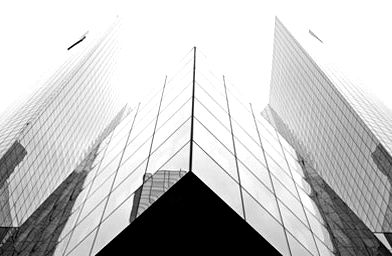
Back in 2008, the global economy came crashing down sending many organizations and individuals into a state of financial ruin. Three major banks in Iceland collapsed, forcing the country into a deep recession. Fingers were pointed at the banking institutions — bank officers and mortgage lenders were blamed for abusing their fiduciary duties toward their customers by putting indulgence and manipulative greed above the stability of the society.
Since then regulations have been strengthened and the financial industry has adopted sophisticated mathematical models and high performance computing systems to study and assess all types of risks in the attempt to minimize them. However, is this practice sufficient enough to assure that our money is safe with the banks and their investment strategies are sound?
Erik Vynckier, CIO of AllianceBernstein, will be speaking about “High Performance Computing in the Financial Industry: Problems, Methods & Solutions,” at the upcoming ISC conference in Frankfurt, Germany in July, which focuses on supercomputing technology in research and enterprise settings.
Prior to entering investment banking and later joining the insurance sector, you had also spent a considerable amount of time in the petrochemical industry. What were some of the connections you make there now with respect to complex modeling and the systems required to do it well?
Vynckier: Mathematical modeling and technology are great unifiers of knowledge across sectors. You can change application domains, and by catching up on the knowledge base, quickly re-establish yourself in a new area.
The mathematics and the quantitative modeling expertise, as well as the development & implementation of numerical programs aren’t necessarily all that different in the financial sector from the industrial sector.
There is one danger to watch out for however: simple one-for-one porting of models from one context to another, without paying mind to the actual mechanisms at work in the application is very naive and risky. The devil hides in the details!
In fact, scientific models poorly ported to the financial arena led to some grave mistakes and even catastrophic failures. IIl-adapted models that didn’t fit the financial markets were crudely implemented, often without questioning or investigating the key assumptions that made them successful in science. In this way, poor modeling contributed to and aggravated the credit crisis.
So how did this all translate into the financial modeling realm?
Vynckier: At previous companies I have implemented a high performance computing platform for real-time, dynamic cross-asset hedging of guaranteed life assurance policies. I also developed a scenario tool for the projection and stress testing of derivative overlays commonly used in liability driven investment strategies. Accurate valuation, accurate hedging, optimal collateral planning and confident product development resulted – all on the same platform. Sharing an integrated platform across different functions and departments limits development costs and increases the speed of developing and bringing to market new financial products.
Can you describe the kinds of work your company is doing with high performance computing?
Vynckier: At AllianceBernstein, the agenda for high performance computing and big data in finance centers is about making best use of time-series data from the capital markets, such as traded prices and volumes and order book data, as well as other economic data for optimally investing client money. Smart beta – distilling sound quantitative strategies from economic and market data – requires searching, backtesting and fundamentally understanding investment trends, such as the well-documented systematic biases of carry, value, momentum and low volatility anomalies.
On top of identifying the investment strategies that promise success, estimating and controlling the implementation costs of these trade-intensive strategies is crucial. Risk management of quantitative strategies requires not just back-testing, as the future may not be apparent from the past, but also implementing effective diversification across strategies. Managing volatility down to an acceptable level is also important and is accomplished through volatility-targeting and tail-risk hedging. Each step of the investment process can benefit from big data techniques.
What kinds of risks are there to be managed and are they being managed adequately now?
Vynckier: There are many risks in the economy and, in particular, in the financial sector. Let’s start by listing the individual risk categories.
Financial institutions, but also corporates and households, carry a lot of financial risks: credit risk or companies or sovereigns defaulting; foreign exchange risk on a foreign investment or in the course of international trade; interest rate risk on a bond, a loan or a mortgage, or on the mismatch between assets and liabilities of the corporate or financial balance sheet; and equity risk (in a company stock or a portfolio of stocks. …
Insurance risks are also commonly incurred: these might be risks on events, such as catastrophic weather or geologic events, violent wars and terrorism, or risks on trends like mortality and longevity risks on a pool of lives, or operational risks such as the business impact of mistakes and criminal activity, including cyber terrorism.
Whereas credit risk was the trigger of the credit crunch, in fact liquidity risk has caused the most hardship for financial institutions and their clients: as well as consumers and companies. Liquidity risk materializes when payments are missed because companies cannot meet deadlines. On top of business failures originating with liquidity constraints, illiquidity can be forced on a financial institution when posting collateral to a counterparty or cash variation margin to a clearing house in support of a derivative overlay.
Each of these risks is now being studied with large-scale models that need to run on high performance platforms, since the size of the trading books and the granularity of the phenomena being modeled – multiple markets, multiple instruments, multiple time-steps, multiple counterparties and multiple types of collateral and credit support annexes. The investigation of empirical data also quickly scales so as to require big data techniques for real-life risk management.
All of these risks are monitored and managed at the enterprise level. By combining big data techniques with enhanced, more granular regulatory reporting, economies can now, in fact, go a step further. The next breakthrough in risk management is tackling systemic risk! Individual firms cannot see through the cob-web of counterparty risks which connects and magnifies individual risks into economy-wide systemic risk. But with the advent of Pillar 3 disclosure requirements, the regulators stand a chance of seeing through the cob-web. Big data techniques will inevitably be required to search through the masses of financial data hiding the connections between the different financial institutions.
So what does it take to achieve effective risk management?
Vynckier: Best practices in risk management simply lift the industry as a whole to a higher level. Furthermore, industry-wide application of best practices pre-empt dysfunctional regulation from taking a foothold. For quality, speed of deployment and cost effectiveness, buying commercial software is nowadays often the better option. The niche is being filled by independent analytics vendors and risk consultancies which develop software for multi-clients, often even for direct competitors.
There should be a dose of realism amongst CEOs that their financial institutions do not necessarily have unique quantitative risk or software development skills compared to the best of the sector. The difference between successful and failing financial institutions lies more in the culture of the organization and the engagement of the people running the business than in the quantitative risk management software or hardware per se. The best organizations will recognize their limitations and set up effective cooperation with the specialist vendor ecology to achieve best-in-class tools.
Does the European finance sector rely 100 percent on the Monte Carlo simulations for risk management? Are these simulations powerful enough to meet the new regulatory requirements, not to mention avoid market meltdowns? Are there alternative techniques being explored?
Vynckier: The financial industry relies on a large number of quantitative techniques to invest money, trade securities, manage risks, allocate capital and map out its future business. Monte Carlo stands out for the versatility and ease of formulation of new applications, but sometimes other numerical techniques such as Partial Differential Equations and Fast Fourier Transforms are also used and they can prove more efficient than Monte Carlo for the right applications. Automatic Differentiation is now spreading into the financial sector as well.
Optimization techniques such as Levenberg-Marquardt for fitting the model parameters to replicate the market prices of commonly quoted instruments are routinely applied. Complex optimization of investment strategies over large sets of real-world scenarios involves stochastic dynamic programming. Stochastic programming has expanded into institutional asset-liability management, as well as in wealth and retirement planning. These large-scale optimization problems require high-performance techniques or cloud platforms.
Vynckier will be providing more details about this and other financial services companies’ ambitions on high performance computing systems this July at ISC in Germany. The Next Platform will be on hand, hope to see everyone in Frankfurt…
Be the first to comment