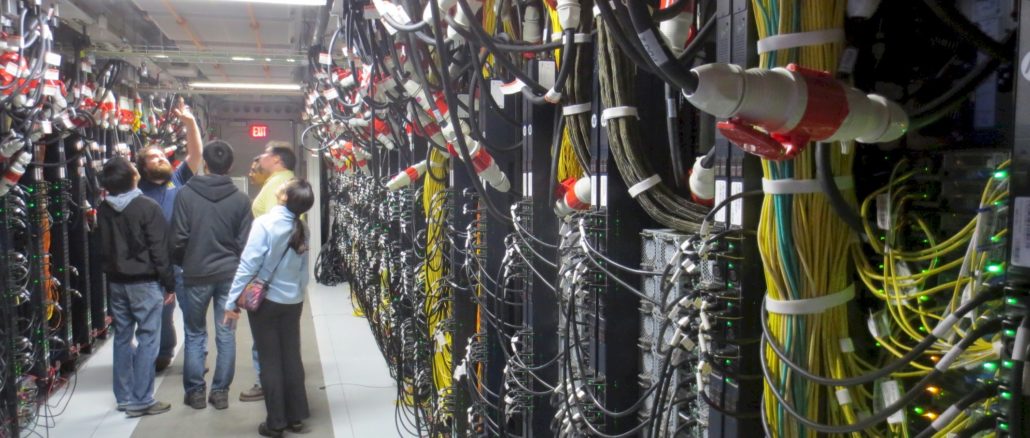
The University of Michigan is one of the top academic centers in the United States with over $1.5 billion in research expenditures in 2018. From discovering next-generation medical, sustainability, energy, transportation, and other innovations to supporting campus-wide general research efforts, the computational backbone has to be strong, well-organized, high performance, broadly accessible and relevant, efficient, and cost-effective.
This is a tall order for all universities and research centers but when it comes to cost efficiencies, the University of Michigan has some unique considerations.
All academic and research centers are committed to keeping compute costs low, but few are challenged to commit to full cost recovery. We will describe how these considerations factor into system-level decisions and where full integration, services, expertise, and partnerships play a more critical role than might be easily imagined.
We will also delve into the various choices The University of Michigan made to ensure a robust, usable system that can reach the widest number of users with the highest performance possible.
Building campus-wide compute resources is a major challenge. Balancing the need to offer bleeding edge technology to plow through workloads quickly is important, but so is creating a system that is general purpose enough that most users can easily take advantage of systems. The University of Michigan has struck an ideal balance up and down the stack and with the help of Dell EMC, can keep a high performance general HPC cluster highly available and efficient.
Running an Academic Supercomputing Center Like an Enterprise
When it comes to the cost of delivering world-class computing resources for a wide array of users, often with wildly different workload demands, academic supercomputing sites are laden with countless technical and usability challenges to consider.
Most are also burdened with questions upfront and ongoing capital expenses for large systems, but only a select few are further pushed by the need to account for and recover all costs. It is not common to think about campus-wide research clusters as operating like mission-critical enterprise datacenters with strict daily cost controls.
This is the case for The University of Michigan, where supercomputing investment decisions for campus-wide machines must factor in exceptional performance, usability, and management characteristics, as well as one hundred percent cost recovery.
In short, this is a case of an academic supercomputing site that operates under similar constraints to the most ROI-driven enterprises on the planet. This means every decision, whether compute, acceleration, storage, network, facility-centric, or operational has to be rooted in clear value.
To be fair, all HPC centers operate with a careful eye on costs, but as Brock Palen, director of Advanced Research Computing Technology Services at the University of Michigan, explains, ensuring cost recovery means making the smartest possible decisions based on a diverse set of workload profiles and generalizing value from those scattered points. As one might imagine, this is no easy task, especially with such a rich set of workloads ranging from compute-intensive to data-intensive to I/O bound with some single node (or even core) jobs in the mix as well.
Profiling workload characteristics and user requirements is relatively straightforward but what is more challenging is designing a system that can match those needs while building in room for growth and change.
~2,500 people access the Great Lakes cluster for simulation, modeling, machine learning, data science, genomics, and more. The cluster has > 44 NVIDIA GPUs inside Dell EMC PowerEdge servers often used for AI, machine and deep learning, and visualization.
With the addition of machine learning into the mix, fresh consideration for accelerators like GPUs and their software stacks becomes more pressing, for instance. The availability of more efficient, higher bandwidth network options present new opportunities as well. With more parallel jobs taking more than a few cores (or even nodes) that also kicks in new solutions that can get research results faster.
But of course, with all of this comes extra cost, and in some cases, added complexity.
We will unpack each of those issues in a moment, but first, it is worthwhile to understand the revolution Dell EMC brought to the university from an integration and professional services angle. These elements are often taken for granted, but for The University of Michigan, which has historically taken an “add as you go” approach to building clusters, the true value of top-down integration has been made abundantly clear.
Expert Integration Floats “Great Lakes” Supercomputer
The University of Michigan’s fresh approach to value-conscious supercomputing will be much easier to track, manage, and understand given the integrative skillsets Dell EMC brought to the table.
