments in neuromorphic computing has been something of a piecemeal experience, as happens with all novel architectures. There are few companies with scalable devices and the research is often specific to one experimental architecture or use case. In other words, no total picture has emerged for neuromorphic yet, especially for the datacenter.
Perhaps in response to this lack of ecosystem-wide clarity, a wide vendor and research collaboration has put together a roadmap for neuromorphic computing that provides past, present, and future challenges for the various approaches and most important, provides insight into the range of applications these technologies might capture first—if at all.
What is most notable about the neuromorphic roadmap is the absence of datacenter-centric applications. Creating scalable neuromorphic systems has been on Intel’s own roadmap with Loihi, for instance. The low power and potential for high performance networking held early promise, especially as the first wave of AI chip startups hit the market five years ago. However, the broad base of contributors to the roadmap rarely mention devices or applications that can address scalable distributed computing. Not that this is a surprise, after all, this is still a nascent area. But if we take this wide survey of neuromorphic in 2021 at face value, the area has shifted to an edge/embedded story.
The roadmap does include discussions on current and future projections for various neuromorphic device materials, including phase change and valence change memory, ferroelectric devices, nanowire networks, spintronics and novel 2D and organic materials for composing neuromorphic systems. A review of many of these, with the exception of memristors and phase change memory still appear to require quite a bit of research to gain any commercial traction, even for edge/embedded.
Segments of the roadmap include analysis of where neuromorphic might fit for future deep learning, spiking neural networks, and what hardware might be best suited for these areas. Roadmap contributors from UCLA (Peng Yao and Joshua Yang) see non-volatile devices based on resistive switching technologies (memristor, phase change, ferroelectric devices) as applicable approaches for AI. “With the utilization of more emerging devices and advanced 3D integration processes, in-memory computing promises a bright future for deep learning hardware.”
Oxford and Munster researchers added a discussion on photonics to the roadmap, citing the precise needs from foundries to push photonics into the mainstream, particularly for AI applications. “New challenges in handling huge amounts of data in the fields of artificial intelligence and machine learning that bring conventional electronic processors to their limits have resulted in a surge of interest in photonic computing. This is because of their inherent advantages: high throughput, a high degree of parallelization together with the ability to carry out linear operations at very low energies.”
Spiking neural networks, which have shown promise in real-time sensing and edge applications, have long been a target for neuromorphic development. But as Giacomo Indiveri from ETH Zurich notes in the roadmap, making this a practical reality will take quite a bit of ongoing work. “Implementing spiking neural network computing systems with analog CMOS circuits and memristive devices is hard. Besides a few example proof of concept systems applied to very specific tasks, such as sensory processing or spatio-temporal pattern recognition, no general purpose solution exists yet.” He adds that while there is great promise, especially for edge applications, there is also no “well-established formal methodology that exists for automatically designing or programming them.”
In addition to positioning various neuromorphic hardware approaches, the roadmap touches on key algorithms, including the software side of spiking neural networks, computational neuroscience, stochastic computing and reservoir computing, along with CNNs and other neural networks. This sets up what is the most valuable section in our view, an analysis of where commercial applications are most promising.
Although there has been much written about the datacenter (accelerator) role neuromorphic devices might play in future systems, the roadmap does not assign much value in this area. Rather, the target application areas include embedded and edge applications (robotics, autonomous vehicles, sensor-driven and time-series edge systems, and even wearable technology.
The lack of datacenter-centric applications is telling. While neuromorphic devices were heralded a few years ago as potential rivals to GPUs and non-brain inspired AI ASICs in datacenter acceleration due to their lower power consumption, the roadmap does not reflect this, favoring instead reality due to manufacturing, maturity, materials, and more.
Be the first to comment