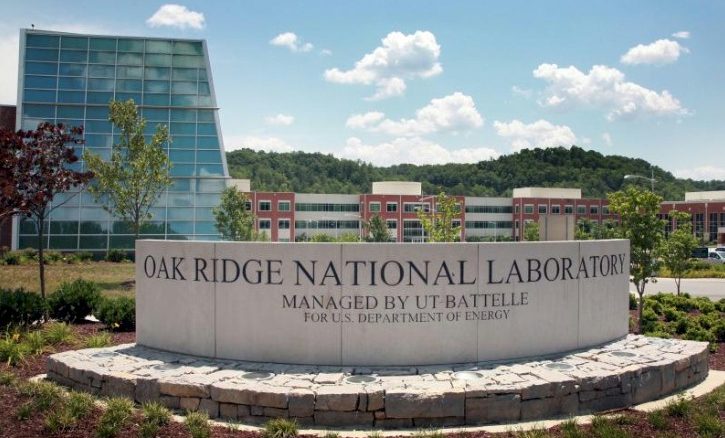
The confluence of big data, AI, and edge computing is reshaping the contours of high performance computing. One person who has thought a lot about these intersecting trends and where they are taking the HPC community is David Womble, who heads up the AI Program at Oak Ridge National Laboratory. At The Next Platform’s HPC Day ahead of SC19, we asked Womble to offer his take on how this might play out.
From his perch at ORNL, he sees a couple of trends that are taking place in parallel: the merging of traditional simulation and modeling with AI and the blending of edge computing with datacenter-based HPC. Together, he believes, these mashups will profoundly change the way high performance computing is done.
Of course, some of these changes are already well underway. AI-optimized hardware, like Nvidia’s V100 GPU has become standard componentry in many HPC systems over the last couple of years, including ORNL’s own “Summit” supercomputer. This has encouraged practitioners to explore the use of artificial neural networks for a wide array of science and engineering applications.
Womble sees artificial neural networks being used in a number of different ways, one of the most important being to serve as surrogates for physics-based simulations. By training the neural networks to simulate the behavior of the physics, they can shrink the number and scope of the more computationally-intense simulations, reducing system load. As a consequence, greater scalability on these systems can be achieved than would otherwise be possible if everything was performed using brute-force physics calculations.
“The concept of using a machine learning or AI-based surrogate for a physics-based model allows us to have a computationally efficient approach to crossing scales,” explained Womble. He thinks the AI models are migrating from strictly science domains to those of engineering, especially in areas like materials design and combustion modeling, where these techniques are currently being employed. According to Womble, tremendous progress has already been made.
That’s not to suggest that simulations are going to be replaced entirely. Womble noted that a large body of knowledge and techniques based on these physics-based models has been constructed and they have proven to be quite accurate. The opportunity now is to supplement them with AI technology.
Related to the emergence of these AI techniques is edge computing, which is conveniently close to the source of much data: sensors, factory monitors, and all kinds of other streaming data source. As such, edge computing is ideally suited to use its proximity to data to train and inference artificial neural networks (as well as perform some of the more mundane tasks of data reductions and filtering). However, because there is only so much computational hardware that can be squeezed into edge device, not all AI belongs there.
Womble envisions a hierarchy of edge environments, with the outermost layer ingesting the streamed data and performing some initial processing. At this outer layer, the dictates of data latency and limited hardware necessitates very fast inference and perhaps only limited training.
Closer to the datacenter may be an inner-edge environment that does more extensive training and data analysis. That environment may be comprised of something closer to a conventional server, with more powerful hardware and less stringent requirements for real-time processing. Womble characterized this environment as “the beginning of HPC” – less powerful than a full-on supercomputer and perhaps and not so tightly wound communication-wise. The results of this layer will feed into a more traditional HPC datacenter, where even more extensive analysis and model building can take place.
As a result, he believes traditional HPC is going to look a lot more distributed in the future. “I think we will be placing machines that are the equivalent scale of Summit very soon at some of the larger facilities,” Womble said. “So you might place a quarter of a Summit-scale machine at our Spallation Neutron Source facility.”
Again, some of these changes are already in evidence. At ORNL, the scope of their partnerships has broadened because of this edge-to-datacenter connection. Womble said they are increasingly talking with organizations interested bring the edge into their computational infrastructure, for example, groups who do city planning, automobile companies who are building smart vehicles, and government agencies interested in disaster prediction and response.
With the ORNL’s legacy of expertise with physic-based simulations, data analytics, and now AI, the lab is well-positioned to help to reshape the nature of high performance computing into one that is much more data-centric. “It’s a very natural evolution to think about how to bring edge in,” said Womble.
Be the first to comment