One might politely call the previous way of computing at the university “ad hoc” when describing the multiple generations of compute, storage, network, and system software that had been pieced together over several years. The University of Michigan had not purchased a fully integrated supercomputer in the past, thus multiple elements were brought together at different times (and piecemeal from different vendors), creating a management monster.
Monster or not, the need for compute at a major research center does not always wait for formal upgrade cycles. The system had to deliver for its wide range of users for a span that went well beyond the typical four to five year lifespan. Instead of acquiring a new integrated whole, teams met demand by adding pieces in response to user demand, a strategy that provided a resource but not one that was operationally efficient.
“We would get our compute nodes from one vendor, fabric from another, yet another for storage. We did not ask for proposals for unified systems,” Palen explains. “Ultimately we ended up with equipment of many ages and all the problems that come from many generations and different vendors. Even from a fabric standpoint, we could not follow any best practices. We did not have a torus or a fat tree, it was just getting another switch and dropping it in where we needed. This might have been acceptable for some users, but we want to support true HPC and be as efficient as possible.”
This piece-meal cluster approach was replaced by the group that Palen now leads, the Advanced Research Computing Technology Service. Its first cluster was also built from parts supplied from different vendors. This was called Flux, which is being decommissioned to make way for a new system fully integrated by Dell EMC featuring the latest Nvidia “Volta” V100 GPUs powered by NVIDIA’s AI and HPC accelerated software stack.
This new system, is the Great Lakes Supercomputer and it brings together all the ROI and value considerations matched against diverse user requirements. The result is a unique, integrated system that satisfies cost/value and workload demands, provides room for growth, change, and scalability, and lets the University of Michigan provide some bleeding edge elements without risk to the cost recovery mission.
Defining Value for Massively Mixed Workloads
Heading this mission for cost-effective, cost recovery conscious supercomputing is Brock Palen, the head of the Advanced Research Computing (ARC) Technology Services group at The University of Michigan.
The current 13,000 core Great Lakes supercomputer has to account for everything, from the hardware to datacenter maintenance, electricity, personnel, software environments, and full depreciation. It has been designed to expand up to 50 percent in the future.
One of the most difficult aspects of building a campus-wide cluster is making sure the technology balance is there to deliver widely usable services. “We had to do a lot of benchmarking to understand user behavior and what people wanted out of a system. In that process we found we did not have a good sense of I/O demand. For instance, there are several life sciences jobs that put significant demands on the network and I/O, even if they are not classic HPC, they still require a lot of the components of an HPC system from a high performance fabric and scratch file system,” Palen says.
He adds that they also realized that half of the workloads were not high core count jobs and access to storage and high performance data movement were paramount. Nonetheless, they still needed to support the existing large-scale traditional HPC jobs, as well as support an increasingly bright future for machine learning across disciplines.
“Our business model on the legacy system encouraged users to run longer, thinner jobs. People were more likely to get 20 cores for a month than 200 cores for a week even though the total amount of compute time was the same. We have fixed that with this system with payment by the hour consumed. We expect people to do one big job, finish it up, and get on with their science instead of going at the speed of the business model for the system,” Palen explains.
With Dell EMC’s expert assistance, the team came to several critical technology decision points rooted in current real-world user profiles and with an eye on what lies ahead as AI finds its way into an increasing number of research applications.
Technology Decision Making with ROI, User Value in Mind
While the Great Lakes cluster is not one of the largest or fastest research systems, it has some elements that make it unique. What is notable about these decisions is that they are based on diverse workload trends. These trends indicate a few things, including a need for a strong, reliable network; access to accelerators for certain high-value applications; requirements for a balanced CPU architecture; and the packaging of the system to enable the kind of cooling and system management ease the big HPC centers enjoy.
“We want to make sure users can do more large-scale, tightly coupled parallel HPC work and have it function more reliably and predictably than the last system,” Palen says, “and part of that is starting with the kind of true classic fabric most application and middleware developers would expect to exist.”
“We chose technology that is practical now,” Palen notes. “We are in the business of creating a usable resource so going toward novel architectures for an improvement in a few application areas is not practical, that’s not how we operate.”
While ongoing and capex costs are a concern at The University of Michigan, this does not mean Palen’s team made decisions based on what had the lowest upfront investment. From the outside, it actually looks like they made some expensive improvements to their previous cluster in terms of networks and switching, GPUs, and CPUs. However, as Palen explains, the ROI on each of these decisions makes the upfront investment less dramatic than it might seem from the outset.
For instance, the previous cluster was making use of some of the oldest HPC-oriented GPUs available, the Nvidia K20 and K40 accelerators. While these functioned long beyond their intended lifespan, a rise in the number of GPU accelerated applications from the CUDA ecosystem and researchers has led to an increased demand. “It is not just about demand,” Palen says. “The speedups for certain applications by moving to the Nvidia Volta V100 GPUs on Great Lakes blows the legacy GPUs out of the water.”
