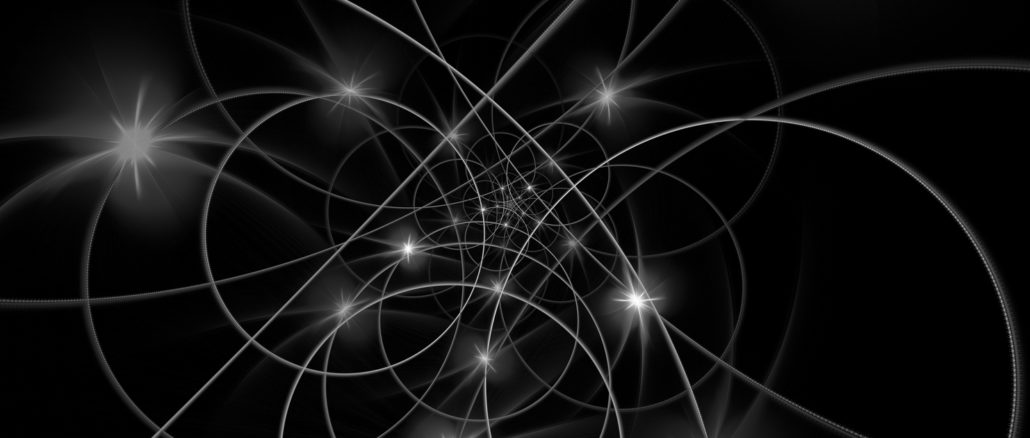
Like all major server-focused tech companies, HPE has been keeping close tabs on where quantum computing might go in the future. And while some of the company’s research leads see a bright future on a distant horizon for quantum’s role in drug discovery and materials research, there is little promise for real enterprise workloads, particularly AI if it does indeed find firmer footing among large companies at scale.
For this reason, one of HPE’s seasoned research leads has pulled up stakes on quantum investigation and shifted closer to the present-term, choosing to focus on homegrown processors for deep learning and analytics like HPE’s somewhat mysterious Dot Product Engine (DPE) and other forms of acceleration coupled with compute platforms that already available.
Ray Beausoleil is a Stanford-trained physicist who has spent almost 25 years at HPE. Throughout his career he has kept pace with current developments in quantum, memristor, and other technologies to understand how these might snap into future enterprise platforms. However, his tenure focusing on quantum has come to an end as he switches gears to consider more generalizable acceleration.
HPE’s quantum research began in the 90s with a team based at the Bristol office of HP Labs. Beausoleil was then put in charge of an experimental group in the U.S. to compete for government funds to support quantum research efforts but the effort was challenged by procurement and materials science problems. This group survived for seven years and its work then fed into exploring new ways to make quantum a better fit for high performance computing. Since then he has broadened his focus to include optics for both classical and quantum, much of which is fed by the early promise of memristors—a key component of the dot product engine, which HPE sees as an advantageous accelerator for a wide range of workloads; from traditional HPC to future neural network training.
Beausoleil tells The Next Platform that one of the reasons he ended quantum research is that for nearly all enterprise applications, problems can solved classically or quasi-classically, meaning that accelerators coupled with traditional compute can do the job. “Although quantum holds great promise, there are many roadblocks we need to overcome before it is feasible or even useful for delivering reliable, repeatable results—and we are still decades away from that, adding on to the fact that we’ve been considering quantum computing for 70 years,” he argues.
This is not to say that the potential for quantum is limited once key technical problems are solved, but it will not be able to tackle key problems in enterprise and scientific computing and may only find a home in those arenas as an accelerator for certain parts of a workload. In enterprise, however, he says that the role of quantum will be small since quantum systems are not good at the “three R’s” as they say in the U.S. (readin’, writin’, and ‘rithmetic). “We don’t yet know how to take a database, code it into qubits, and work on it effectively. In addition, the answers we put into and receive from quantum computers are still only probable.”
None of this is good news for the future of enterprise quantum computing—and in fact, it reminds us that the problem set that can be tackled by quantum is extremely small without a lot of hope of vast growth in the next several years, even with the addition of more reliable qubits with stable coherence times. Part of this is because so much is lost in translation between classical and quantum computing worlds, and some of it is the way problems are solved practically.
“Quantum computers are best applied to problems that are simple to state and have an answer that’s simple enough to check and look at and understand. Of course, in between that simple beginning and end is an insanely complicated calculation that just cannot be done in the classical universe. They don’t handle very large datasets or complex outputs and they are just not good at doing ordinary algebra—you have to actually do all the algebra and not just statistically analyze a linear algebra problem.”
