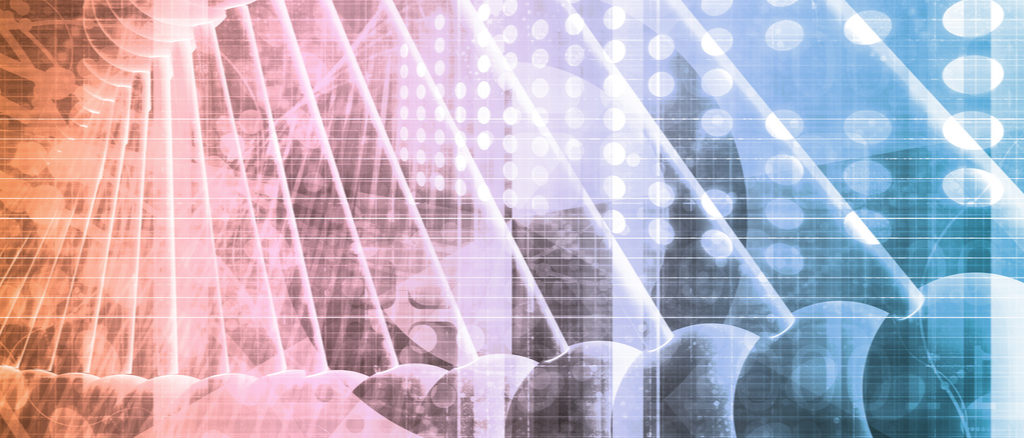
Don’t expect big on-prem HPC hardware investments from biotech companies given COVID drivers. While storage and more nuanced GPU deployments will push some spending, especially from startups, cloud has become even more important and data analysis, management tools are also central. More important? Budgets have to support distributed workforces and collaboration platforms.
It might be tempting to think that a rise COVID-19 related research and development for drug discovery and related biotech initiatives could propel computing infrastructure investments, particularly among the world’s largest pharma and biotech companies, but this is likely not the case. While there are several government programs that are pushing investment into COVID research efforts, the real computer science drivers have less to do with hardware capabilities and more to do with data management and analysis, especially in commercial biotech.
There are some caveats to this overall outlook. While commercial companies might be less inclined to make large fresh investments in their own datacenters, centers in research and academia have already been a different story. With CARES Act funding in the U.S. and similar efforts elsewhere in the world, HPC and some non-profit centers have been able to upgrade or spin up new clusters dedicated at least in part to molecular modeling and other relevant workloads. Aside from these research-oriented centers, hardware investments from stealth and startup biotech companies will also continue, if not stronger than usual given cloud reticence (security, regulatory, etc) matched with the unprecedented volume of data available—and not just for COVID-related cures.
As for where on-prem hardware investments exist and could grow, there are a few areas of interest, according to Chris Dagdigian, Co-Founder and Technical Director of Infrastructure at BioTeam, a consulting and services group with deep roots in HPC and biotech hardware and software. Overall, any potential gains from government or other funding in response to COVID will be negated by costs borne to other parts of the organization. As Dagdigian tells us:
“Candidly, from talking to our customers, we don’t see any signs of exploding budgets due to COVID. It’s belt-tightening, actually. The influx of funding and resources related to COVID is being offset by the expenses of supporting an entirely mobile workforce with huge collaborative needs. The market itself will be flat and with competitive offsets. Anytime you see a boost for HPC or analytics, they’re dealing with other costs so the effect on the HPC market will be net neutral or with a bit of a downturn as people shift or postpone new builds or infrastructure spends. The only exception seems to be startups building new labs with new on-prem infrastructure.”
Dagdigian points to storage and GPUs as elements in the stack that will require some special attention, especially with an increase in AI/ML in workflows ranging from genomic analysis to drug discovery and beyond. For reference, his team has been entrenched with some of the top pharma and biotech companies over the last nearly twenty years with specific emphasis on HPC systems and tooling with roughly equal footprint in federal/government bioHPC and commercial life sciences companies.
“In the past, scientists have always been worried about running out of storage. We were able to buy big storage and solve capacity requirements but there were financial tradeoffs. They could get big storage but necessarily the fastest,” Dagdigian says. “Until recently that was an invisible tradeoff. They would trade availability and even performance to have enough storage.” What bucked that tradeoff trend is the arrival of AI/ML with new demands on storage. “AI/ML changes the storage performance style because the speed of I/O and storage systems has an impact on the speed of training a model and how much it’s possible to train. Further, if you’re always going back and training on old data that means it can’t live on a cheap tier, it needs to be accessible.”
