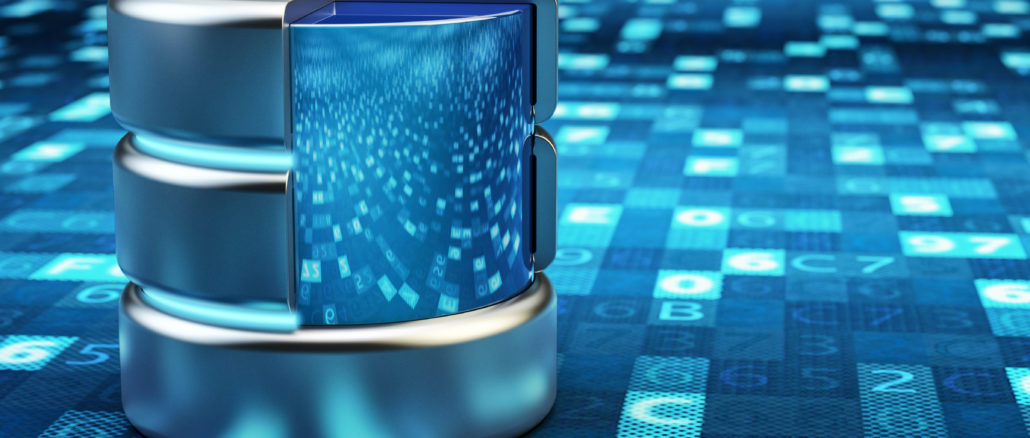
SPONSORED FEATURE: So, you’re finally ready to jump on the AI bandwagon. You have oodles of data lying around your company and you’re eager to unlock its value. But wait a minute – is your infrastructure ready to handle it? Look closely, and you’re likely to find bottlenecks that will choke your AI pipelines. Fixing those issues is a vital part of the AI journey.
With the current interest in generative AI, there has never been a better time to get your infrastructure ready for AI workloads. In August 2023, McKinsey reported that generative AI had prompted plans from 40 percent of organizations to increase their overall investment in AI.
Today, companies are using both generative and non-generative AI for a wide variety of enterprise use cases. The top one is customer service, according to Forbes Advisor’s survey of 600 business owners. In second place is cybersecurity or fraud management, as 51 percent of companies explore the use of machine learning to spot suspicious activity. The use of AI for enterprise digital assistants comes in third, indicating a strong interest in the generative AI that increasingly underpins those personal productivity agents. Then come CRM, inventory management, and content production.
Cloud computing has powered a lot of these AI use cases, but it’s often cheaper for larger companies to handle at least part of the AI workload on their own premises. However, they face two key challenges.
Shortcomings in enterprise infrastructure
The first is that their existing infrastructure is often inadequate to support the unique requirements found in AI workloads, warns Steve Eiland, global HPC/AI storage product manager at Lenovo.
“As many folks start to understand what they want to do with their AI solution and put it together, they don’t figure out where the bottlenecks are in their systems,” Eiland says. They run into performance issues as they struggle to build and execute the data pipelines that feed hungry machine learning applications.
Eiland breaks those data pipelines into four main components. The first is data ingestion, which handles upstream filtering and buffering. Second comes data preparation, in which data scientists clean, normalize, and aggregate data for the training process. This is also the part of the pipeline where human operators will apply metadata to that data, labelling it for supervised machine learning.
Then comes training, the compute-intensive process in which the statistic model for inference is created. As data scientists know all too well, this is an iterative process that often requires many training runs to fit the desirable outcomes as accurately as possible. Eiland also includes post-training data archiving as part of the data pipeline.
“Instead of putting a seamless infrastructure together, companies break each piece into segments and each piece ends up working as a silo,” Eiland says. “Those silos cause latency and timing issues, and everybody’s also doing their own thing within their own silo.”
Siloed infrastructure, constrained by performance bottlenecks, is one of the problems that Lenovo hopes to solve with its “AI for All” strategy. It draws on its broad data infrastructure portfolio to create unified configurations of CPU, storage, GPU, and network equipment certified to work together from end to end. The company focuses on verticals like retail, manufacturing, finance, and healthcare, consulting with customers to assemble AI solutions mapped to their specific requirements.
Software-defined storage for AI pipelines
Lenovo’s solution includes storage based on software-defined storage principles. This concept enables customers with data-hungry AI workloads to scale up storage capacity without sacrificing performance, says Alexander Kranz, Director of Strategy at Lenovo.
“When you look at a traditional storage array, you can add capacity easily but adding performance is often more difficult,” he says. “The ability to keep that linear growth with performance and capacity is very valuable in these kinds of workloads.”
To address the largest, most high-performance data sets, a software-defined storage solution is often required to deliver the capacity and performance scale to power the most demanding AI pipeline needs. Lenovo has added a partnership with WEKA and architected solutions that can provide a single namespace across storage infrastructure located anywhere for example, including in the cloud or compatible on-premises systems.
