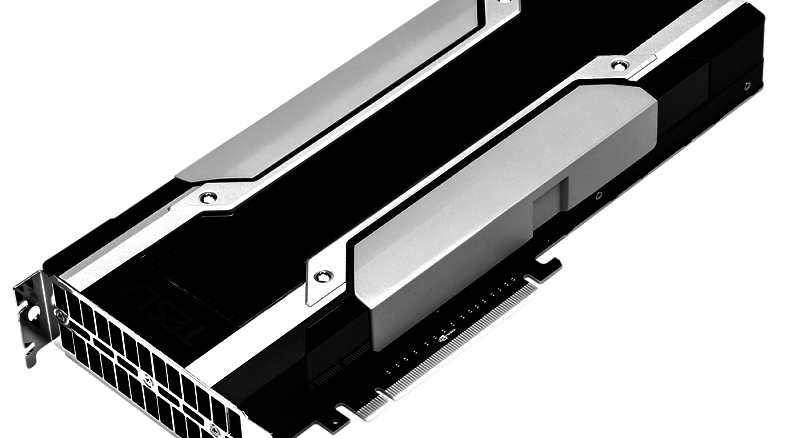
As we have noted over the last year in particular, GPUs are set for another tsunami of use cases for server workloads in high performance computing and most recently, machine learning.
As GPU maker Nvidia’s CEO stressed at this year’s GPU Technology Conference, deep learning is a target market, fed in part by a new range of their GPUs for training and executing deep neural networks, including the Tesla M40, M4, the existing supercomputing-focused K80, and now, the P100 (Nvidia’s latest Pascal processor, which is at the heart of a new appliance specifically designed for deep learning workloads).
While we have heard a great deal over the last year from companies like Baidu, Flickr, and others, on-premises GPU-laden systems are the key to training deep neural nets, but according to IBM, there will be a new wave of users who want to circumvent the on-site boxes and take advantage of GPUs on IBM’s cloud.
While cloud rival Amazon Web Services, among others, are sporting GPU cards for high performance computing (HPC) and deep learning users, the partnership between Nvidia and IBM is giving Big Blue a leg up in terms of making a wider array of GPUs available to suit different workloads. Currently, IBM’s cloud boasts the K80, as well as the lower power and less beefy K10. Today that suite of GPU options was enriched with the addition of the virtualization-ready Nvidia M60 cards, which can support a wider range of workloads—from HPC applications, to machine learning workloads, to virtual services and gaming platforms.
As Jerry Gutierrez, HPC leader for SoftLayer tells The Next Platform, adoption of GPUs in their cloud is moving from experiment to production. “Just two years ago, there were several companies kicking the tires with GPUs, but now we’re seeing more of a production focus in everything from machine learning to VDI. Customers are getting more comfortable with the idea of cloud in general, and now cloud for HPC, machine learning, and other types of workloads.”
“We’re at an inflection point in our industry, where GPU technology is opening the door for the next wave of breakthroughs across multiple industries.”
With the existing K80 GPUs, which as a reminder, are the same as found on the world’s top supercomputers, Gutierrez says the most prolific users are in financial services. While he says machine learning use cases are still in the experimental phase on the K80 GPUs, adoption for accelerating risk analysis has jumped significantly over the last year in particular as more financial companies moved from the testing phase to actual production.
IBM is expecting that the M60 GPU will be useful for customers who want to move machine learning and deep learning training into the cloud, but he says for now, a lot of them have started by using the far cheaper Titan X GPUs ($1000 versus several thousand) as Baidu does. He says that while he knows many shops are using these consumer cards, the Titan X can’t be licensed for use in the cloud (Nvidia’s Marc Hamilton talks about that in more detail here), but the K80 is already serving production machine learning use cases and the M60 will do so as well.
At the very low end of the GPU spectrum on IBM’s cloud, there is a Kepler generation graphics card, the K10, which Gutierrez says is something of a niche product with only a few users. Where the greatest momentum is can be found with the K80—a trend he expects will be echoed when the Nvidia Grid technology is enabled on a GPU that can support multiple workloads.
One challenge IBM is working through with Nvidia now is on the licensing front. With the virtualized instances, finding a model where users can pay monthly or weekly (versus per instance or on shorter time periods) has been problematic. He notes that the two teams are working together to establish a pricing per use paradigm that works for both end users and the graphics card maker.
While not surprising given their alliance with Nvidia in the OpenPower Foundation, the IBM cloud has more GPU options than other large public cloud vendors. As our own Timothy Prickett Morgan noted earlier this year, at the moment, Nvidia identifies six cloud providers that provide cloud-based GPU capacity or hosted GPU capacity. (The former is available on demand at hourly rates, while the latter is for longer-term hosting engagements.) Amazon Web Services was the first to offer GPUs on demand among the big public clouds, back in November 2010, when it put Tesla M2050, using the “Fermi” GPUs from Nvidia, on its CG1 compute instances, which sported “Nehalem” Xeon X5570 processors from Intel and 10 Gb/sec networking to link nodes together. Those Tesla M2050s provided 515 gigaflops of double precision floating point oomph across their 448 CUDA cores, and each node had two of them; AWS allowed customers to glue up to eight nodes into a baby cluster with 8.24 teraflops aggregate and if they needed more than that, they had to call.
Three years later, AWS launched its G2 instance types, which use Nvidia’s GRID K520 GPUs, which are useful for both compute and visualization work. These server nodes, as it turned out, had four K520 cards and used Intel’s “Sandy Bridge” Xeon E5 processors. In April of this year, AWS expanded the G2 instance so the whole server and all four K520s could be deployed as a single instance. These K520s are really aimed at single precision workloads and does not support error correction on the GDDR5 memory on each card. So it is suitable for seismic analysis, genomics, signal processing, video encoding, and deep learning algorithms, but not the heavy duty HPC simulations that model physical, chemical, and cosmological processes and generally use double precision math. In any event, the latest G2 instances have up to four GPUs across two cards in the server, each with 1,536 CUDA cores and 4 GB of frame buffer memory to run applications; Nvidia does not provide floating point ratings on the GRID devices, but the cores run at 800 MHz, a little faster than on the Tesla K10 that it most resembles, and that means the four GPUs should weigh in at around 9.8 teraflops at single precision. The other vendors that Nvidia cites as having GPUs either on demand or hosted include Nimbix,Peer1 Hosting, Penguin Computing, Rapid Switch, and SoftLayer.
Be the first to comment