In other words, the old trend in HPC beyond just life sciences is starting to break with big, slow, capacity-driven, disconnected islands of storage (archive, nearline, performance, etc). The rise of AI/ML has meant storage needs to be consolidated or at least globally addressable. This also means performance and throughput requirements are edging up and for this, there are no tradeoffs if the goal is to have cost-effective AI/ML.
GPUs are another area of interest in terms of on-prem growth but it goes beyond just number of devices installed to more nuanced installations. Even though the technical considerations for GPU deployments are more fine-grained, this is one place where hardware budgets will be stretched.
“The trend we’re seeing now is that it’s necessary to be much more careful about planning and procuring and managing GPUs. It’s not just about picking a GPU these days, it’s about coming up with a strategy to place and interconnect those. There are many different scientific workloads that work best with one GPU per node, others need multiple GPUs in a single chassis, and a third class of those problems want fancy interconnects,” Dagdigian explains. “In the early days you could get away with putting one GPU card in a node to satisfy the needs of chemists, cryo-EM, structural chemists and so on. But now we have to make very specific decisions about what GPUs, how many per chassis, and what workloads need NVLink. It’s harder to plan for a GPU deployment now because these technical decisions need to be baked into the purchase and can have long-lasting ramifications about what workloads you’re optimized or biased for.”
Among the broad range of biotech HPC use cases in research and commercial spheres BioTeam sees, Dagdigian estimates around 80-90% of the sites they’ve been involved with have GPUs on site and 100% of them have at least a rack or fixed allocation. “GPUs are endemic and beneficial to more than just one workload. You’re probably not doing computational chemistry, molecular modeling, or structural biology without at least some part of that running on GPU. Beyond that, you’re not running a PacBio gene sequencer without a GPU because the SMRT Analysis software uses GPUs to crunch the data coming off the instrument. Cryo-EM uses GPUs for certain steps. The use cases are so huge that it’s now unusual to find an environment where they’re not built in.”
As a side note, Dagdigian also points to one emerging trend that he says will have an impact on what biotech HPC centers put on prem. He says the competition between AMD and Intel is heating up and while they haven’t seen the shift to AMD yet, he is encouraging more benchmarking from companies that are making big system investments. This is not just a CPU comparison, he says that AMD’s GPUs are competitive with Nvidia’s offerings according to their own benchmarks. The problem is, support and procurement folks at many of the commercial biotech companies have long memories and they haven’t forgotten issues with AMD, something the company has worked hard to quell in HPC with some notable wins at some of the largest supercomputing sites on the planet in 2020 alone.
Ultimately, and not surprising if gauged against other segments of the economy that are driven by IT (which is nearly everything in 2021), the cloud is the real winner. It’s not just about the flexibility and built-in tooling, datasets, and collaborative platforms the majors provide, especially in this age of distributed teams working remotely, it’s also very practical. Quite simply put, even if a company wanted to spin up a new on-prem datacenters, for most of 2020 it was hard to move equipment in. It wasn’t just about supply chains, it was about staffing and the management required. This was an impetus for more companies to take the leap when they might not have been so ready.
The one other exception to this rather grim news for the HPC hardware business is in traditional HPC. The large academic research centers have been able to bolster their computing resources or add entirely new machines. This is an important part of the story, says Brian Osborne, Bioteam director.
He doesn’t believe COVID has implications or ramifications for compute in drug discovery (and nearly all life sciences companies are involved in this directly or indirectly) but it definitely matters for the larger life sciences research world. “It’s the massive sharing of genomes. When you’re talking about examining human genetic variation and how that does or doesn’t affect clinical outcomes, that’s major compute, not just a server or two. It’s supercomputing class compute. These are datasets the size of which we’ve never seen. It’s population data overlain with clinical outcomes and layered with genomic variance—we’ve never seen this number of infectious agent genomes in the history of the world. Human datasets and infectious agent datasets distributed over the globe mean that in the research world this work, in part spurred by COVID, is life-changing.”
“There are still some open questions. Do nation-scale system increase in number? Will nations have the funds to go from 10-20 supercomputers? I don’t know the answer but those purchases dwarf those at the commercial companies and are just as important,” Osborne concludes.
Be the first to comment