Lenovo’s High Performance File System, with WEKA Data Platform enables customers to build AI data pipelines sourcing data from multiple locations across a single software-defined storage infrastructure. It helps provide access to the relevant data where and when it’s needed with minimal management overhead, compressing complex data pipelines. That’s critical for customers trying to feed those pipelines, says Kranz.
“How do you keep these GPUs active and used?” he muses. “We often find customers buying them because they think they need them, but they don’t have the data pipelines ready to drive that infrastructure.”
Enterprise customers with smaller AI data sets can leverage the Lenovo ThinkSystem DG Series storage arrays with Quad-Level Cell (QLC) flash technology for best price-performance. The Lenovo DG series provides enterprise class unstructured data storage for read-intensive enterprise AI workloads, offering faster data intake and accelerating time to insight.
Supporting multiple deployment models
For AI workloads, a global namespace allows users to make zero-cost copies instead of copying data to different storage solutions from within data silos, Kranz says.
Kranz recognizes that there’s a strong impetus for many to deploy AI in various configurations rather than purely on their own premises. This includes both hybrid cloud and edge-based configurations where data is collected on edge devices and either processed locally or sent to a central point.
The Lenovo High Performance File System solution provides an easy option for customers to transfer AI data to and from the cloud for processing, he says. Lenovo’s ThinkEdge solutions can also sit at the edge and run AI workloads locally.
“Many of our customers have edge data relevant to AI, such as sensor and video data. The ability to efficiently move that data back to the core to be used to continue to improve AI models over time is important,” Kranz adds.
Condensing network, compute, and storage with HCI
Lenovo also excels at hyperconverged infrastructure (HCI), which simplifies the deployment of virtual workloads used for AI/ML tasks like model training by reducing management overhead.
“Our systems allow for that data to be easily moved back and we can even use data reduction where appropriate to reduce the amount of data being sent from the edge to the core,” says Kranz. “This also applies in reverse: sending the new models for the inference engines at the edge to run.”
Inferencing is often a critical part of the pipeline, vital to making sure that AI projects deliver business value. This can be especially for those which harvest and process information at edge locations. While these datasets may not be especially large, they can be mission critical, and organizations still need them to be easily accommodated, often using variable combinations of compute and GPU resources. Security inferencing at the edge, for example, can be not only mission critical but also safety critical depending on the specific application, which means AI may be the single most important workload in that location.
HCI’s software-defined nature makes it easier to scale data and computing resources for AI. The ThinkAgile line of HCI servers merge network, storage, and compute together using integrated data processing units (DPUs), otherwise known as SmartNICs.
These merge high-speed network interfaces, software-defined storage management, and NVIDIA accelerators onto a single ASIC. Lenovo estimates that offloading the high-speed networking function onto a separate DPU can free up 20 percent of the CPU’s time, while removing the bottleneck for high-speed data transfer to the AI accelerator.
Storage as a service
As more enterprises adopt AI, different approaches to data management will also be required depending on the individual requirements of both the workload and the organization involved. The requirements for training and implementation of off-the-shelf AI models will be different than large scale generative AI (GenAI) models or LLMs. And there will also be different performance and RAS requirements depending on the specific model and data included.
The other thing that Lenovo can do to help customers address those diverse requirements is to flex the data storage that they need across their on-premises systems. AI workloads frequently need high-capacity storage for short amounts of time as they prepare vast amounts of data for training runs. That presents customers with a difficult choice: over-provision storage and face high capital expenditures, or under-provision and watch AI workloads choke during periods of high demand. Neither of those is appealing, which is why storage as a service is becoming increasingly important for customers.
Lenovo’s TruScale Data Management solutions offer installed equipment that customers pay based on usage. Customers can increase and reduce their usage of the systems at will, only paying for their current capacity, making this storage pricing model similar to the public cloud.
There is another service level within this service-based storage model: TruScale Infinite Storage. This includes a full-stack refresh on all storage-related hardware after a set period, including controllers. This helps keep customers up to date as they strive to sustain and enhance the performance of their AI pipelines, says Kranz.
Kranz also highlights some other notable advantages in managing AI workloads using this optimized end-to-end approach. One of them is security for sensitive data used in machine learning environments.
“AI relies on a huge volume of unstructured data. That’s why beyond normal encryption for data at rest and in flight, we also offer the ability to create immutable snapshots and copies, automated ransomware protection to detect and alert against suspicious behavior, and multi-factor authentication to reduce the risk of unauthorized access,” he says.
Lenovo automates as much of the infrastructure management as possible to maximize performance. For example, it offers quality of service features that allow users to prevent bottlenecks by setting minimum and maximum IOPs settings.
Despite the obvious potential, it’s still very much early days when it comes to enterprise adoption of AI technology. As more organizations come to embrace the technology, it’s likely that greater volumes of mission critical workloads with enhanced requirements around security will come into the picture.
Ultimately, AI looks set to change the way that companies work, from the inside out. The efficacy of these projects depends on many things, including building a solid strategy, creating an ROI model, and putting proper safeguards in place. But none of it will get off the ground unless the data flows freely.
Sponsored by Lenovo.
Be the first to comment