There are other practical barriers for quantum in enterprise. For instance, there is no such thing as a quantum hard drive so anytime a calculation happens using a database for analytics or a neural network there is a conversion that is currently not possible. This is especially true for deep learning training since it would require ridiculous numbers of iterations just to do simple things like grab weights after every execution.
Given its enterprise focus and these major roadblocks for quantum, it is no surprise that HPE is targeting more near-term accelerators that have a better chance at adoption. Again, this harkens back to their DPE device, which is memristor based and in theory, could offer significantly lower power consumption and big compute capability, especially for matrix vector multiplication-heavy workloads like AI as well as for good old fashioned linear algebra. But even with memristors, there is still a long road ahead to make a technology an actual product—and another steep path to get people to actually use it since it is not without some new thinking, programming, support, and so on.
Memristors are available at TSMC and other fabs; these are not far-flung devices. However, the technology has quite a bit of maturing to do before it can be mass produced as a computational accelerator.
Beausoleil says it took him some time to come around to the idea of memory-driven computing, but he sees now how accelerators hung off a big memory machine (he naturally points to the Superdome Flex system from HPE) can deliver the post-Moore’s capabilities that will be more feasible to adopt than quantum. “One of the reasons I’m not doing quantum computing is that this new platform is exciting; it will allow companies to try wild ideas for accelerators on something that has an actual roadmap and is tested and demonstrated to outperform traditional processor-based compute systems.”
Right now the research team is focusing its post-quantum efforts on memristor scaling in particular. “Even when using external fabs enhanced by internal ones like we have here to do a proof of concept, making that a product–having that technical transfer at high levels of integration—is very difficult. We are trying to take something that works well in the lab and scale it out and in doing so, we have to make tradeoffs for reliability, practicality, and cost if we want to make these arrays by the billions.”
Even with all the technical and productization challenges, the steepest climb is delivering this to potential users, especially when it requires some real footwork. The way the system would operate is different though and the attachment to legacy systems is the biggest thing standing between Ray’s ideal future of accelerator experimentation and enterprise reality. “A full memory-driven system will require great steps to support and program and for us, our product folks are working hard to make it look as much like a legacy system as they can without getting in the way of enhanced functionality.”
And just because quantum might just one accelerator among many to snap into memory-driven systems, if and when they ever do find adoption, Beausoleil says that all the quantum work has not been lost. “Every physicist uses quantum mechanics to an extent, even if the emphasis is still on classical compute. The accelerators we are researching now to assist classical computers require an understanding of quantum mechanics to work properly,” he says, also noting that one area where quantum systems might be most useful is discovering replacements for silicon, which is nearing the end of the road.
Bravo. One who thinks along my line. When people do not understand magnetism properly, neither Gravitation quantum mechanics is at the beginning. Quantum computing depends on quantum mechanics which is not understood properly either. Physics today is flawed since it relays on the logic of Aristotle or these syllogisms that are the base of Boole algebra that is also flawed. Dieter L. Frischknecht. DLF3Q logics contains a system inherent „Kàlmàn“ filter as to accelerate computing or increasing precision with many steps in an algorithm. The DLF in dlf3q-logics are just my initials. The material is not published. I did not say that before. End of story.
Aren’t comments scrutinized by staff to sort out the likes of above DLF3Q nonsense,
or is my classical thinking too limited to understand the quantum of truth here ?
For readers new to your site and this article, it would have been helpful to write out what “HPE” stands for upon first reference.
Hewlett Packard Enterprise. We assume these to be household names in this industry and among our readers very familiar with it but fair point and well taken.
Bravo for Beausoleil! Quantum computing is an exercise in supercharging the computer’s ALU, (Arithmetic Logic Unit) via substitution of essentially multi-state spin valves for each of it’s presently dual-state bit positions. Faster is better, but even an instantaneous ALU is trivial in the total compute time stack. The real break-throughs need to be made elsewhere via AI scheduling of data and compute resources.
Imagine a sub-picosecond railroad round-table used to turn locomotives around: Impressive, but trivial in the total task of running the railroad. If we had Quantum today, cheap and with zero error rate, we’d discover John Non Neumann’s bottleneck still very much in place.
HPE resources have been transferred to contracts expected on the new Cray and HPE merger in building the New fastest computer for USA New Star Wars Monitor System.
https://www.theverge.com/2019/5/17/18629716/hpe-cray-acquisition-supercomputers-exascale-performance-deal
Intel & AMD are already doing Quantum Computer research.
You need the quantum: processor, FPGA interface and platform build before building a it.
A Quantum Computer must be run by the most powerful ruler first and a better one made before released to even a universities use.