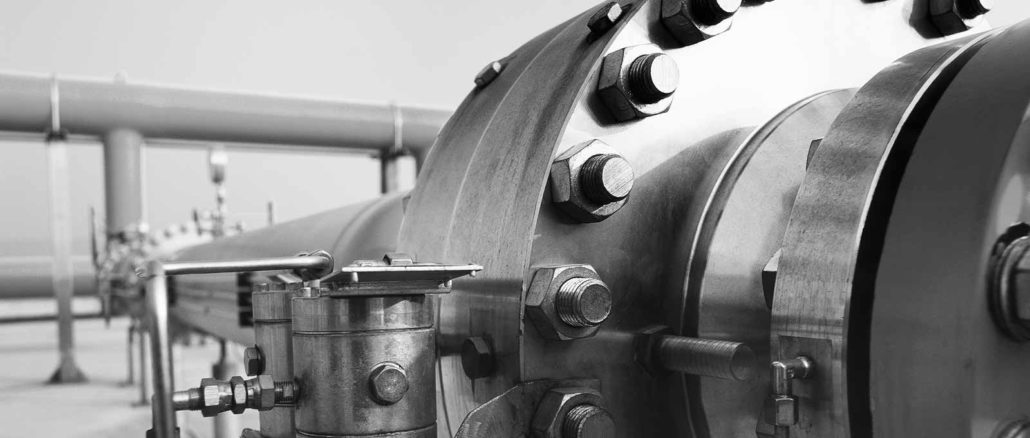
All the compute power in the world is useless against code that cannot scale. And neither compute or code can be useful if growing data for simulations cannot be collected and managed.
But ultimately, none of this is useful at all if the industry that needs these HPC resources is having more trouble than ever acquiring them. It comes as no surprise that the national labs and major research centers will be the first to get exaflop-capable systems, but in a normal market (like the one oil and gas knew not long ago) these machines would be relatively quickly followed by commercial HPC systems.
This will likely not be the case unless there is a rapid financial turnaround for energy companies.
Few industries have a tale to tell about the good old days quite like the oil and gas firms that have weathered the financial storm of the last few years. Despite the tumultuous economics of resource discovery, extraction, and production, competitive pressures are still pushing technology investments, including those that go toward a most precious asset—high performance computing.
Large supercomputers in oil and gas come with multi-million figure pricetags, and with the downturn new procurements are made with a much closer eye on ROI. Making decisions about new machines is somewhat easier now since there are likely to be larger gaps between processor generations—and less of a performance boost with them as well.
As oil and gas industry researcher and expert, Jan Odegard noted during the International Supercomputing Conference (ISC) this week, there is a greater need than ever for more robust supercomputing might, but for the systems that have just come onto the horizon, the cost is still far too high and it might be time to stop waiting in between processor generations and start looking to more heterogenous systems for a more tailored approach to seismic and reservoir analysis simulations.
Odegard is Associate VP of Research Computing and Infrastructure at the Ken Kennedy Institute at Rice University and the founder of the annual industry-focused Rice Oil and Gas Workshop. As one might imagine, the school’s home in prime energy territory, Houston, Texas, is advantageous. “Ten years ago, we started engaging with industry here in Houston to leverage our expertise. If you draw a circle around Rice with a 30-mile radius, there were 3600 energy companies of some sort,” he says—at least, until the industry took a nose dive. In that time, he has seen a shift in both the system and software requirements to move oil and gas forward, and both aspects are going to take some serious work to move the industry to the next level in terms of high-resolution simulations and data-intensive computing requirements.
“This has always been a data intensive community. We have used more cycles as an industry to compute FFTs than anyone before the Googles and Facebooks came along,” Odegard says. We were always at the edge of what computing had to offer,” but the edge of computing now comes with a wait time. “We used to see new processors every 18 to 24 months, it almost wasn’t enough time to get the codes up to speed. Now with times stretching into the 36 or even 48 month range, we are seeing an opportunity for increased heterogeneity and different architectures to fill the gap.”
As his chart that shows industry algorithms mapped along a line leading to exascale suggests, the clean upward curve is no more and the systems at the top end are far too costly and still quite out of range, even for the bleeding-edge supercomputers.
What is clear from the above chart is that there are applications that can take advantage of far more cores, assuming of course, the software can keep pace. The most noteworthy stories in HPC for the industry this year were trillion-cell simulations at Saudi Aramco on the largest machine at KAUST, a 716,800 processor scaling job from ExxonMobil on the Blue Waters supercomputer, and a very impressive effort from oil and gas software company, Stone Ridge that was notable for its scaling, efficiency, and time to result. These were all important code scalability stories—but there is still work on the code side to have seismic and reservoir analysis jobs efficiently use the entirety of an exascale machine.
Aside from the larger issues of code scalability (which are not unique to oil and gas) the sheer volume of data also represents a challenge, Odegard says. “If you look at seismic vessels that collect seismic data, you’ll see that in 1995, one of these towed six streamers; in 2005 there were sixteen and they were longer. In 2014 there were 24 that were 8 km long on one PGS ship. In this most recent vessel there are about 1200 or 1300 sensors per streamer. When you add that data just from one survey they ran that towed within a field of 280 sail lines for 2.6 million km of acquired seismic data (round trip to the moon 3.5 times) that gives you a sense of scope.”
In the end the example seismic collection effort took 660 terabytes of data back to the HPC group, which already has existing I/O and compute challenges. Those teams then want to visualize this data in ultra-high-resolution 3D images, which takes a combination of more compute power and fine-tuned algorithms. “Data volumes are a big challenge. The technology beyond 5 or 3 nm is hard to see, and there is this pipeline of data that is needed for sound decision making about where to drill.”
If the current host of chip and systems vendors cannot deliver an architecture that suits, Odegard says the community will look to more piecemeal architectures—GPUs, FPGAs, DSPs, and any other compute element that meets the needs of a particular workflow.
Even though the oil and gas industry make use of an order of magnitude more compute, there is still a need to keep scaling codes. The data deluge has to result in useful information fed into simulations and output as high-res 3D images. But Odegard says there is another problem for the future of HPC in oil and gas—a talent shortage. We have long heard national labs and academic centers talk about the Silicon Valley effect in its talent acquisition but Odegard says the “Fortran generation” is retiring, leaving a major gap. “People do not think of oil and gas as an IT field” and this has left a shortfall in replacing the geophysicists and others that have become experts in industry codes and systems. AI is coming to the field, however, which is could mark a shift in how these codes are written—and might open the way for younger developers or those from other computer science and engineering fields.
The point for Odegard and others that presented on energy industry applications in HPC at ISC is that having access to large-scale supercomputers is critical for competitiveness and risk mitigation. The more data exploration companies can collect, the more informed their models are. And the accuracy of these models (or lack thereof) can be the difference between spending millions on a drilling project that is successful versus dry. With an ROI like that, it might seem that this is one area where oil and gas wouldn’t question spending its money—but in times like these, ROI matters more than ever.
Be the first to comment