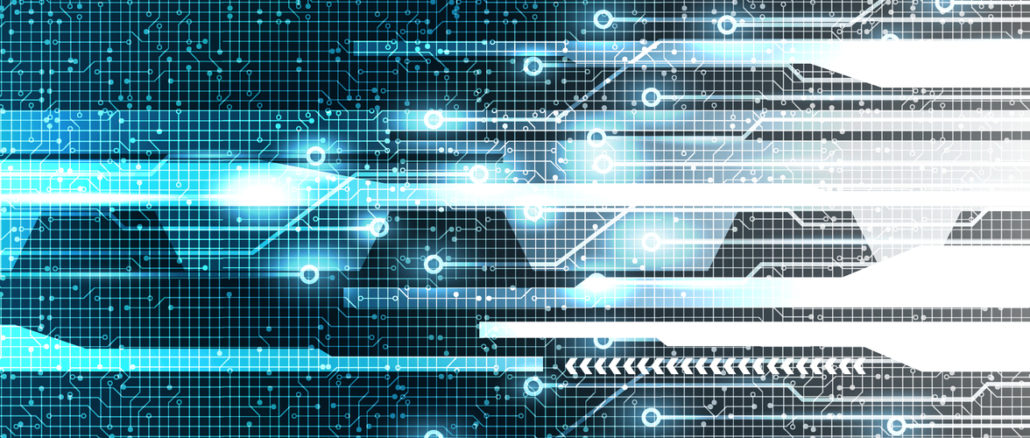
To catch a glimpse of the future of what memory devices and approaches will dominate the AI-centric datacenter, there are few better forecasters than Evangelos Eleftheriou.
Eleftheriou has been part of IBM Research Zurich for thirty-five years and has been behind seminal research into dozens of computer storage and memory technologies, including pioneering work in some of the central capabilities that made magnetic recording possible. He is also well known for his work on phase-change memory (PCM), including problem-solving around some of the trickiest issues, including eliminating drift in PCM devices. More recently he has dug into neuromorphic computing, specifically focusing on an complete phase change-based neuromorphic architecture.
In addition to his work on devices, he has also been investigating role of emerging memory devices for AI workloads, both for inference at the edge and training. While there’s been plenty of attention heaped on in-memory AI over the last few years, especially among ML hardware startups, Eleftheriou believes here is still untapped opportunity in the face of data access and movement and energy consumption bottlenecks. He says that there are possibilities in introducing novel computational primitives that can facilitate deep learning workloads in memory.
Despite his wide purview of the myriad novel devices, Eleftheriou is steadfast in the idea that SRAM with its reliability and ability to take on higher precision work, will take the center stage in the near term, especially since other technologies, including PCM and resistive RAM (rRAM) have endurance and scalability issues that are not likely to be resolved in short order.
“The emerging non-volatile memory technologies like PCM or rRAM clearly provide low power consumption but they have finite endurance in contrast to SRAM. I would view those technologies as candidates for inference engines for edge applications but even there you could also use SRAM, which doesn’t have the same limitations,” Eleftheriou explains. He adds that the real advantage of SRAM is playing out in recent research that highlights higher precision capabilities of SRAM, from 1-bit to Int-8 accuracy, something that could push more in-memory AI inference in particular into the cloud, he adds.
Still, he believes that there are roles for non-SRAM devices to play in the in-memory AI market, an area he expects will grow over the next five years. We have already seen PCM on the market despite some the limitations he cites and that will continue to have momentum but as for rRAM, Eleftheriou is less optimistic. “It’s not clear if it will make it as a technology. It’s not straightforward, there is a lot of variability and as that scales, it will create larger problems. PCM has the better opportunity; it’s not a new technology, it has been deployed and we understand well the problems and the physics.”
In five years or so, what I would like to see is the emergency of non-volatile memristive technology, probably as low power consumption inference engines for many edge applications. I also see more SRAM approaches with higher precisions for more demanding workloads and deployments in the cloud.
It is interesting to note that despite all the interest in rRAM in recent years, he also sees a dwindling in interest and commercial momentum. “The activity around rRAM is not as high as it used to be,” Eleftheriou says. “There was a lot of hype five years ago but it has somehow faded away. For us, our focus is on PCM, we understand that well inside IBM in addition to SRAM for different deployments.”
In 2020 alone, Eleftheriou has published on a variety of in-memory AI topics, including the development of an SRAM-based in-memory matrix-vector multiplier with Int-8 precision and promising scalability and power efficiency and research on mixed-precision deep learning in computational memory and in multi-memristive devices. He has also been exploring increased precision of SRAM and other devices in addition to a compilation mechanism for neural networks for in-memory processing.
He delivered a keynote on the topic of innovations in in-memory computing today at the HiPEAC 2021 (online this year, of course). More info here.
Be the first to comment