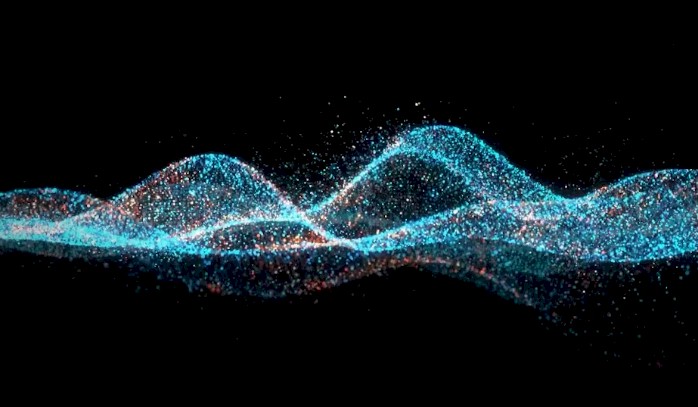
For a decade before the generative AI boom took off in late 2022, classical artificial intelligence, used for all kinds of self-learning predictive algorithms, was destined to be a very large component of the IT stack at most organizations in the world. With all of the excitement around GenAI, it is important to remember that these foundation large language models represent only a portion of the AI market, and ultimately the IT market, because in the long run, AI will be as normal as a relational database or a Web server, or consuming compute, storage, and networking in the cloud.
But when a thing is new, as AI still is even after a decade of production use, we still like to dice and slice the market up to show who is benefitting from the new wave, and how. There are a lot of opinions about how AI is changing the IT market, particularly when it comes to how the money is piling up – or not. So we like to show you interesting forecasts when we see them.
The prognosticators at IDC put out some numbers recently, forecasting that worldwide spending on AI infrastructure, services, and applications would grow at a compound annual growth rate of 29 percent between 2024 and 2028, reaching $632 billion at the end of the forecast period. If you work that backwards, then the AI market is projected to be about $228 billion in 2024.
IDC says further that the GenAI segment of the overall AI market will account for $202 billion in revenues across hardware, software, and services in 2028, with a compound annual growth rate of 59.2 percent and representing 32 percent of overall AI spending. If you work that CAGR backwards, then GenAI would only push $31.5 billion in revenues in 2024. So we are immediately wondering how IDC is carving up the massive GPU and networking revenues for the datacenter business at Nvidia, which should surpass $100 billion in revenues this year. We think the shape of the curves IDC is drawing are about right, but we question the small revenue numbers implied by the CAGR rates for 2024.
We concur that software will be the largest spending category for the AI market once you start counting AI-enabled applications, and we think that within a few years, there will not be back office software from third parties that is not augmented by AI in some fashion. IDC says that software will be more than half of the AI spending over the forecast period, and two thirds of that software spending will be for AI-enabled applications and AI platforms. (Which will essentially become enterprise software.) AI software will grow at a 33.9 percent CAGR between 2024 and 2028 inclusive, AI services will grow at 24.3 percent, and hardware will grow at a slower (and unspecified) rate.
The top AI applications that will drive the market are augmented processing claims, digital commerce (mostly recommendation engines and search engines we presume), augmented sales planning and prospecting, smart factory floors, and augmented product design.
Also interesting is the assertion that more than half of AI spending – $336 billion – will be done in the United States in 2028, and that this share of spending will be about the same from 2024 through 2027. GenAI spending is expected to drive $108 billion in revenues in the United States by 2028. Europe will be the next biggest market for AI and GenAI, and China will be third. No surprises there, since that is the relative ranking of the economies of those regions.
In a separate report, IDC said that AI platform software would hit $153 billion in revenues in 2028 and is growing at a CAGR of 40.6 percent from 2023 through 2024. (That 2023 is not a typo.) AI platform software was $27.9 billion in 2023, up 44.4 percent from the $19.3 billion level in 2022. Microsoft is the biggest AI platform provider, followed by Palantir, OpenAI, Google, and Amazon Web Services, says IDC.
We think it is important to distinguish revenues made through companies from investments made by companies when it comes to AI. We see a lot of the latter and not a lot of the former excepting Nvidia and a smattering of other companies like AMD.
But more importantly, we also think it is hard to draw lines between AI spending and not AI spending because we have insufficient data. For instance, how do you allocate the $13 billion that Microsoft has invested in OpenAI? And do you count the round-tripped money that comes back to Microsoft when OpenAI pays to rent capacity on the Azure cloud to train its models? Ditto for Anthropic and its relationships with Google and Amazon Web Services. When the entire enterprise software stack from Microsoft, Oracle, and thousands of on-premises and SaaS software vendors add AI functions to their applications, how do you allocate the AI portion of that?
Inquiring minds want to know. . . .
What we know for sure is that companies investing heavily in AI right now will bend over backwards to show that their efforts have return on investment and to prove to Wall Street or their company owners (if they should be so fortunate as to not be public) that their investments in AI are panning out.
We saw this during the ERP Boom of the late 1990s – and it was not hard to show the ROI of using SAP R/3 instead of having to gut them have four digit dates instead of two digit dates. Bending the company into pretzel shapes to fit SAP’s conception of each industry was far easier and less risky but probably as costly as dealing with the Y2K issue when it became apparent to everyone in 1998. At the same time, during the Dot Com Boom during the late 1990s, it was not hard to prove out the ROI of having a presence on the commercial Internet and using standardized technologies to link machines and applications to each other.
It will be tough to count what share of functions that might have otherwise have been hand-coded by programmers are now being done by AI algorithms or how many new capabilities have been created because only AI could do them at a practical scale and cost. How on Earth did we do language translation before large language models? Poorly, slowly, and at great cost. And now, we have a Universal Translator, which is quite frankly amazing. But perhaps not so much to the untold numbers of people who have spent their lives understanding the subtleties of French or Japanese or German or English to help better convey meaning across cultures.
No one could measure forces and energies before the Big Bang. You just explode a Universe into existence and you see what happens with a few basic rules. And you can only see what happens by living it, and you can never experience the totality of it from the inside. In concept, this AI explosion is no different.
The fact is, AI is happening, people are spending fortunes, and it will transform the world – but it is very difficult to say precisely at what speed and by how much. Which was the same for the written word, roads, science, canals, railroads, telephones, television, and the Internet. And because we are scientists, who like to cut things into their components and measure them even as they are moving, this is terribly frustrating. So rather than criticize IDC, Gartner, and others for trying to figure this AI revolution out, we commend them as we do the scientists at CERN who monkey around with the Large Hadron Collider trying to find a God particle.
Be the first to comment