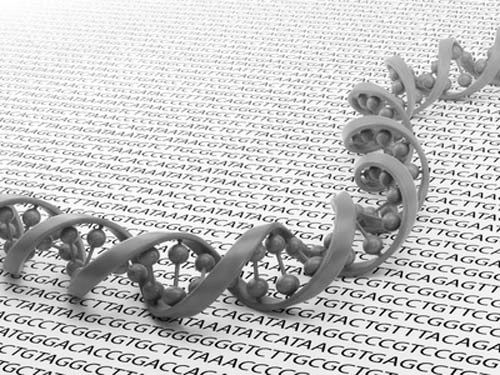
With the announcement of FPGA instances hitting the Amazon cloud and similar such news expected from FPGA experts Microsoft via Azure, among others, the lens was centered back on reconfigurable hardware and the path ahead. This has certainly been a year-plus of refocusing for the two main makers of such hardware, Altera and Xilinx, with the former being acquired by Intel and the latter picking up a range of new users, including AWS.
In addition to exploring what having a high-end Xilinx FPGA available in the cloud means for adoption, we talked to a couple of companies that have carved a niche from FPGA expertise to suit specific domains. Ryft, makers of an appliance for FPGA-boosted large-scale data analytics described how its hardware and software business will shift
When we spoke with Edico Genome a year ago, genetic sequence analysis had been pared down to twenty minutes using their custom-tailored FPGA-accelerated “Dragen” systems. That was an impressive feat then, but the company’s Gavin Stone now says they’re pushing near-real time for analysis. “We are able to do this at the speed of the data,” he tells The Next Platform, saying that the key to this speed is a mix between their own algorithmic tweaks and partial reconfiguration with both Xilinx and Intel/Altera FPGAs.
“Having eight of these FPGAs in a server is a big deal for us. As fast as you can move the data around we can analyze the genome, which is something that took weeks a few years ago, then days not long ago, and now is within minutes to nearly instantly.” This speedup now means processing elements, whether the FPGA or CPU (in the case of the F1 instances, there is a beefy Broadwell attached) is freed up for more complex analysis. “The current algorithms have an adequate level of accuracy, but because of computational and practical limits, there were many algorithms we’ve had in development that could deliver far higher accuracy numbers that previously realized. With FPGAs like this available, we are going to find and develop ways to make current gene analysis even better,” Stone adds.
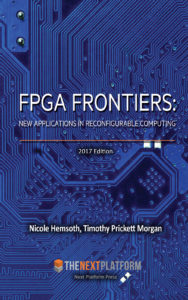
The company’s current FPGAs have around a million logic elements, but the newest Xilinx UltraScale parts in the F1 instance have 2.4 million. This is a big boost for genomics workloads, Stone says, and one that will allow teams to maximize the real estate available on the FPGA for far more complex workflows. Of course, to do this means taking a leap in the partial reconfiguration zone—swapping in and out different elements onto the FPGA for specific parts of the workload. This is not a simple task technically but it is the key to getting the full performance and efficiency out of a reconfigurable device.
Partial reconfiguration capabilities have always been available on FPGAs but it has been very difficult to make use of, in part because of the timing challenges with pulling parts in and out with a “live” FPGA that’s running other operations. “People have been using this approach but only in small elements; maybe carving out 5% or 10% blocks to swap. We are doing this at a 90% swap level, keeping things like the PCIe controller, drivers, and other essential functions alive and then swapping in other engine blocks on the fly.” With the genomics pipeline, for example, there is one block to handle compression, another for mapping and aligning, another for variant calling and so on. Ultimately, that single device can be used fully as an accelerator for many different functions, leading to a far faster time to result—something that is a key point of differentiation for users at genomics centers that want to provide analysis results at the point of care.
“With partial reconfiguration, there is no part of the device that lays dormant. It’s technically challenging, but once it works, it works extremely well. The barriers to getting to this point have been huge and we’ve worked with Xilinx closely on this, but this is becoming more mainstream as people start to realize how to truly use the FPGA to its fullest,” Stone says. This work has paid off for Xilinx and end users in other areas, who will find it easier to make use of partial reconfiguration—something that could promise an even larger field for FPGAs to play in.
Although partial reconfiguration is a challenge for many shops, this is the sweet spot for Edico Genome and the reason they can deliver such fast results. That capability will be coming to the cloud soon, albeit hindered slightly by the data movement delay. This is not something that will add a huge barrier, and Stone says this will keep the company’s own hardware business alive since there will always be centers that need the ultra-low latency time to results on site.
When Edico’s business started, the idea had been around custom ASICs, but the volume and flexibility story wasn’t there. Stone says he expects this ASIC versus FPGA question will be less pressing as those in genomics and other areas realize that even though using partial reconfiguration is still not simple, it beats the economics of driving a chip to production and taking such a big financial risk. “We are truly able to use the FPGA to its fullest,” says Stone, pointing to the benefits of their partial reconfiguration approach. “We do reconfigure on the fly, in the middle of the workflow, which not many people do. Normally, you have to do a full reconfiguration, but we’re keeping a lot of the FPGA live and swapping portions in and out. There is no way we could do that with an ASIC. And as many see now, genomics is evolving quickly; the algorithms change and update and pushing those through quickly with an ASIC would not be feasible.” Stone says that the costs of FPGAs will come down as will some of the programmatic complexity, making ASICs make less sense.
“There is widespread adoption of FPGAs now; it’s really caught fire over the last few years as we’ve seen the writing on the wall with Microsoft’s Catapult project and others getting a lot of attention. There used to be niche providers in the cloud but with Amazon putting FPGAs out there, it is going to more mainstream—not just for genomics but other data-rich applications.”
Be the first to comment