The addition of the latest generation GPUs can also be considered future-proofing the cluster for the rise in AI application counts. This is especially important for training, Palen says, which will need all of the elements that make a V100 more costly than its legacy counterparts (increased memory bandwidth via HBM2, TensorCore compute capability, more overall cores, a robust AI software stack with optimized models to speedup time to solution, and GPU accelerated HPC applications) . “Yes, you get faster if you spend more but if these jobs run far faster, it is worth the higher cost,” he adds.
The team made some datacenter investments to create a blast cooler, which blows frigid air from every angle to get maximum life out of their Dell EMC built cluster.
Real Network Connections
The real gem in this system is still something of a rare one. The Great Lakes cluster sports HDR100, which Palen says was directly enabled by Dell EMC and their ability to line up partners like Mellanox to deliver the kind of cluster the university requires in terms of low latency, high bandwidth, and cost effectiveness.
Oddly enough, the HDR100 element, when fully priced out, was not much different than other options.
“Going back to our desire to better support a wider range and number of jobs, we adjusted our business model to fit those users who want wider jobs done faster. We wanted that higher bandwidth fabric over the ad hoc ones we were accustomed to with inconsistent MPI performance,” Palen outlines, adding that the technology was not the problem, but rather the way it was built over time and was not planned. “When we put out our proposal, we asked for two different configurations. A 2:1 fat tree with a single rack or top of rack switch island configuration with the uplinks between racks just enough for storage I/O and parallel jobs staying in one rack.” He says they looked at this for cost reasons but found that with HDR100 they could connect twice the number of hosts to a given number of network ports. This meant the HDR100 breakout with the higher bandwidth and higher port count meant they could get the performance they wanted at a price point that was not as bleeding edge as the technology.
“We could have done HDR at an 8:1 oversubscription in an island and saved more, but the savings were not as much as we expected with the higher port counts. This is where we decided we could not save every dollar but that the cluster would certainly be able to achieve its mission of supporting those wider, faster parallel jobs.”
The economics of the team’s findings are noteworthy, but so is the discovery that an ad hoc approach to infrastructure can get messy quickly, especially from a network perspective. As Palen’s team learned during the previous clusters with on-the-fly upgrades and replacements, it takes true integration to make data movement seamless and high performance.
More than that, with expert integrators that have deep connections, it was possible for The University of Michigan to be on the leading edge with HDR100, something that would not have been available to them from Mellanox at all if they continued the ad hoc method of cluster construction.
“Dell’s early technology team sent a crew because the HDR100 cards were not even an official SKU yet. They came out with screwdrivers and static straps because they had to take off the backplane and put in a different one to fit in the chassis. They were willing to do the work to make all of this work. Dell knew what it would take from the beginning and they took care of it.”
The main compute is based on the Dell EMC PowerEdge C6420 machines, which put four two-socket nodes into a 2U enclosure as well, with high memory nodes based on the PowerEdge R640 and GPUs inside the PowerEdge R740.
Working with industry and government, the University of Michigan (U-M) is a leader in Smart City technologies, with the goal of advancing transportation safety, sustainability and accessibility. Mcity, in partnership with the U-M Transportation Research Institute, has 2,500 vehicles operating throughout a connected vehicle infrastructure in Ann Arbor– the largest connected vehicle deployment in the United States.
These privately-owned cars and trucks, plus city buses, along with selected intersections, curves, and freeway sites, are equipped with Dedicated Short Range Communication (DSRC) devices. These devices communicate information such as vehicle position, speed, and direction of travel that can be used to alert drivers of potential crash situations. The data collected from these vehicles can inform the work of developers building safety and other applications for widespread use.
“Advanced mobility vehicle technology is evolving rapidly on many fronts. More work must be done to determine how best to feed data gathered from sensors to in-vehicle warning systems. We need to more fully understand how to fuse information from connectivity and onboard sensors effectively, under a wide variety of driving scenarios. And we must perfect artificial intelligence, the brains behind self-driving cars.”
The benefits of connected and automated vehicles go well beyond safety. They hold the potential to significantly reduce fuel use and carbon emissions through more efficient traffic flow. No more idling at red lights or in rush hour jams for commuters or freight haulers. Connected self-driving cars also promise to bring safe mobility to those who don’t have cars, don’t want cars or cannot drive due to age or illness. Everything from daily living supplies to health care could be delivered to populations without access to transportation.
Racked, Stacked, and Ready for Research
Careful, balanced technology decision-making matched with Dell EMC’s expertise, partnerships, and technology services have ushered in a new era in HPC at The University of Michigan.
Gone are the days of the management concerns from organically built clusters as the Great Lakes cluster gets ready to fire up for users by late fall 2019.
For Palen’s part, being able to focus on the needs of the diverse end users and be able to present a solution that is fully integrated and ready for additions without extensive change management is a win.
“The professional services and technology teams made it possible for us to get this moving quickly. Having their team rack it and stack means we know it will just work. I would work with this team again in a heartbeat,” he adds.
Sorry, I got about half way through this article/puff piece before it twigged to see who the author was. Could I make a suggestion to clearly indicate “sponsored” articles at the main page listing.
Tmgcore’s OTTO